Spatial prediction of soil surface properties in an arid region using synthetic soil image and machine learning
GEOCARTO INTERNATIONAL(2022)
Abstract
Evaluation of spatial variability and mapping of soil properties is critical for sustainable agricultural production in arid lands. The main objectives of the present study were to spatialize soil organic carbon (SOC), soil particle size distribution(clay, sand, and silt contents), and calcium carbonate equivalent (CCE) by integrating multisource environmental covariates, including digital elevation model (DEM) and remote sensing data by machine learning (Cubist, Cu and random forest, RF) in an arid region of Iran. Additionally, Synthetic Soil Images (SySI) were achieved from multi-temporal images of bare soil pixels based on Landsats 4, 5, 7, 8, and a DEM. Three hundred topsoil samples (0-30 cm depth) were collected based on the conditioned Latin hypercube sampling (cLHS) approach in Afzar district, Fars province, southern Iran. The models were calibrated and validated by the 10-fold cross-validation approach, and the performance was evaluated using root mean square error (RMSE), the ratio of the performance to interquartile distance (RPIQ), and coefficient of determination (R-2). Also, the prediction accuracy was assessed by the relative RMSE (rRMSE). The performance of the best models based on the RPIQ index showed that the model for predicting clay (1.89) had a good prediction, for sand (1.64), SOC (1.55), and CCE (1.59) had a fair prediction, while the model for silt (1.13) performed poorly. We found that the Cu and RF models had the highest and lowest prediction accuracies for CCE (rRMSE = 14.31%) and SOC (rRMSE = 43.93%), respectively. We discovered that a combination of high-quality RS data (SySI) and variables derived from DEM were reasonably able to predict soil properties. We revealed a strong promise to enhance the accuracy of digital soil mapping, especially in regions with limited soil data. Moreover, the application of RS data can reduce the soil sampling cost and, accordingly, soil mapping.
MoreTranslated text
Key words
Spatial prediction,environmental covariates,synthetic soil image (SySI),Cubist,random forest,machine learning
AI Read Science
Must-Reading Tree
Example
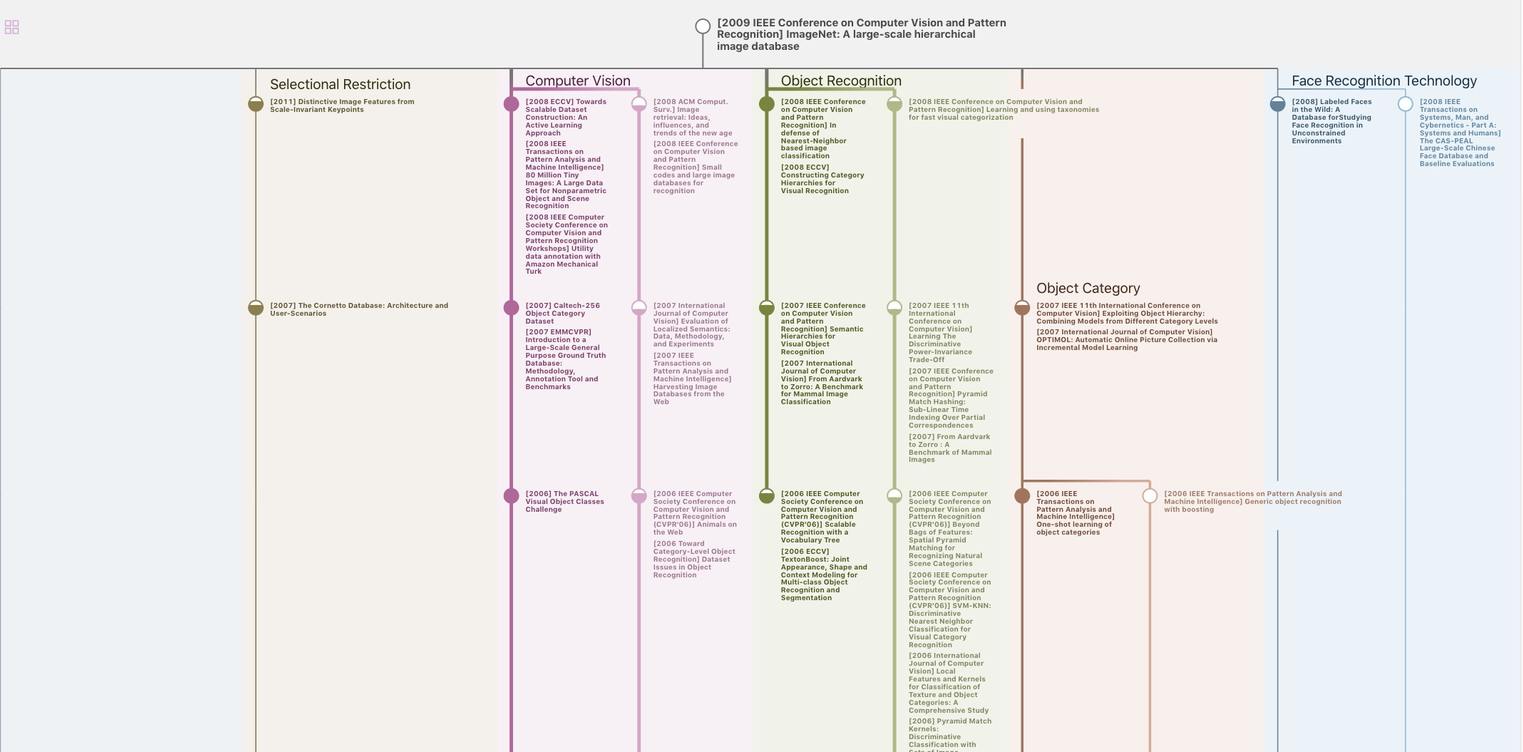
Generate MRT to find the research sequence of this paper
Chat Paper
Summary is being generated by the instructions you defined