Mapping of soil organic carbon using machine learning models: Combination of optical and radar remote sensing data
SOIL SCIENCE SOCIETY OF AMERICA JOURNAL(2022)
摘要
Soil organic C (SOC) plays an important role in soil quality. Thus, it is of great significance to know the spatial distribution characteristics of SOC. Environmental variables, such as natural predictors, remote sensing (RS) variables, and digital soil mapping approaches have been widely used in SOC prediction. However, it is still challenging to determine which methods and variables are effective to map SOC in farmland. In this study, we compare the performance of three machine learning models, including random forest, Cubist, and the support vector machine model for the prediction of SOC contents using RS variables, topographic variables, climate variables, soil property variables, and spatial position variables as environmental covariates. The recursive feature elimination (RFE) technique was used to select optimal variables. Of the 130 sampling sites of the cultivated layer that were collected in Shanggao county, 70% of the data were used for training and 30% for validation. Prediction accuracy and uncertainty was quantified using the bootstrap approach with 100 iterations. The performance of the model was verified by the mean absolute error, RMSE, R-2, and Lin's concordance correlation coefficient (CCC); the SD was used as a measure of prediction uncertainty. Results showed that the Cubist model combined with the RFE method (Cubist_RFE) had a mean R-2, RMSE, and SD of .84, 2.39 g kg(-1), and 0.73 g kg(-1), respectively, which showed the highest prediction accuracy and the lowest uncertainty. The soil property variables (soil alkaline hydrolysis N and pH) and RS variables (soil adjusted vegetation index, enhanced vegetation index, and band7 of Sentinel-2) were the controlling factors of SOC prediction. Thus, the results indicate the potential of the Cubist model in SOC prediction, and the combination of the optical and radar RS data is a viable way for predicting SOC.
更多查看译文
AI 理解论文
溯源树
样例
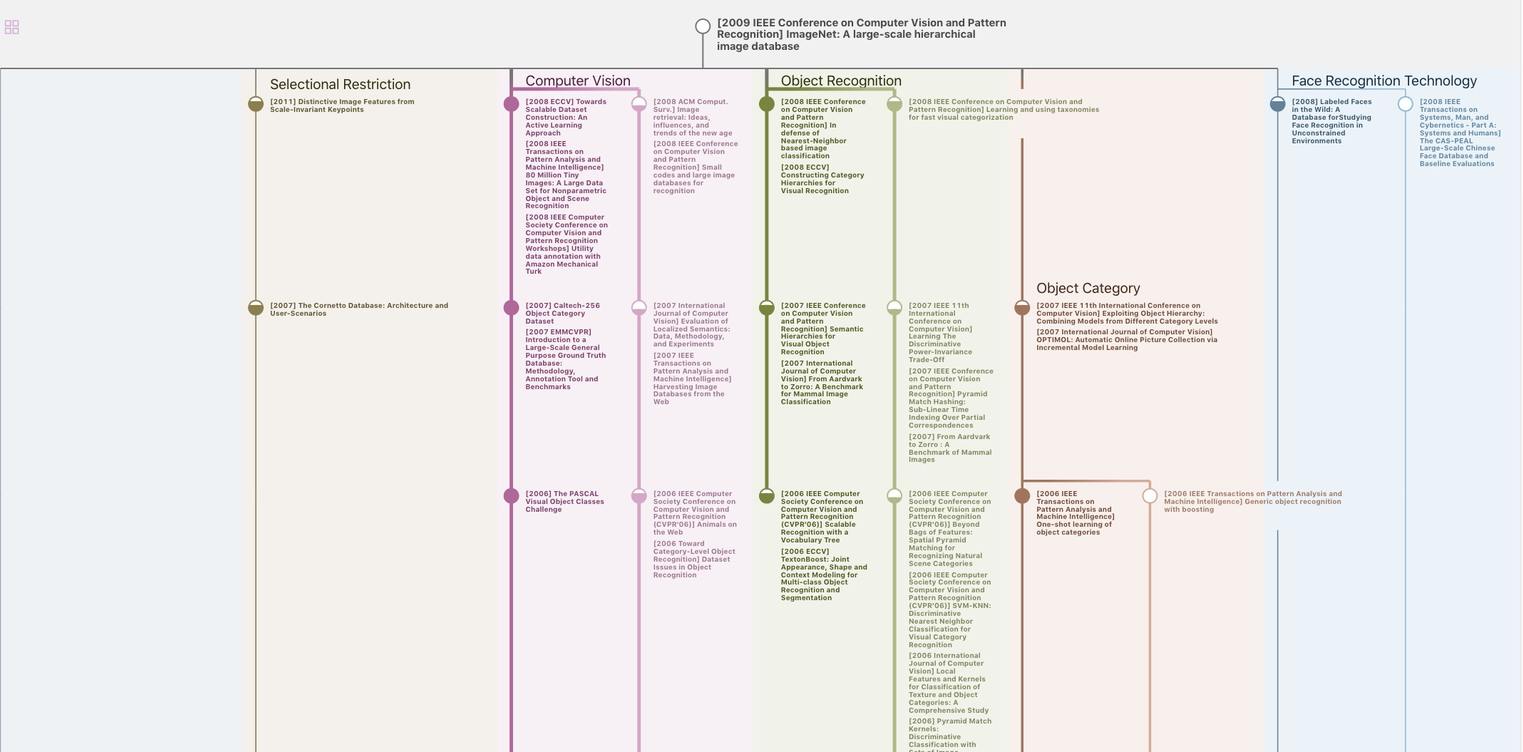
生成溯源树,研究论文发展脉络
Chat Paper
正在生成论文摘要