MRlogP: Transfer Learning Enables Accurate logP Prediction Using Small Experimental Training Datasets
PROCESSES(2021)
摘要
Small molecule lipophilicity is often included in generalized rules for medicinal chemistry. These rules aim to reduce time, effort, costs, and attrition rates in drug discovery, allowing the rejection or prioritization of compounds without the need for synthesis and testing. The availability of high quality, abundant training data for machine learning methods can be a major limiting factor in building effective property predictors. We utilize transfer learning techniques to get around this problem, first learning on a large amount of low accuracy predicted logP values before finally tuning our model using a small, accurate dataset of 244 druglike compounds to create MRlogP, a neural network-based predictor of logP capable of outperforming state of the art freely available logP prediction methods for druglike small molecules. MRlogP achieves an average root mean squared error of 0.988 and 0.715 against druglike molecules from Reaxys and PHYSPROP. We have made the trained neural network predictor and all associated code for descriptor generation freely available. In addition, MRlogP may be used online via a web interface.
更多查看译文
关键词
lipophilicity prediction, logP prediction, transfer learning, physicochemical property prediction
AI 理解论文
溯源树
样例
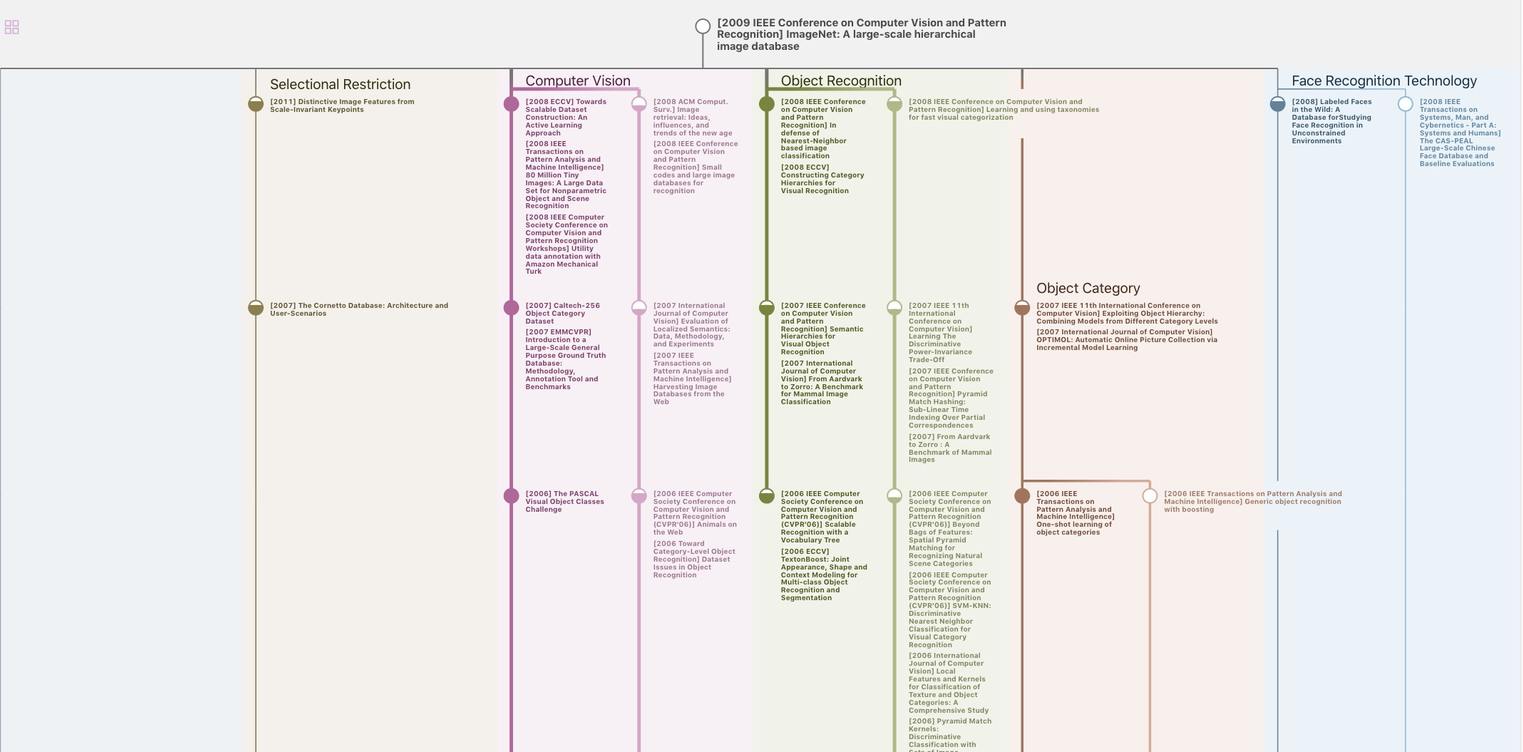
生成溯源树,研究论文发展脉络
Chat Paper
正在生成论文摘要