Application and Evaluation of an Improved LSTM Model in the Soil Moisture Prediction of Southeast Chinese Tobacco-Producing Areas
JOURNAL OF THE INDIAN SOCIETY OF REMOTE SENSING(2022)
Abstract
Aiming at the time series characteristics of soil moisture changes in the core planting area of tobacco, a soil moisture prediction model based on the combination of principal component analysis (PCA) and long-term short-term memory neural network (LSTM) was established and evaluated for tobacco precise production management and tobacco field water-saving irrigation applications. This study proposed a method using PCA to optimize the model of LSTM to predict soil moisture in tobacco-growing areas at different time intervals. The PCA method is mainly used to reduce the dimension of the original input layer meteorological data. The data are processed by dimensionality reduction to solve the input variables, and the neural network has a larger network size, resulting in reduced efficiency. LSTM network is used to solve the defects of traditional neural network model in time series data analysis. Experimental analysis shows that the LSTM prediction model of tobacco field soil moisture using PCA method can provide good short-term and medium-term prediction. The average absolute percentage error (MAPE) of the predicted soil moisture in tobacco fields is 0.27%, which is better than the full factorial radial basis function neural network (RBF NN), recurrent neural network (RNN) and traditional LSTM. The PCA and LSTM model meets the precise management requirements of actual tobacco cultivation and provides a new method for automated water-saving irrigation control of tobacco cultivation.
MoreTranslated text
Key words
Soil moisture,Principal component analysis,Deep learning,Long short-term memory neural network,Forecast
AI Read Science
Must-Reading Tree
Example
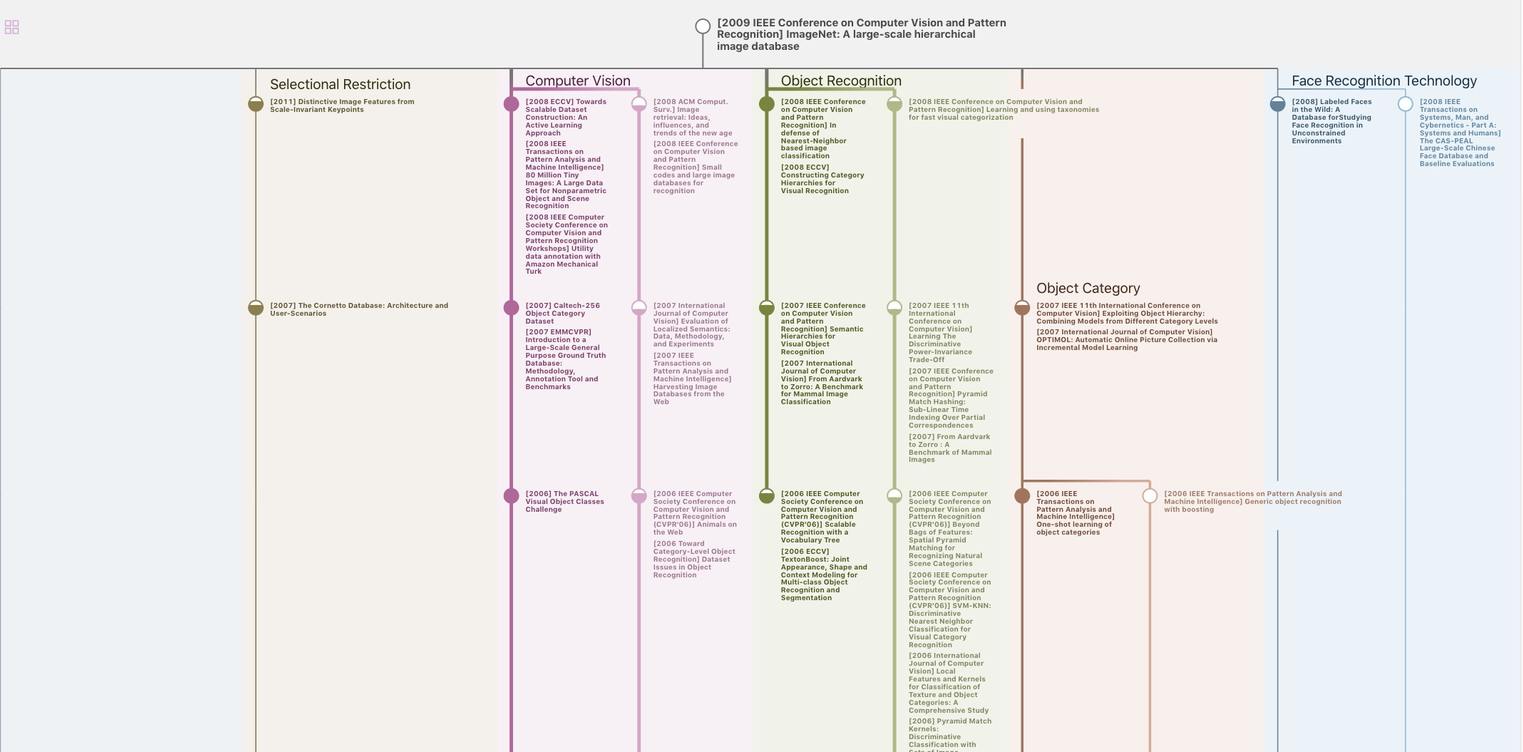
Generate MRT to find the research sequence of this paper
Chat Paper
Summary is being generated by the instructions you defined