Development of metro track geometry fault diagnosis convolutional neural network model based on car-body vibration data
PROCEEDINGS OF THE INSTITUTION OF MECHANICAL ENGINEERS PART F-JOURNAL OF RAIL AND RAPID TRANSIT(2022)
Abstract
Traditional track geometry fault diagnosis methods, such as track geometry car, track detection trolley and manual measurement, suffer from low detection frequency. Therefore, using vibration data from in-service metro vehicles to diagnose metro track geometry faults can improve the efficiency of metro track condition detection. Hence, a metro track geometry fault diagnosis model using car-body vibration was established in this study based on a convolutional neural network model, and the effects of the structural hyperparameters on the model performance were analysed. First, the problem of car-body vibration data collection was addressed, and a mileage-locating model without the global navigation satellite system was developed. Then, the metro track geometry fault diagnosis model was derived using a convolutional neural network whose architecture is determined by four hyperparameters. Finally, a case study was performed using the Beijing Subway 1 track geometry car detection data and car-body vibration data. The results demonstrated the effectiveness of the proposed model.
MoreTranslated text
Key words
metro, track, car-body vibration, track geometry fault, convolutional neural network
AI Read Science
Must-Reading Tree
Example
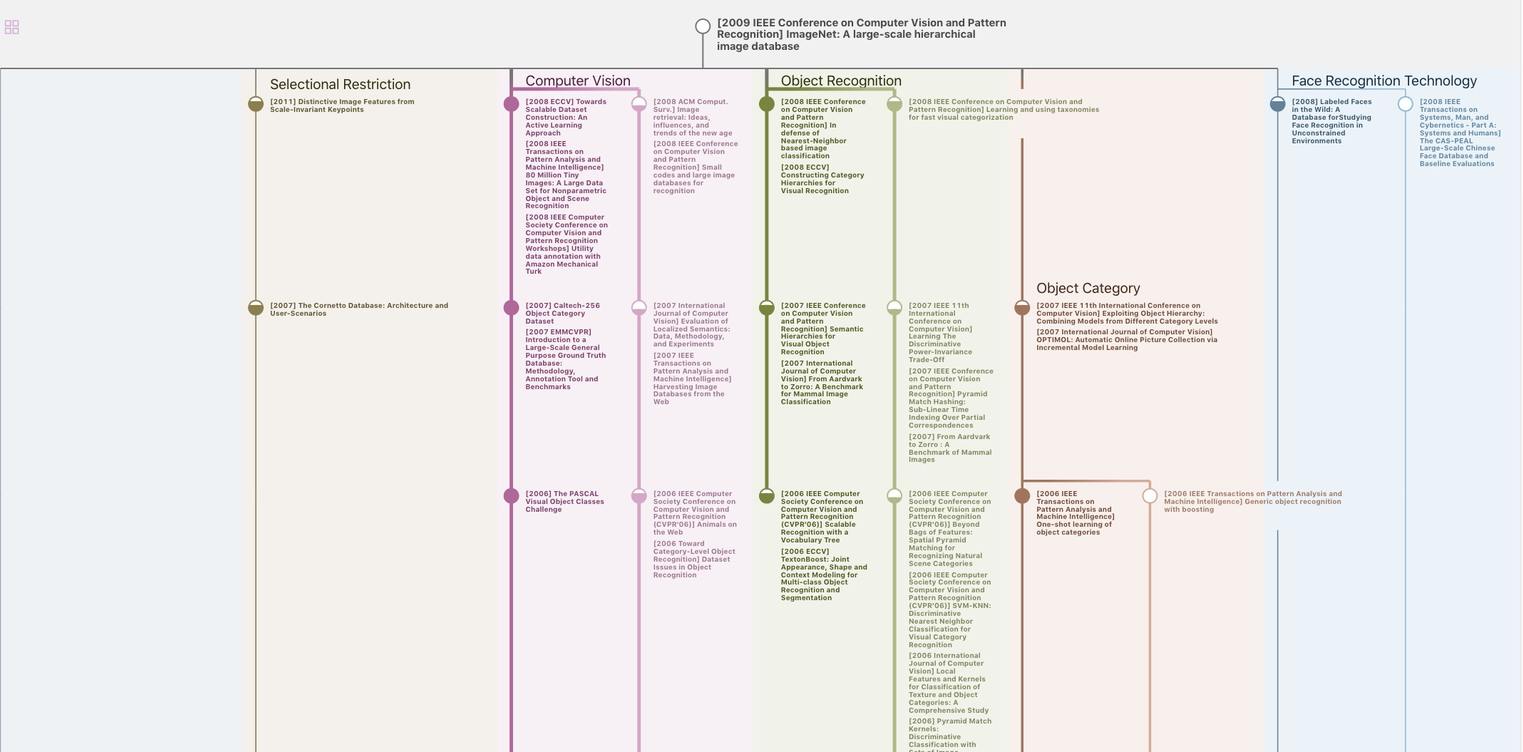
Generate MRT to find the research sequence of this paper
Chat Paper
Summary is being generated by the instructions you defined