A Feature and Classifier Study for Appliance Event Classification
SUSTAINABLE ENERGY FOR SMART CITIES, SESC 2021(2022)
Abstract
The shift towards advanced electricity metering infrastructure gained traction because of several smart meter roll-outs during the last decade. This increased the interest in Non-Intrusive Load Monitoring. Nevertheless, adoption is low, not least because the algorithms cannot simply be integrated into the existing smart meters due to the resource constraints of the embedded systems. We evaluated 27 features and four classifiers regarding their suitability for event-based NILM in a standalone and combined feature analysis. Active power was found to be the best scalar and WaveForm Approximation the best multidimensional feature. We propose the feature set [P, cos Phi ,TRI, WFA] in combination with a Random Forest classifier. Together, these lead to F-1-scores of up to 0.98 on average across four publicly available datasets. Still, feature extraction and classification remains computationally lightweight and allows processing on resource constrained embedded systems.
MoreTranslated text
Key words
NILM, Non-Intrusive Load Monitoring, Feature evaluation, Appliance classification
AI Read Science
Must-Reading Tree
Example
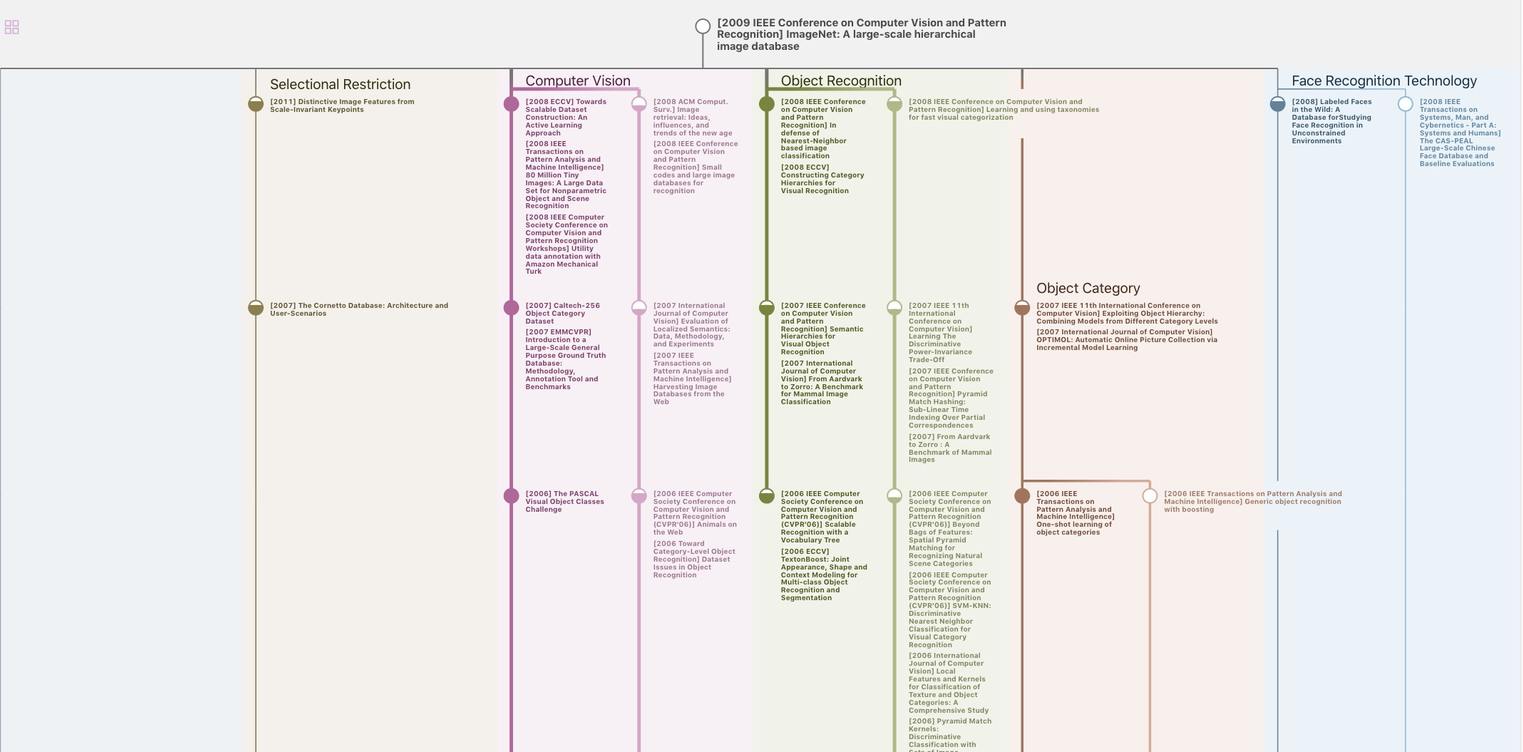
Generate MRT to find the research sequence of this paper
Chat Paper
Summary is being generated by the instructions you defined