Effective medium crack classification on laboratory concrete specimens via competitive machine learning
Structures(2022)
摘要
With the advent rise of automation, it is now possible to trace and detect damage in structural systems with ease. Unfortunately, existing inspection methods continue to suffer on a number of fronts; i.e., laborious, costly, and mediocre performance. In order to bridge this knowledge gap, this work aims to develop an autonomous and open-source deep learning (DL) approach capable of detecting varying scales of damage (i.e., micro and macro cracks) in concrete structures. In pursuit to fine tune and optimize this approach, we carried out an investigation to examine the influence of DL various architectures, network depths, regularization techniques, and transfer learning methods. Based on our investigation, we proposed a new transfer learning method to improve the accuracy of a micro-and macro-crack DL classifier. The deployed DL architecture is based on a much improved VGG-16 model and achieved an accuracy of 99.5%, and an F1-score of 100% when examined against a comprehensive dataset and an accompanying physical testing program.
更多查看译文
关键词
Classification,Cracks,Damage detection,Machine learning,Transfer learning
AI 理解论文
溯源树
样例
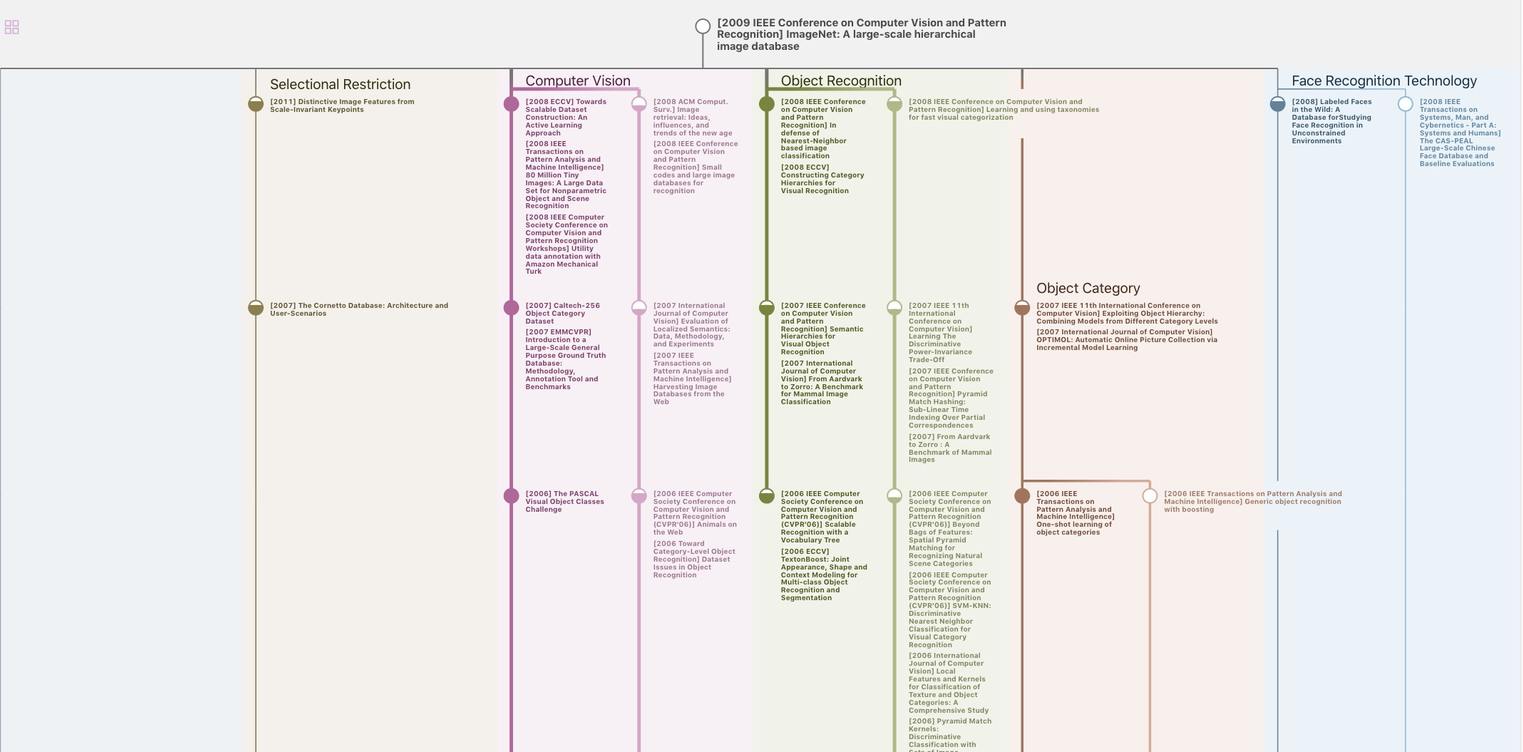
生成溯源树,研究论文发展脉络
Chat Paper
正在生成论文摘要