Learning methods for structural damage detection via entropy-based sensors selection
INTERNATIONAL JOURNAL OF ROBUST AND NONLINEAR CONTROL(2022)
摘要
In this article the problem of data-driven structural damage detection is considered exploiting historical data collected from a structure. First, a novel technique based on Kalman filtering and on a combination of regression trees theory from machine learning and auto-regressive system identification from control theory is derived to build switching models that can be used to detect structural damages. A technique is also proposed leveraging principal component analysis together with the poly-exponential approach to create nonlinear models to be used for structural damage detection. Finally, a novel sensors selection algorithm based on the notions of entropy and information gain from information theory is developed to reduce the number of sensors without affecting or even improving, as it happens in our experimental setup, the model accuracy. The presented techniques are validated on three independent experimental datasets, showing that the proposed algorithms outperform previous and classical approaches, improving the prediction accuracy and the damage detection sensitivity while reducing the number of sensors.
更多查看译文
关键词
fault detection, machine learning, regression trees, sensor selection, structural damage detection, structural health monitoring
AI 理解论文
溯源树
样例
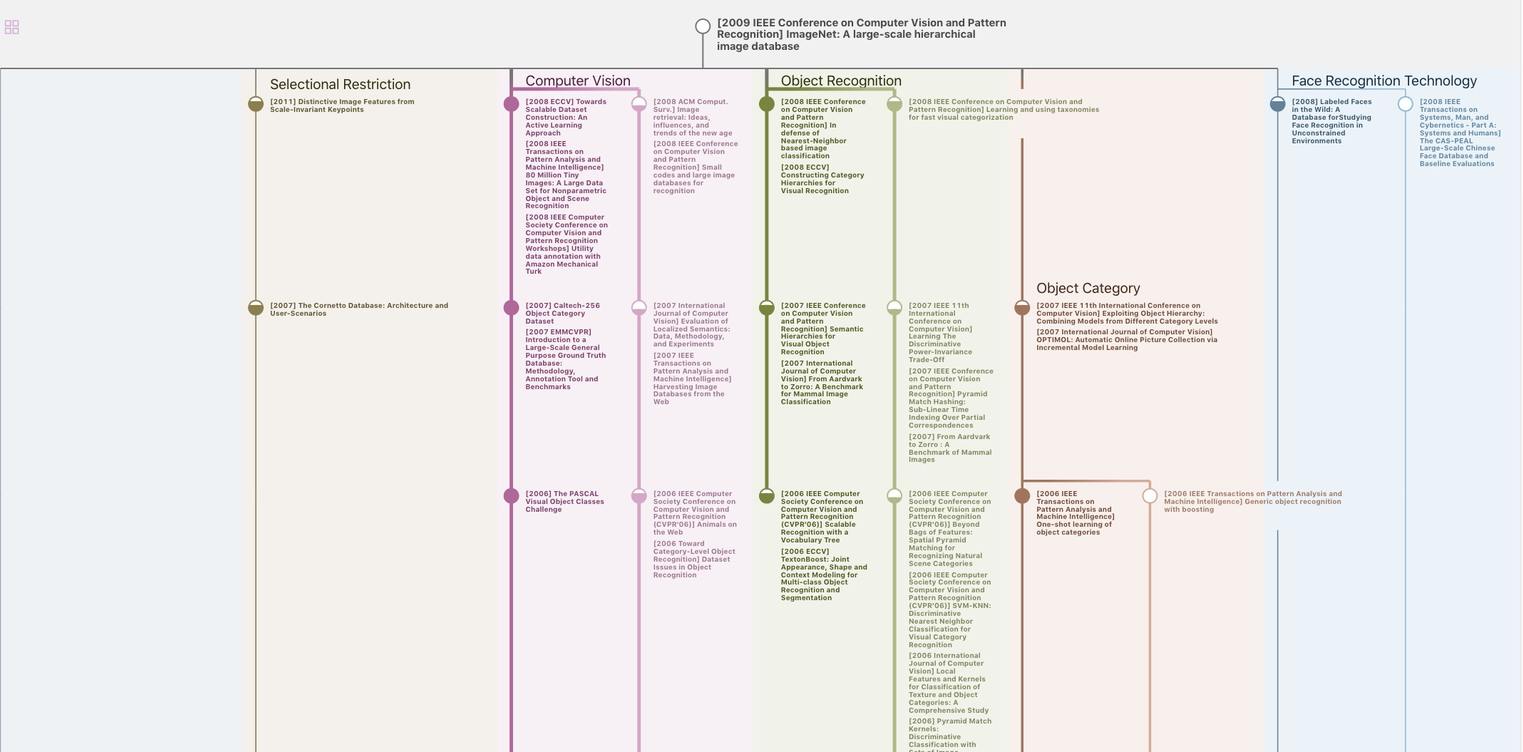
生成溯源树,研究论文发展脉络
Chat Paper
正在生成论文摘要