Crop classification for UAV visible imagery using deep semantic segmentation methods
GEOCARTO INTERNATIONAL(2022)
摘要
Unmanned aerial vehicle (UAV) has become a mainstream data collection platform in precision agriculture. For more accessible UAV-visible imagery, the high spatial resolution brings the rich geometric texture features triggered large differences in same crop image's features. We proposed an encoder-decoder's fully convolutional neural network combined with a visible band difference vegetation index (VDVI) to perform deep semantic segmentation of crop image features. This model ensures the accuracy and the generalization ability, while reducing parameters and the operation cost. A case study of crop classification was conducted in Chengdu, China, where classified four crops, namely, maize, rice, balsam pear, and Loropetalum chinese, it was shown more effective results. In addition, this study explores a fine crop classification method based on visible light features, which is feasible with low equipment cost, and has a prospect of application in crop survey based on UAV low altitude remote sensing.
更多查看译文
关键词
Crop, UAV visible images, deep semantic segmentation, encoder-decoder
AI 理解论文
溯源树
样例
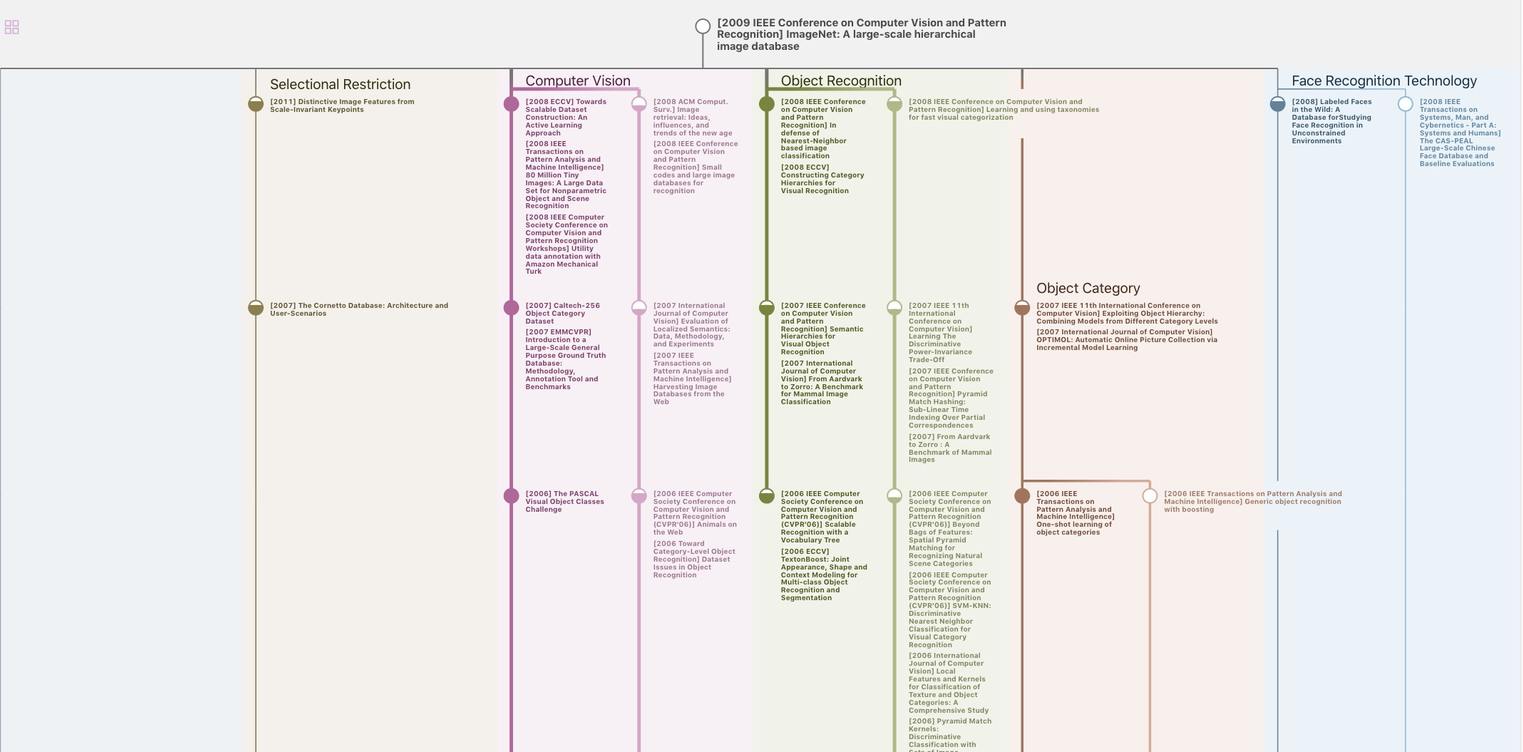
生成溯源树,研究论文发展脉络
Chat Paper
正在生成论文摘要