Adaptive Differential Evolution With Information Entropy-Based Mutation Strategy
IEEE ACCESS(2021)
摘要
In order to balance the exploration and exploitation ability of differential evolution (DE), different mutation strategy for different evolutionary stages may be effective. An adaptive differential evolution with information entropy-based mutation strategy (DEIE) is proposed to divide the evolutionary process reasonably. In DEIE, the number of Markov states deduced from the crowding strategy is determined first and then the transition matrix between states is inferred from the historical evolutionary information. Based on the above-mentioned knowledge, the Markov state model is constructed. The evolutionary process is divided into exploration and exploitation stages dynamically using the information entropy derived from the Markov state model. Consequently, stage-specific mutation operation is employed adaptively. Experiments are conducted on CEC 2013, 2014, and 2017 benchmark sets and classical benchmark functions to assess the performance of DEIE. Moreover, the proposed approach is also used to solve the protein structure prediction problem efficiently.
更多查看译文
关键词
Statistics,Sociology,Entropy,Adaptation models,Markov processes,Information entropy,Diversity methods,Differential evolution,information entropy,mutation strategy,evolutionary stages,Markov state model
AI 理解论文
溯源树
样例
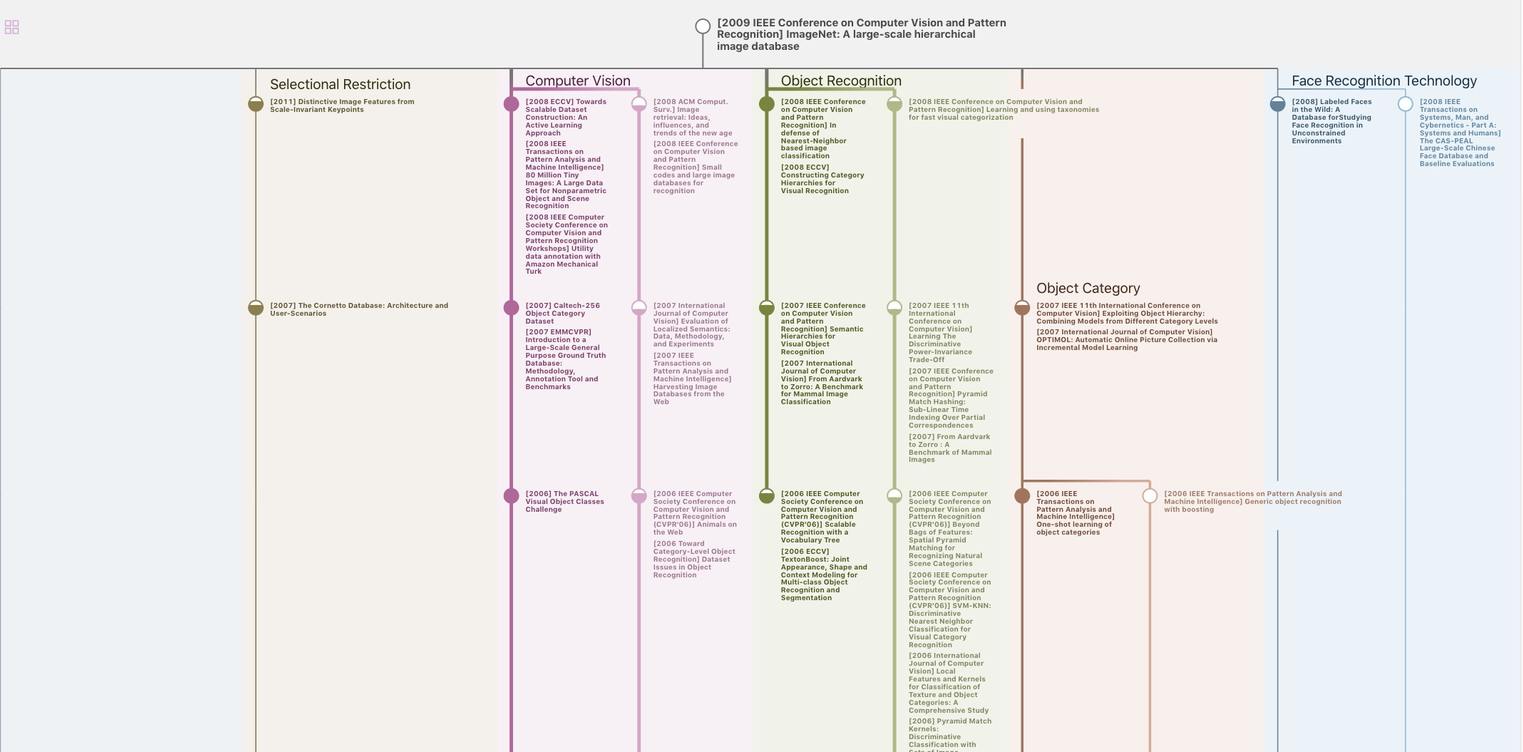
生成溯源树,研究论文发展脉络
Chat Paper
正在生成论文摘要