Multi-modal active learning with deep reinforcement learning for target feature extraction in multi-media image processing applications
MULTIMEDIA TOOLS AND APPLICATIONS(2022)
摘要
The advancement in on demand Multimedia Streaming Applications (MAS) enables faster video transmission as per the user request in various fields. This system suffers from poor speed, flexibility and efficiency in accessing and presenting the multimedia contents from the archive. It mostly undergoes delay, packet loss and congestion during data delivery. Hence, the requirement of manual annotation is required for access and retrieval but it suffers from poor retrieval accuracy over large databases. The need of automatic annotation in MAS takes the lead for increased retrieval accuracy on most similar image retrieval systems based on various low-level features. Thus, it eliminates the gap between the high-level semantics and low-level feature representation. The approach on automated annotation of images is considered dependent on the accuracy of a model while detecting edges, color, texture, shape and spatial information. In this paper, we develop an automated annotation model that retrieves visually similar images from online multimedia streams with optimal feature extraction. The automated annotation model is designed with a Multi-modal Active Learning (MAL) that uses Convolutional Recurrent Neural Network (CRNN) for automatic annotation of labels based on visually similar contents or features like edges, color, texture, shape and spatial information. Further, a Deep Reinforcement Learning (DRL) algorithm is used that increases the performance of the retrieval engine based on validating the visually extracted features. The simulation of MAL-CNN is conducted over large online streaming databases and it is then validated by DRL on an online real-time streaming. The performance is validated in terms of its retrieval accuracy, sensitivity, specificity, f-measure, geometric mean and mean absolute percentage error (MAPE). The results confirm the accuracy of the proposed MAL-DRL model against conventional machine learning, reinforcement learning and deep learning automatic annotation models.
更多查看译文
关键词
Multimodal active learning, Convolutional neural network, Deep reinforcement learning, Feature extraction, Multimedia Streaming Systems
AI 理解论文
溯源树
样例
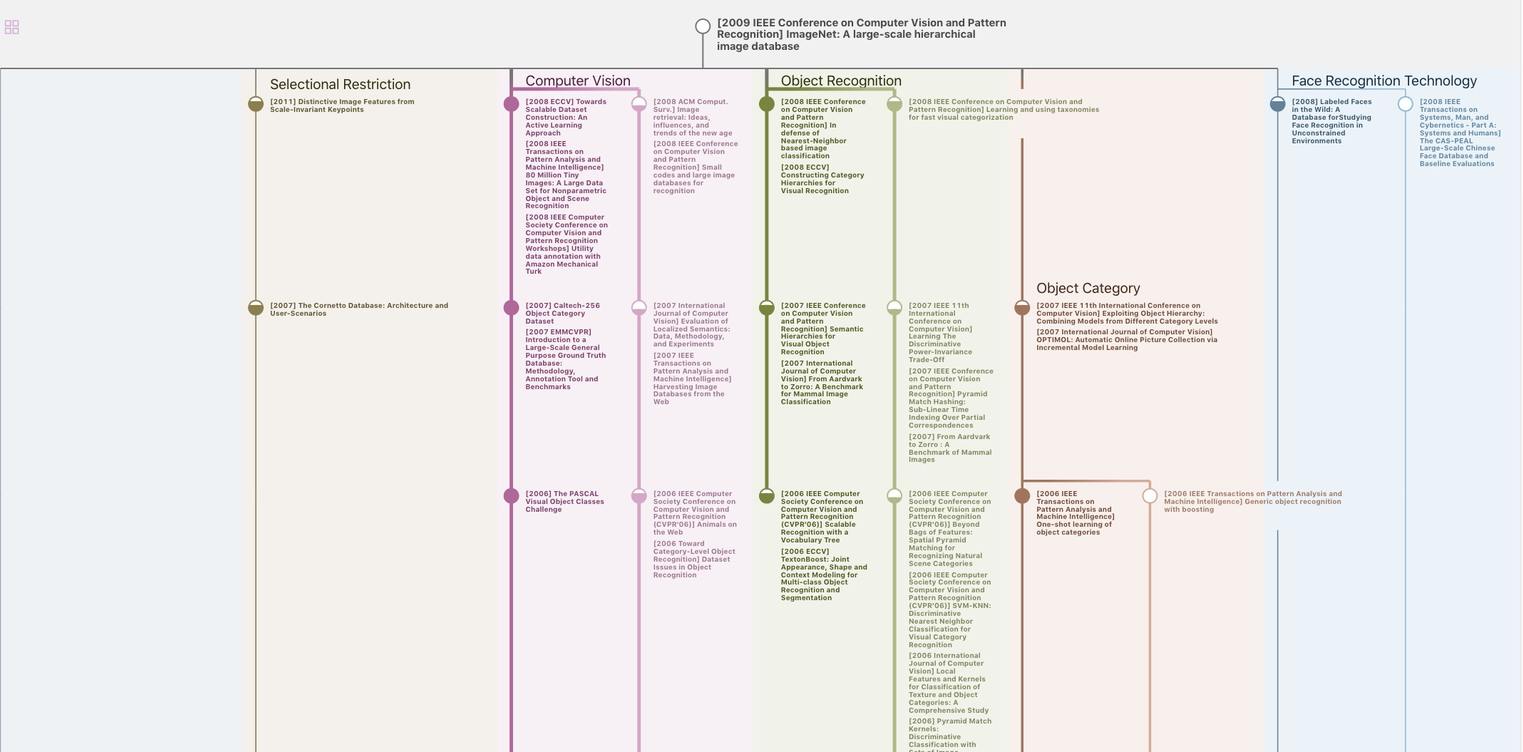
生成溯源树,研究论文发展脉络
Chat Paper
正在生成论文摘要