Learning-based T-sHDP(lambda) for optimal control of a class of nonlinear discrete-time systems
INTERNATIONAL JOURNAL OF ROBUST AND NONLINEAR CONTROL(2022)
摘要
This article investigates the optimal control problem via reinforcement learning for a class of nonlinear discrete-time systems. The nonlinear system under consideration is assumed to be partially unknown. A new learning-based algorithm, T-step heuristic dynamic programming with eligibility traces (T-sHDP(lambda)), is proposed to tackle the optimal control problem for such partially unknown system. First, the concerned optimal control problem is turned into its equivalence problem, that is, solving a Bellman equation. Then, the T-sHDP(lambda) is utilized to get an approximate solution of Bellman equation, and a rigorous convergence analysis is also conducted as well. Instead of the commonly used single step update approach, the T-sHDP(lambda) stores finite step past returns by introducing a parameter, and then utilizes these knowledge to update the value function (VF) of multiple moments synchronously, so as to achieve higher convergence speed. For implementation of T-sHDP(lambda), a neural network-based actor-critic architecture is applied to approximate VF and optimal control scheme. Finally, the feasibility of the algorithm is demonstrated by two illustrative simulation examples.
更多查看译文
关键词
eligibility traces (ET), heuristic dynamic programming (HDP), learning-based optimal control, value iteration
AI 理解论文
溯源树
样例
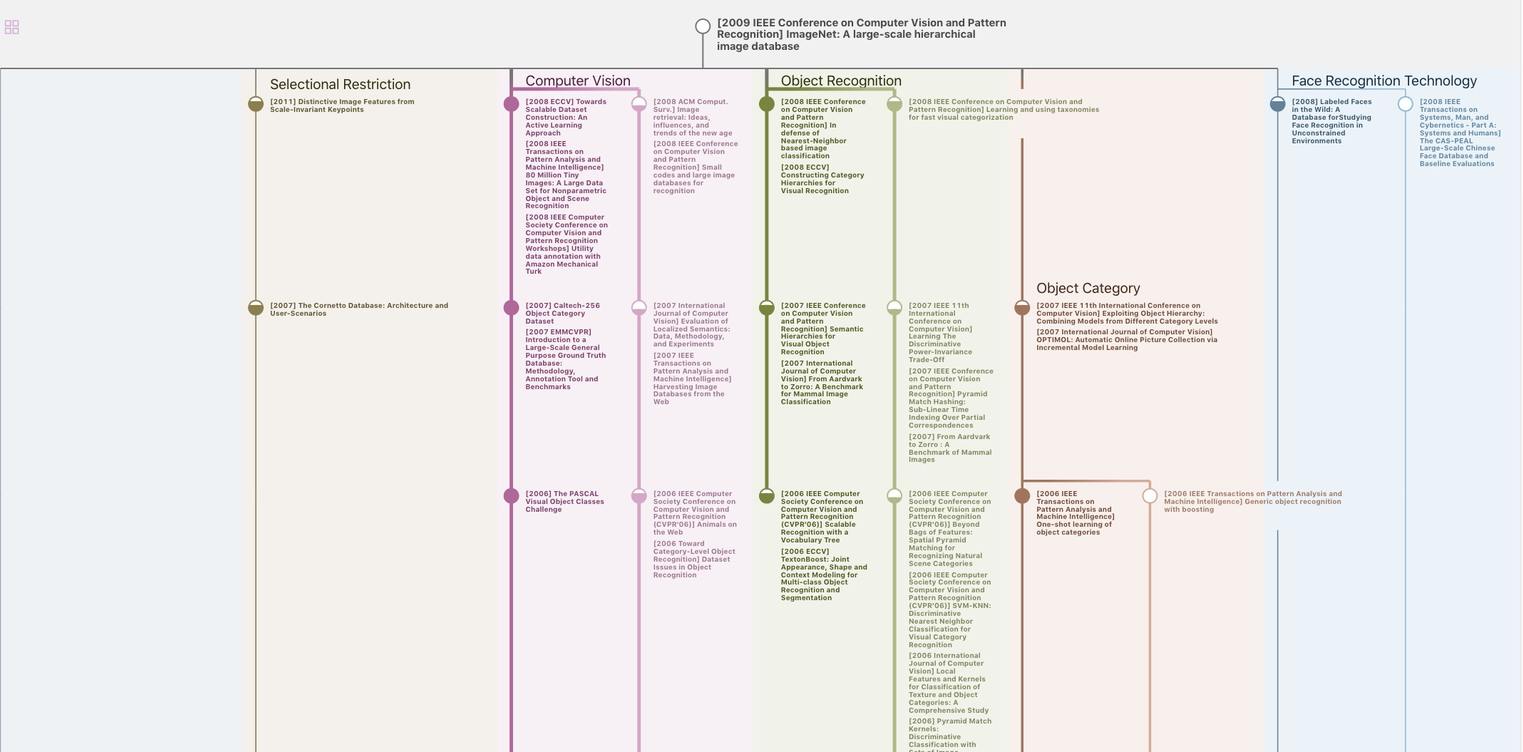
生成溯源树,研究论文发展脉络
Chat Paper
正在生成论文摘要