Machine Learning Models Based on Random Forest Feature Selection and Bayesian Optimization for Predicting Daily Global Solar Radiation
INTERNATIONAL JOURNAL OF RENEWABLE ENERGY DEVELOPMENT-IJRED(2022)
Abstract
Prediction of daily global solar radiation (H) with simple and highly accurate models would be beneficial for solar energy conversion systems. In this paper, we proposed a hybrid machine learning methodology integrating two feature selection methods and a Bayesian optimization algorithm to predict H in the city of Fez, Morocco. First, we identified the most significant predictors using two Random Forest methods of feature importance: Mean Decrease in Impurity (MDI) and Mean Decrease in Accuracy (MDA). Then, based on the feature selection results, ten models were developed and compared: (1) five standalone machine learning (ML) models including Classification and Regression Trees (CART), Random Forests (RF), Bagged Trees Regression (BTR), Support Vector Regression (SVR), and Multi-Layer Perceptron (MLP); and (2) the same models tuned by the Bayesian optimization (BO) algorithm: CART-BO, RF-BO, BTR-BO, SVR-BO, and MLP-BO. Both MDI and MDA techniques revealed that extraterrestrial solar radiation and sunshine duration fraction were the most influential features. The BO approach improved the predictive accuracy of MLP, CART, SVR, and BTR models and prevented the CART model from overfitting. The best improvements were obtained using the MLP model, where RMSE and MAE were reduced by 17.6% and 17.2%, respectively. Among the studied models, the SVR-BO algorithm provided the best trade-off between prediction accuracy (RMSE=0.4473kWh/m(2)/day, MAE=0.3381kWh/m(2)/day, and R-2=0.9465), stability (with a 0.0033kWh/m(2)/day increase in RMSE), and computational cost.
MoreTranslated text
Key words
Feature selection,Mean Decrease in Accuracy,Mean Decrease in Impurity,Bayesian optimization,Solar radiation
AI Read Science
Must-Reading Tree
Example
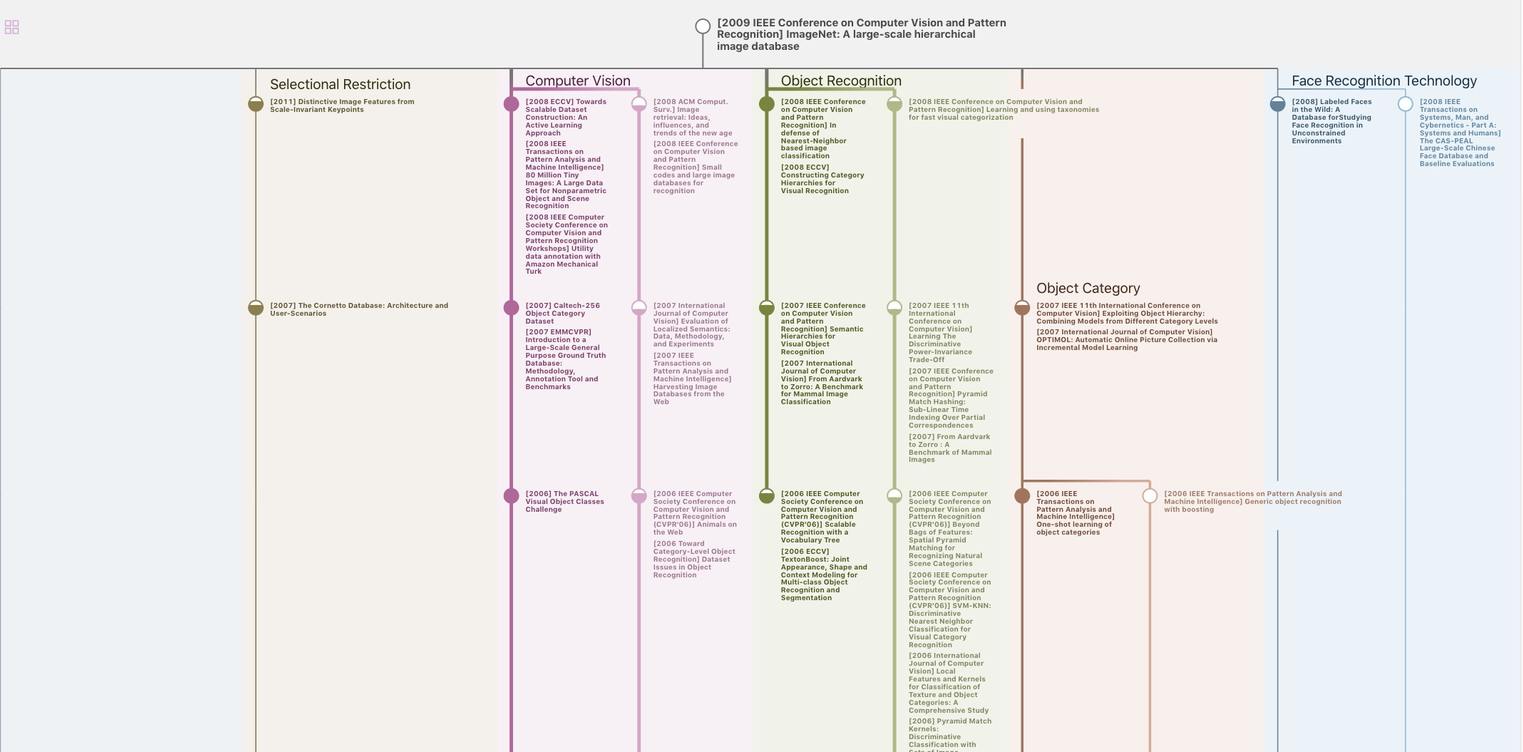
Generate MRT to find the research sequence of this paper
Chat Paper
Summary is being generated by the instructions you defined