Research on the Preliminary Prediction of Nuclear Core Design Based on Machine Learning
NUCLEAR TECHNOLOGY(2022)
摘要
The reactor core design involves the search for and detailed calculation of a large number of schemes. Four different machine learning algorithms were used in this paper: the C4.5 algorithm (an algorithm of decision trees), Support Vector Machine, Random Forest, and Multi-layer Perceptron, respectively. Uranium enrichment, the number of fuel rods containing burnable poison, and the concentration of burnable poison were taken as independent variables in the calculation. The k-eff unevenness coefficient, the radial power nonuniformity coefficient, the radial flux nonuniformity coefficient, and the core life were taken as the number of core parameters fulfilled (CPF). Machine learning models were constructed through learning the training data set, which consisted of a large number of assembly and core schemes whose nuclear design parameters were already known. Using the models, the CPF values for the unknown core data set (the test data set) were quickly predicted. The results show that the cross-validation accuracy of each algorithm was above 94% and that the C4.5 algorithm had the highest accuracy for the overall prediction of the CPF values. For the CPF value prediction of the test data set, the time for the training data set was within 10s, while the Random Forest algorithm has the highest prediction accuracy for CPF = 4 or CPF not equal 4.
更多查看译文
关键词
Machine learning, nuclear core design, decision tree, rapid prediction, random forest
AI 理解论文
溯源树
样例
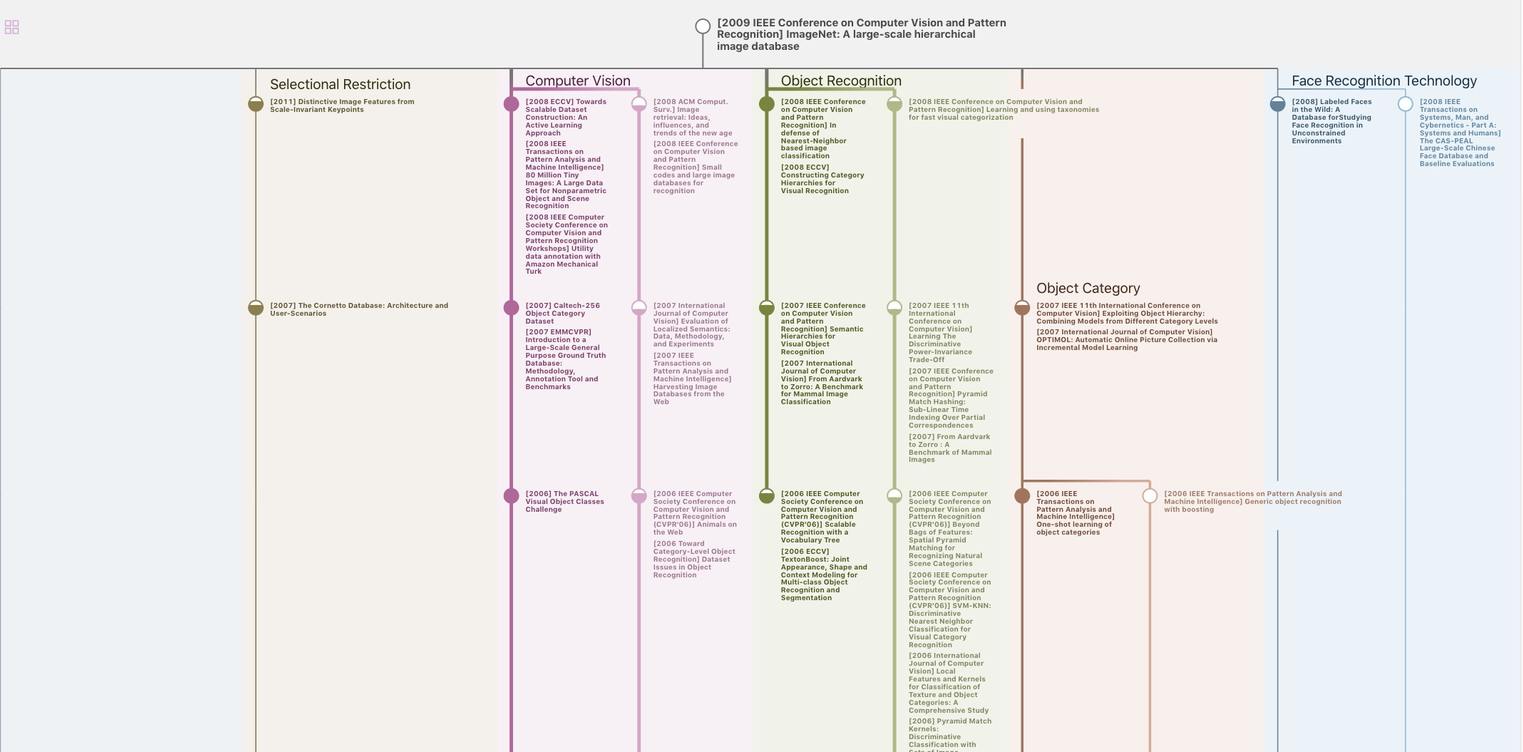
生成溯源树,研究论文发展脉络
Chat Paper
正在生成论文摘要