Bi-objective Bayesian optimization of engineering problems with cheap and expensive cost functions
ENGINEERING WITH COMPUTERS(2022)
摘要
Multi-objective optimization of complex engineering systems is a challenging problem. The design goals can exhibit dynamic and nonlinear behaviour with respect to the system’s parameters. Additionally, modern engineering is driven by simulation-based design which can be computationally expensive due to the complexity of the system under study. Bayesian optimization (BO) is a popular technique to tackle this kind of problem. In multi-objective BO, a data-driven surrogate model is created for each design objective. However, not all of the objectives may be expensive to compute. We develop an approach that can deal with a mix of expensive and cheap-to-evaluate objective functions. As a result, the proposed technique offers lower complexity than standard multi-objective BO methods and performs significantly better when the cheap objective function is difficult to approximate. In particular, we extend the popular hypervolume-based Expected Improvement (EI) and Probability of Improvement (POI) in bi-objective settings. The proposed methods are validated on multiple benchmark functions and two real-world engineering design optimization problems, showing that it performs better than its non-cheap counterparts. Furthermore, it performs competitively or better compared to other optimization methods.
更多查看译文
关键词
Multi-objective optimization, Bayesian optimization, Hypervolume, Gaussian process
AI 理解论文
溯源树
样例
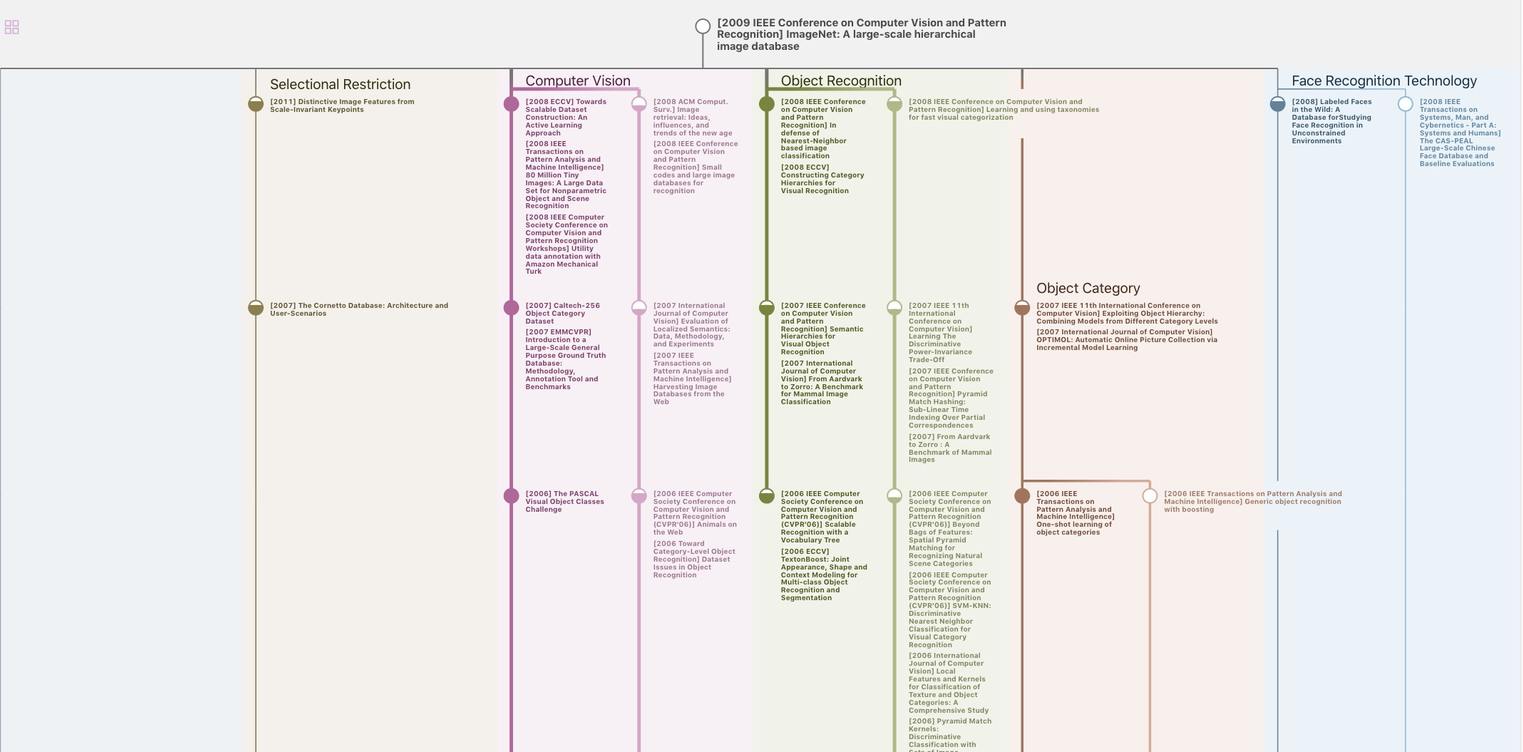
生成溯源树,研究论文发展脉络
Chat Paper
正在生成论文摘要