Real-time digital twin-based optimization with predictive simulation learning
JOURNAL OF SIMULATION(2024)
摘要
Digital twinning presents an exciting opportunity enabling real-time optimization of the control and operations of cyber-physical systems (CPS) with data-driven simulations, while facing prohibitive computational burdens. This paper introduces a method, Sequential Allocation using Machine-learning Predictions as Light-weight Estimates (SAMPLE) to address this computational challenge by leveraging machine learning models trained off-line in a predictive simulation learning setting prior to a real-time decision. SAMPLE integrates machine learning predictions with data generated by real-time execution of a digital twin in a rigorous yet flexible way, and optimally guides the digital twin simulation to achieve the computational efficiency required for real-time decision-making in a CPS. Numerical experiments demonstrate the viability of SAMPLE to select optimal decisions in real-time for CPS control and operations, compared to those of using only machine learning or simulations.
更多查看译文
关键词
Digital twin,real-time simulation optimisation,predictive simulation learning,machine learning,cyber-physical systems
AI 理解论文
溯源树
样例
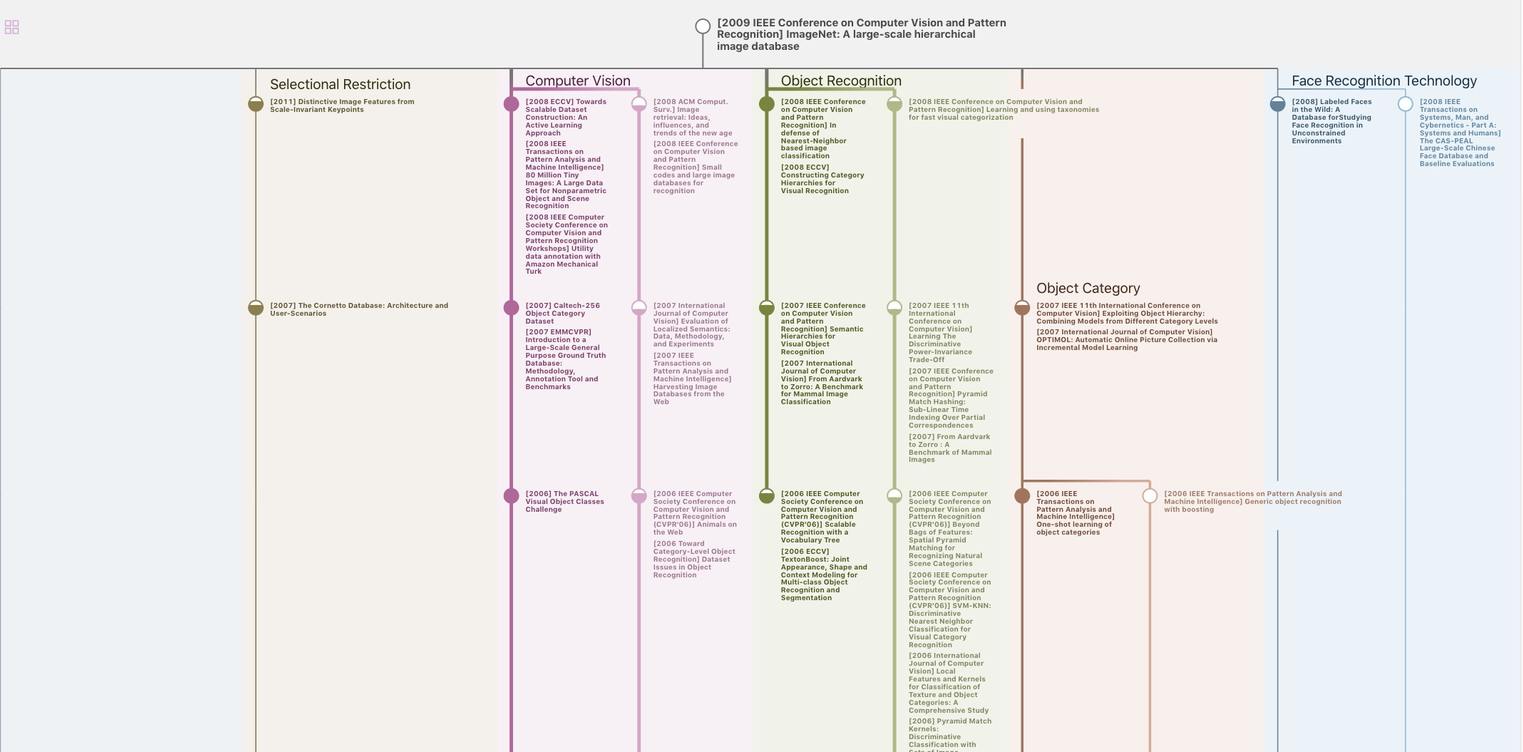
生成溯源树,研究论文发展脉络
Chat Paper
正在生成论文摘要