Sugarcane Crop Type Discrimination and Area Mapping at Field Scale Using Sentinel Images and Machine Learning Methods
Journal of the Indian Society of Remote Sensing(2022)
摘要
Crop mapping and acreage estimation are the simplest yet the most critical issues in agriculture. Remote sensing technology has been extensively used in the past few decades for executing these tasks. The objective of this study is to map sugarcane fields at a catchment level and segregate the plant and ratoon fields using the freely available Sentinel-1 and Sentinel-2 data. The study is carried out at the Kisan Sahkar Chini Mill catchment in the Saharanpur district of Uttar Pradesh. The objective was achieved by a two-step process where firstly the sugarcane fields are identified using Random Forest and SVM classifiers over temporal optical and microwave images. The most accurate result is used as a crop mask to separate the plant and ratoon fields. This was achieved by attempting a phenology based classification and spectral based classification. The results revealed that temporal Sentinel-2 data are highly competent in classifying sugarcane at farm level and segregating the plant and ratoon fields. The sugarcane crop mask was created with a kappa coefficient of 0.95 using the SVM classifier, and the plant and ratoon fields were discriminated using the Random Forest classifier with a kappa coefficient of 0.81. The sugarcane crop area was estimated to be approximately 535 acres of plant crop and 560 acres of the ratoon crop while the mill estimate was 520 acres and 540 acres, respectively. The results showed that Sentinel-2 has promising capabilities and is a convenient asset in delineating small-sized farms and classifying sugarcane and its crop types.
更多查看译文
关键词
Sugarcane mapping,Random forest,SVM,Ratoon,Sentinel,Machine learning,Farm scale,Remote sensing,Object and Pixel based
AI 理解论文
溯源树
样例
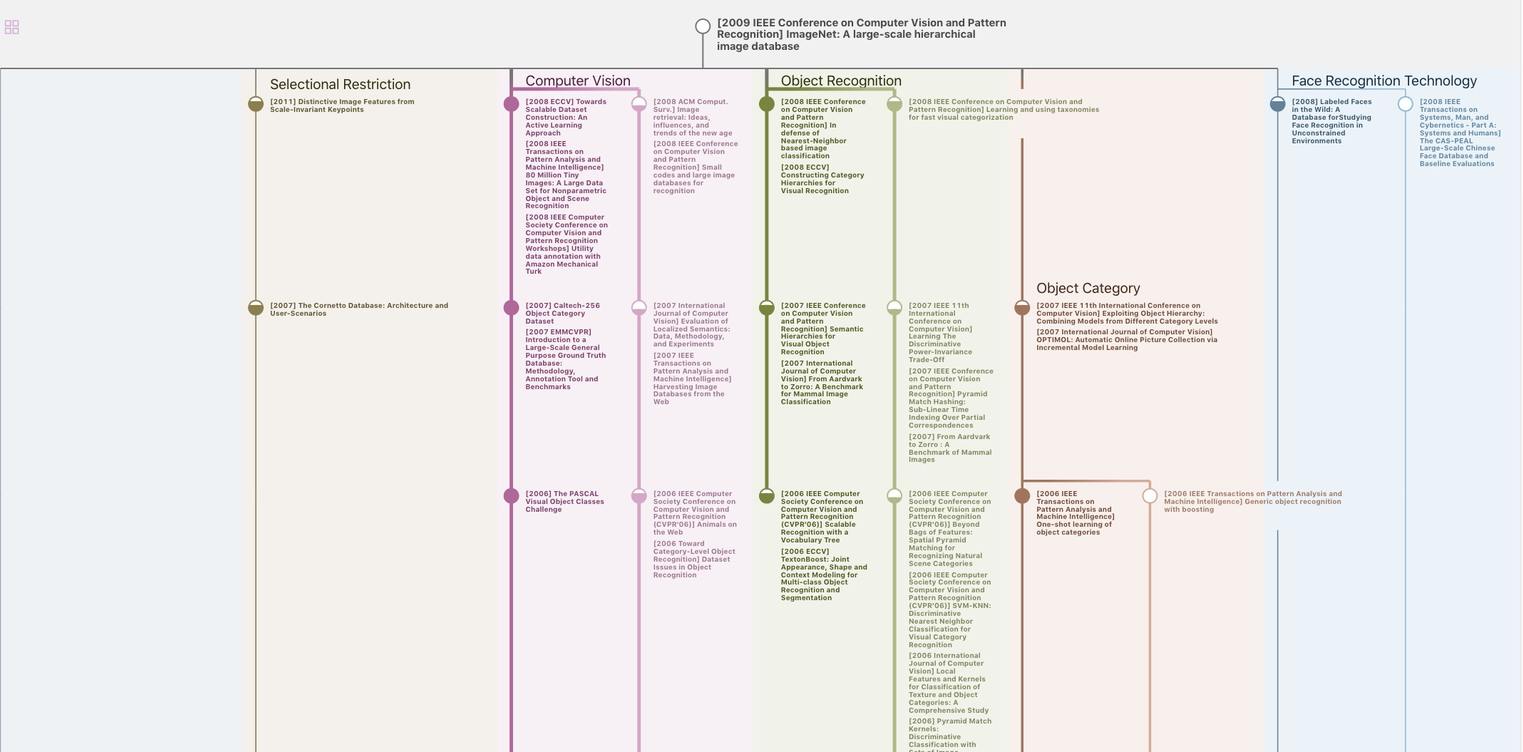
生成溯源树,研究论文发展脉络
Chat Paper
正在生成论文摘要