Deep Active Autoencoders for Outlier Detection
Neural Processing Letters(2022)
摘要
The variety and lack of labels for outliers make it difficult to establish a fixed outlier model, which facilitates unsupervised algorithms for mainstream outlier detection. However, unsupervised methods rely on the assumption that there are no outliers within the dataset or that the outliers are sporadically distributed, thus leading to unsatisfactory detection accuracy within large-scale, high-dimensional datasets, especially image datasets. In view of this, the present study proposes a novel outlier detection method, called Active Autoencoder (AAE), which can be used to break through the bottleneck of unsupervised learning. AAE improves the performance of autoencoder through use of influence-based active learning in combination with a novel way to change sample weights by expansion-shrinkage operator. Experiments on benchmark and fundus image datasets demonstrate that the proposed method achieves superior performance compared to alternatives.
更多查看译文
关键词
Outlier detection,Active learning,Autoencoder
AI 理解论文
溯源树
样例
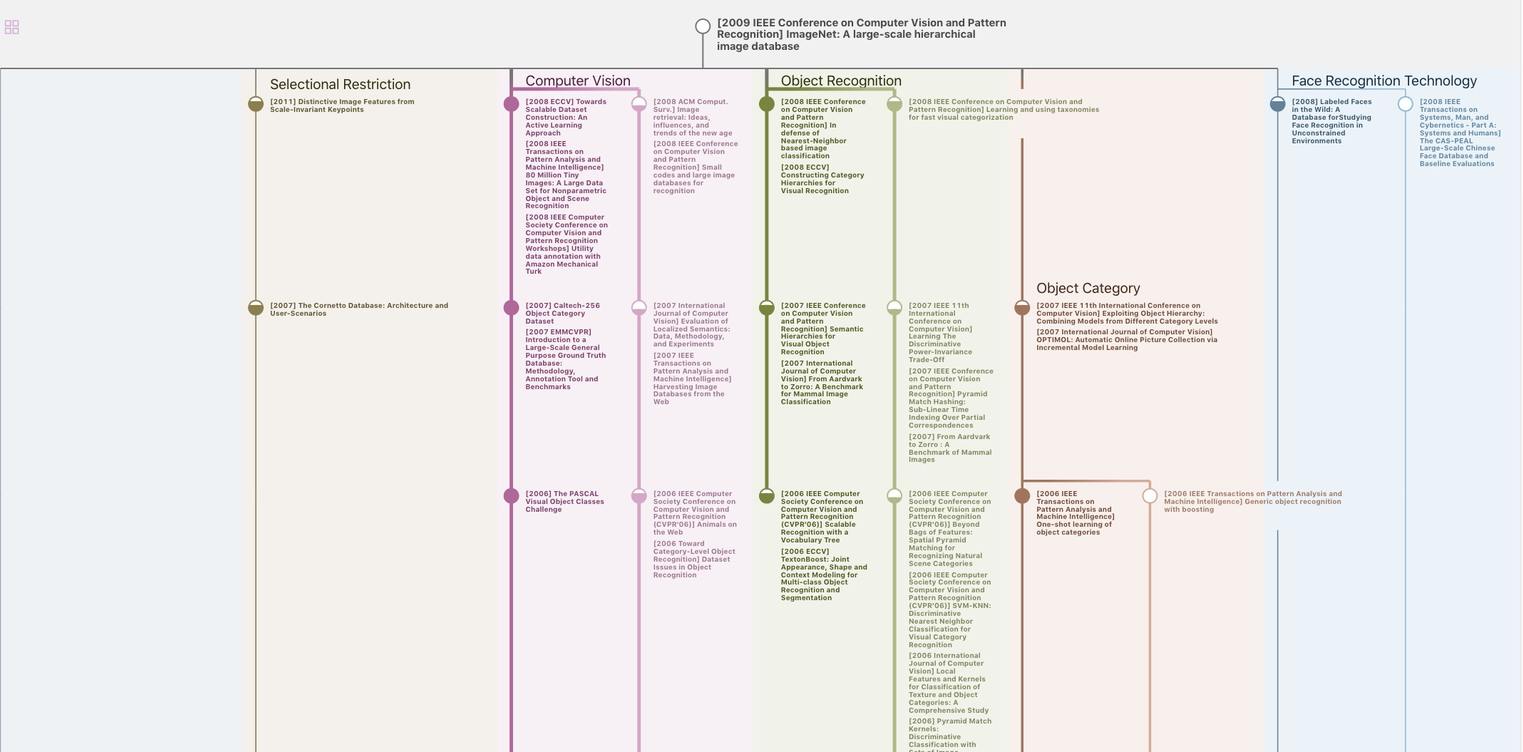
生成溯源树,研究论文发展脉络
Chat Paper
正在生成论文摘要