A reward response game in the blockchain-powered federated learning system
INTERNATIONAL JOURNAL OF PARALLEL EMERGENT AND DISTRIBUTED SYSTEMS(2022)
摘要
This paper focuses on a mobile-crowd federated learning system that includes a central server and a set of mobile devices. The server, acting as a model requester, motivates all devices to train an accurate model by paying them based on their individual contributions. Each participating device needs to balance between the training rewards and costs for profit maximization. A Stackelberg game is proposed to model interactions between the server and devices. To match with reality, our model takes the training deadline and the device-side upload time into consideration. Two reward policies, i.e. the size-based policy and accuracy-based policy, are compared. The existence and uniqueness of Stackelberg equilibrium (SE) under both policies are analyzed. We show that there is a lower bound of 0.5 on the price of anarchy in the proposed game. We extend our model by considering the uncertainty in the upload time. We also utilize the blockchain technique to ensure a truthful, trust-free, and fair system. This paper also analyzes how devices maximize their utilities when making profits via training and blockchain mining in the fixed-upload-time setting. A blockchain-powered testbed is implemented, and experiments are conducted to validate our analysis.
更多查看译文
关键词
Blockchain technique, federated learning, incentive mechanism, mobile-crowd machine learning, price of anarchy, Stackelberg game
AI 理解论文
溯源树
样例
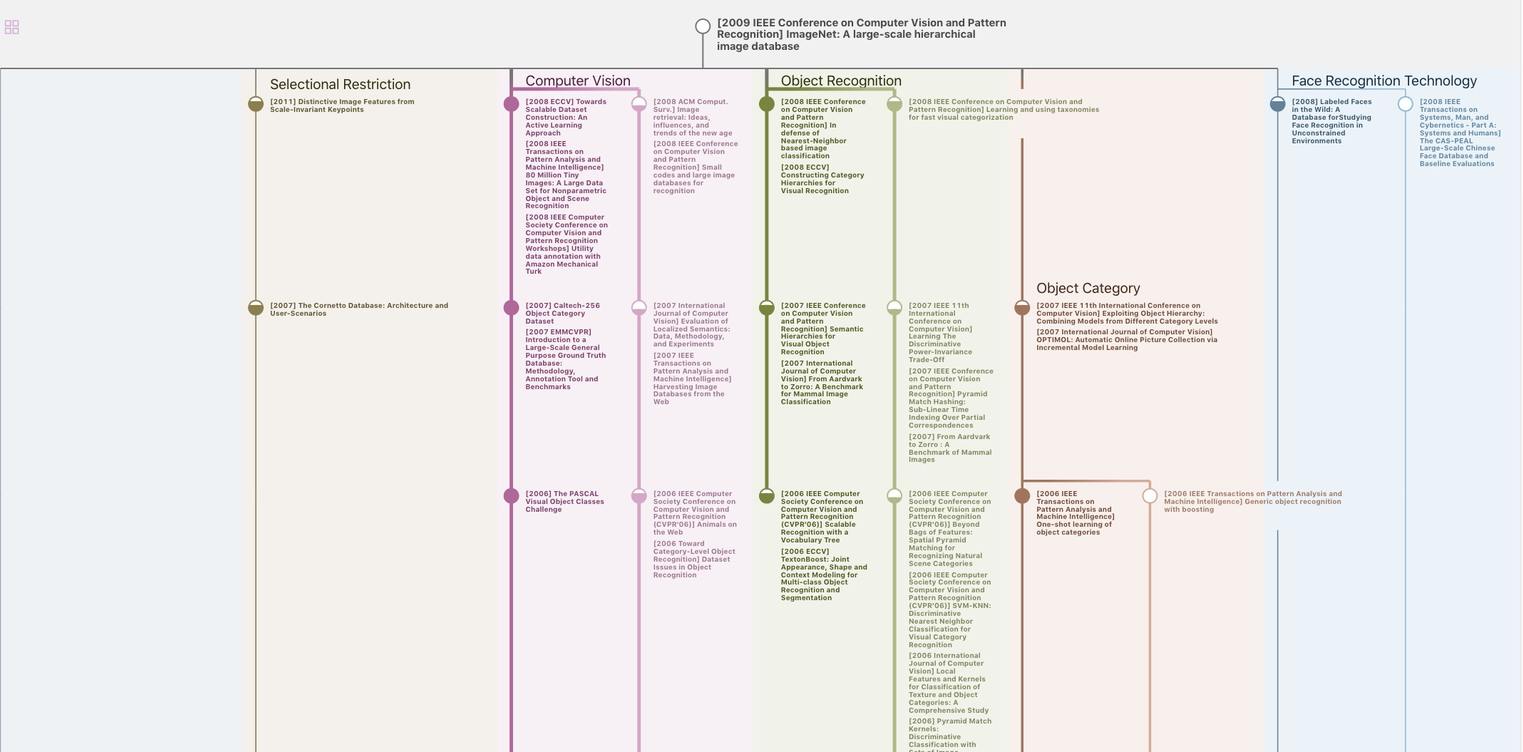
生成溯源树,研究论文发展脉络
Chat Paper
正在生成论文摘要