Robotic Embodiment of Human-Like Motor Skills via Reinforcement Learning
IEEE ROBOTICS AND AUTOMATION LETTERS(2022)
摘要
Current methods require robots to be reprogrammed for every new task, consuming many engineering resources. This work focuses on integrating real and simulated environments for our proposed "Internet of Skills," which enables robots to learn advanced skills from a small set of expert demonstrations. By expanding on recent work in the areas of Learning from Demonstrations (LfD) and Reinforcement Learning (RL), we can train robot control policies that can not only effectively complete a given task but also do so with greater performance than the expert demonstrations used to train the policy. In this work, we create simulated environments to train RL algorithms for the task of inverse kinematics and obstacle avoidance. Many state-of-the-art RL algorithms are compared, and we provide a detailed analysis of the state space and parameters chosen. Lastly, we utilize a Vicon motion tracking system and train the robot agent to follow trajectories given by a human operator. Our results show that reinforcement learning algorithms such as proximal policy optimization can develop control policies that are capable of complex control tasks that integrate with the real world, an important first step towards developing a system that can autonomously learn new skills from human demonstrations.
更多查看译文
关键词
Robots, Task analysis, Robot kinematics, Reinforcement learning, Training, Collision avoidance, Virtual environments, Telerobotics and teleoperation, reinforcement learning, transfer learning, model learning for control, collision avoidance
AI 理解论文
溯源树
样例
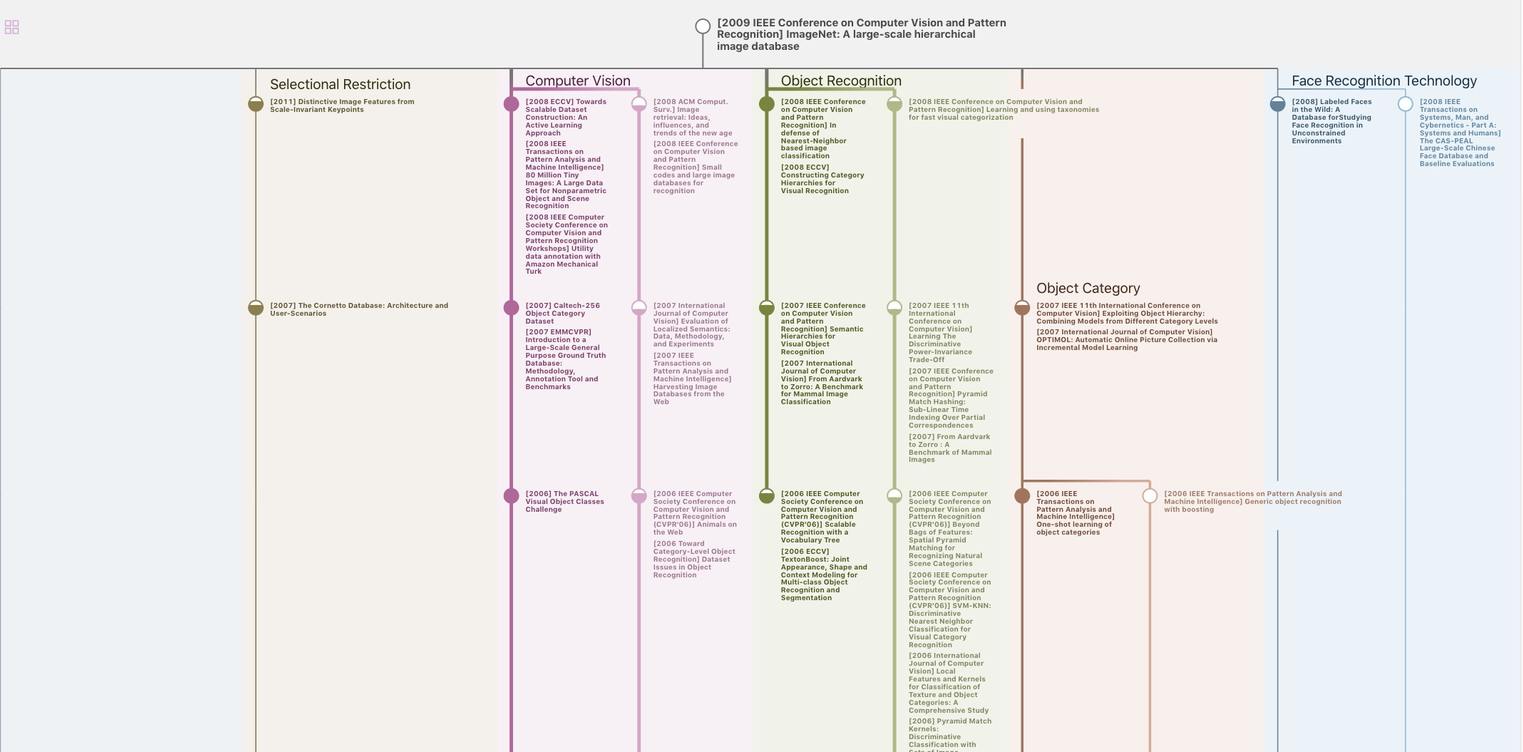
生成溯源树,研究论文发展脉络
Chat Paper
正在生成论文摘要