Polya-gamma data augmentation and latent variable models for multivariate binomial data
JOURNAL OF THE ROYAL STATISTICAL SOCIETY SERIES C-APPLIED STATISTICS(2022)
摘要
New Zealand police has long been suspected of systematic bias against the indigenous Maori. One resource available to investigate this possibility is the annual counts of police apprehensions and prosecutions, by offence type. However, model specification/fitting is complicated as these data are constrained counts, interdependent and multivariate. For example, there are limited options for factor models beyond continuous or binary data. This is a serious limitation for in our dataset, while measurements are clustered, different individuals are measured at each variable. Focusing on principal component/factor analysis representations, we show that under the canonical logit link, latent variable models can be fitted via Gibbs sampling, to multivariate binomial data of arbitrary trial size by applying Polya-gamma augmentation to the binomial likelihood. We demonstrate that this modelling approach, by incorporating shrinkage, will produce a fit with lower mean square error than techniques based on deviance minimization commonly employed for binary datasets. By exploring theoretical properties of the proposed models, we demonstrate a larger range of latent structures can be estimated and the presence of hidden replication improves prediction when data are multivariate binomial, which gives us greater flexibility for investigating associations between ethnicity and prosecution probability.
更多查看译文
关键词
latent variable models, logit models, multivariate binomial data, Polya-gamma data augmentation, principal components, factor analysis
AI 理解论文
溯源树
样例
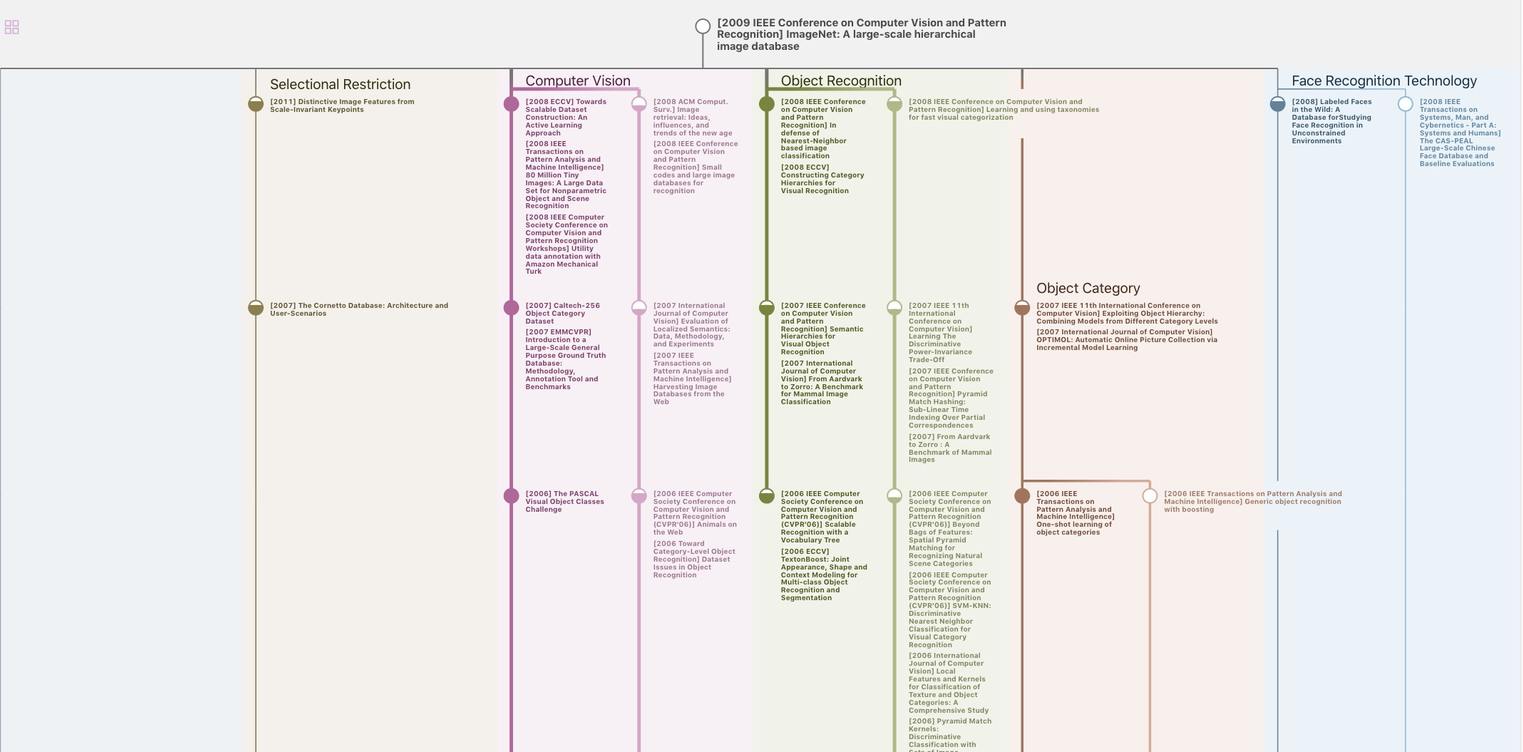
生成溯源树,研究论文发展脉络
Chat Paper
正在生成论文摘要