Effective rule mining of sparse data based on transfer learning
WORLD WIDE WEB-INTERNET AND WEB INFORMATION SYSTEMS(2022)
摘要
Rule mining is an important and challenging task in data mining. Although many state-of-art algorithms have been proposed on dense data, they are not effectively adaptive for sparse data, such as sparse heterogeneous networks. Transfer learning improves the performance of algorithms in the target domain by transferring knowledge from a similar source domain, which provides a feasible and effective method to solve the above challenge. In this paper, we propose a transfer learning-based algorithm to mine rules on sparse data effectively, named TL-ERMSD. The algorithm is capable of detecting the knowledge of a common structure as well as the rules and logics between the source and target domains. Then, rule transfer is carried out by establishing the mapping mechanism between the two domains. We conducted experiments over the heterogeneous network datasets, including the source domain dataset FB15K and the target domain dataset Yago2Sample. The results demonstrate that the proposed TL-ERMSD for rule mining has a significant advantage over the existing algorithms.
更多查看译文
关键词
Rule mining, Sparse data, Transfer learning, Heterogeneous network
AI 理解论文
溯源树
样例
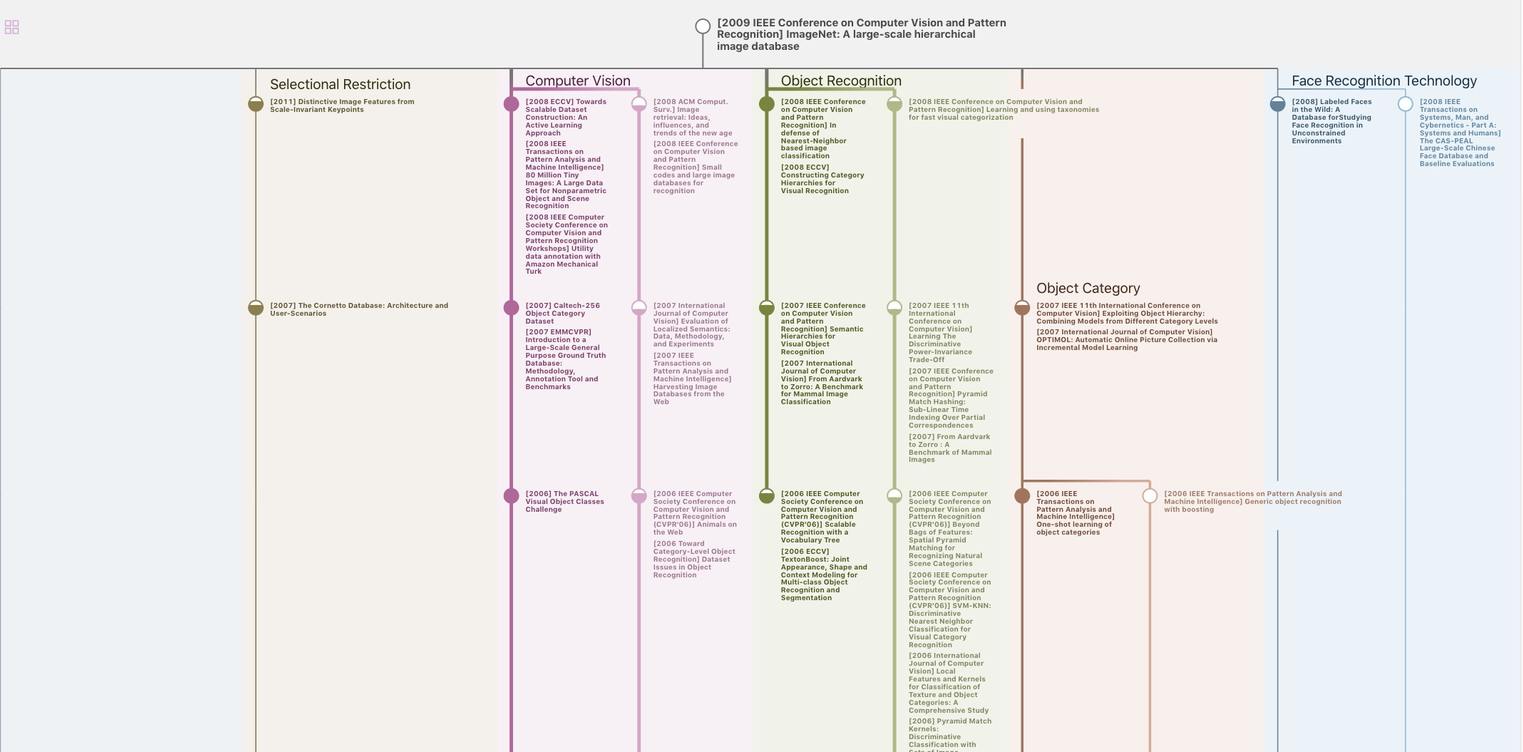
生成溯源树,研究论文发展脉络
Chat Paper
正在生成论文摘要