Conditional Generative Adversarial Networks for Dynamic Control-Parameter Selection in Power Systems
IEEE ACCESS(2022)
摘要
This paper describes the novel application of conditional Generative Adversarial Networks (cGANs) for real-time stability region determination (SRD) in power systems. As the network configuration changes during the course of operation, the availability of the stability region would enable the operator to suitably tune the control parameters to maintain stability while maximizing the dynamic performance. Here, the implementation of the cGANs-based SRD is described using transmission and microgrid case studies, where it is demonstrated to adaptively estimate the stability region for different network configurations with high accuracy. It is also shown that the cGANs approach has a significantly shorter execution time as compared to the conventional model-based method, demonstrating its value for real-time use in practical power systems.
更多查看译文
关键词
Power system stability, Training, Generators, Numerical stability, Real-time systems, Power system dynamics, Circuit stability, Control parameter tuning, distributed generation, generative adversarial networks (GANs), model-free approach, real-time control, small-signal stability
AI 理解论文
溯源树
样例
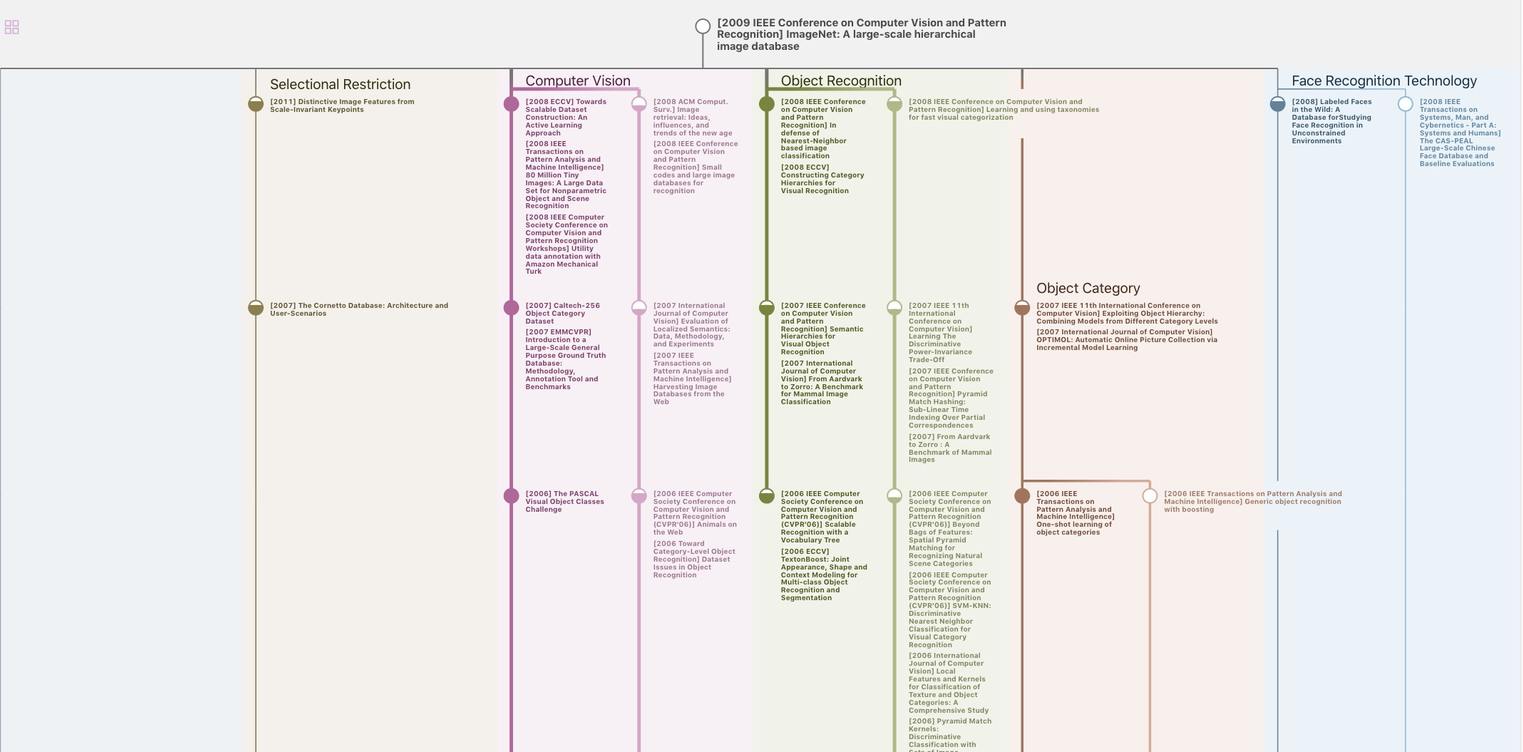
生成溯源树,研究论文发展脉络
Chat Paper
正在生成论文摘要