Understanding the Mechanism of Deep Learning Frameworks in Lesion Detection for Pathological Images with Breast Cancer
SENSORS AND MATERIALS(2022)
摘要
With the advances of scanning sensors and deep learning algorithms, computational pathology has drawn much attention in recent years and started to play an important role in the clinical workflow. Computer-aided detection (CADe) systems have been developed to assist pathologists in slide assessment, increasing diagnosis efficiency and reducing misdetections. In this study, we conducted four experiments to demonstrate that the features learned by deep learning models are interpretable from a pathological perspective. In addition, classifiers such as the support vector machine (SVM) and random forests (RF) were used in experiments to replace the fully connected layers and decompose the end-to-end framework, verifying the validity of feature extraction in the convolutional layers. The experimental results reveal that the features learned from the convolutional layers work as morphological descriptors for specific cells or tissues, in agreement with the diagnostic rules in practice. Most of the properties learned by the deep learning models summarized detection rules that agree with those of experienced pathologists. The interpretability of deep features from a clinical viewpoint not only enhances the reliability of AI systems, enabling them to gain acceptance from medical experts, but also facilitates the development of deep learning frameworks for different tasks in pathological analytics.
更多查看译文
关键词
CADe system, lesion detection, deep features, visual interpretability
AI 理解论文
溯源树
样例
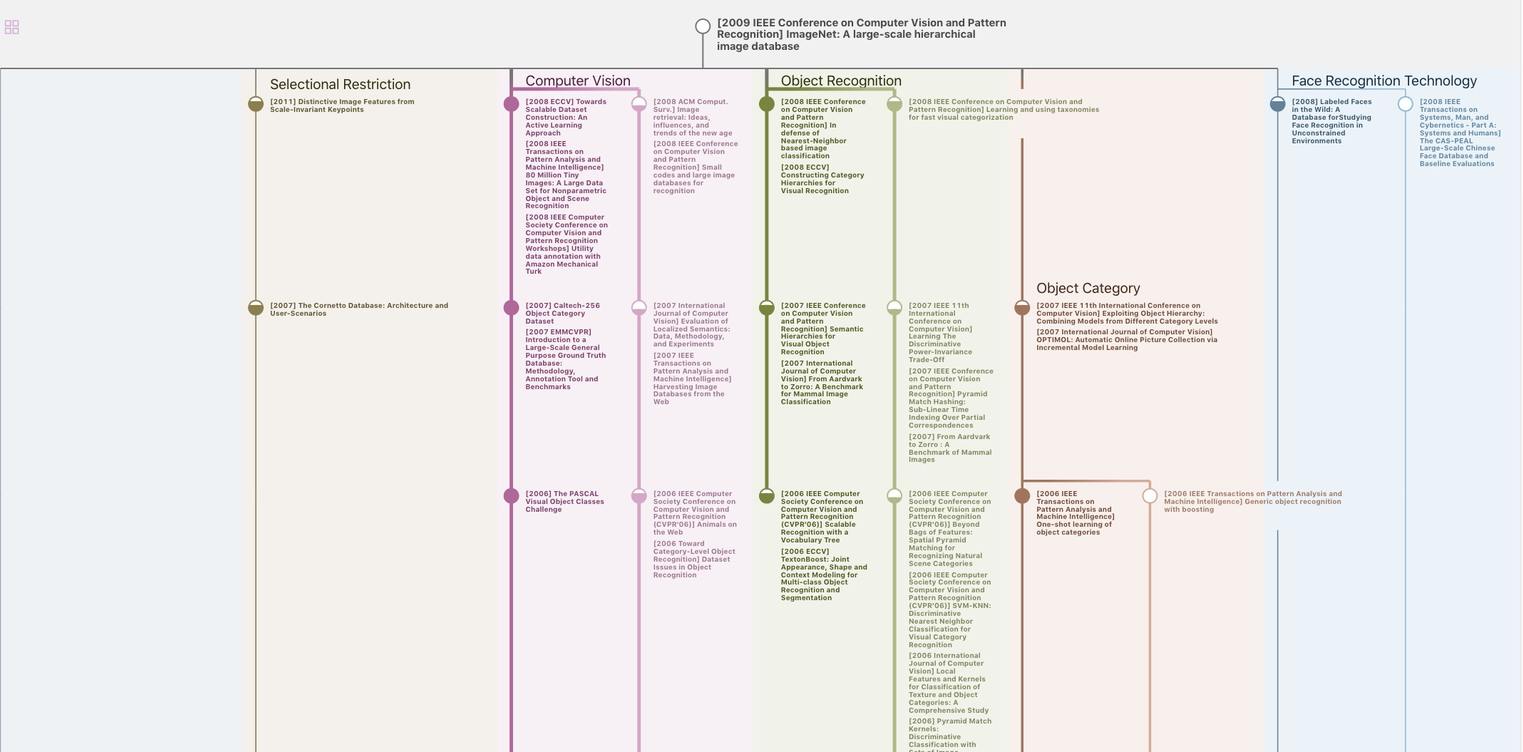
生成溯源树,研究论文发展脉络
Chat Paper
正在生成论文摘要