A Multi-precision Quantized Super-Resolution Model Framework
ALGORITHMS AND ARCHITECTURES FOR PARALLEL PROCESSING, ICA3PP 2021, PT I(2022)
摘要
Equipment's computing capability has been greatly enhanced at present, which helps deep learning achieve excellent results in various applications, such as super-resolution. However, for higher performance, lower model size and faster computing speed, model compression is widely applied to accomplish the goal. For instance, model quantization is a typical compression method, such as quantization aware training and etc. Quantization aware training can take more quantization loss due to data mapping in model training into account, clamping and approximating the data representation range when updating parameters, which introduces quantization errors into loss function. In the quantization process, we used a quantization strategy that we quantized the model in different stages of combination, and found that some stages of the two super-resolution models' generators based on SRGAN and ESRGAN showed sensitivity to quantization during the process, which greatly reduced the performance. Therefore, according to the quantization sensitivity, we use higher bits integer quantization for the sensitive stage, and get the multi-precision quantized model. For quantizing the SR model automatically, we propose a multi-precision quantization framework in this paper according to the ratio of input and output channels in every stage in the model. We also have our work tested on eight classical data sets of super-resolution. Generally speaking, both the two models' PI values approach the original model's respectively.
更多查看译文
关键词
Framework, Super-resolution model quantization, Quantization aware training, Quantization sensitivity
AI 理解论文
溯源树
样例
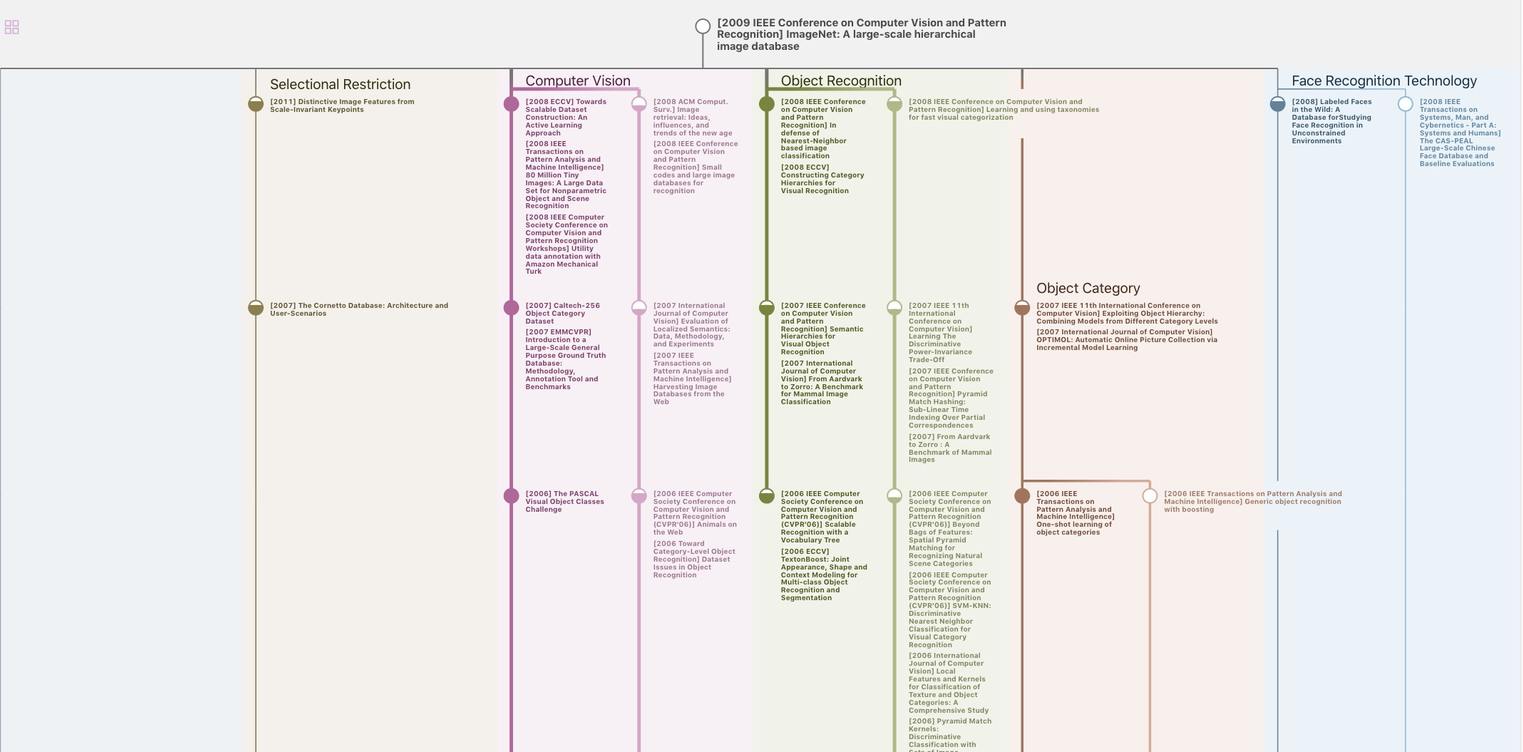
生成溯源树,研究论文发展脉络
Chat Paper
正在生成论文摘要