Land-Use Regression Modeling to Estimate NO2 and VOC Concentrations in Pohang City, South Korea
ATMOSPHERE(2022)
Abstract
Land-use regression (LUR) has emerged as a promising technique for air pollution modeling to obtain the spatial distribution of air pollutants for epidemiological studies. LUR uses traffic, geographic, and monitoring data to develop regression models and then predict the concentration of air pollutants in the same area. To identify the spatial distribution of nitrogen dioxide (NO2), benzene, toluene, and m-p-xylene, we developed LUR models in Pohang City, one of the largest industrialized areas in Korea. Passive samplings were conducted during two 2-week integrated sampling periods in September 2010 and March 2011, at 50 sampling locations. For LUR model development, predictor variables were calculated based on land use, road lengths, point sources, satellite remote sensing, and population density. The averaged mean concentrations of NO2, benzene, toluene, and m-p-xylene were 28.4 mu g/m(3), 2.40 mu g/m(3), 15.36 mu g/m(3), and 0.21 mu g/m(3), respectively. In terms of model-based R-2 values, the model for NO2 included four independent variables, showing R-2 = 0.65. While the benzene and m-p-xylene models showed the same R-2 values (0.43), toluene showed a lower R-2 value (0.35). We estimated long-term concentrations of NO2 and VOCs at 167,057 addresses in Pohang. Our study could hold particular promise in an epidemiological setting having significant health effects associated with small area variations and encourage the extended study using LUR modeling in Asia.
MoreTranslated text
Key words
land-use regression (LUR), air pollution, nitrogen dioxide (NO2), volatile organic compounds (VOCs), geological information system (GIS), BTX (benzene, toluene and xylene)
AI Read Science
Must-Reading Tree
Example
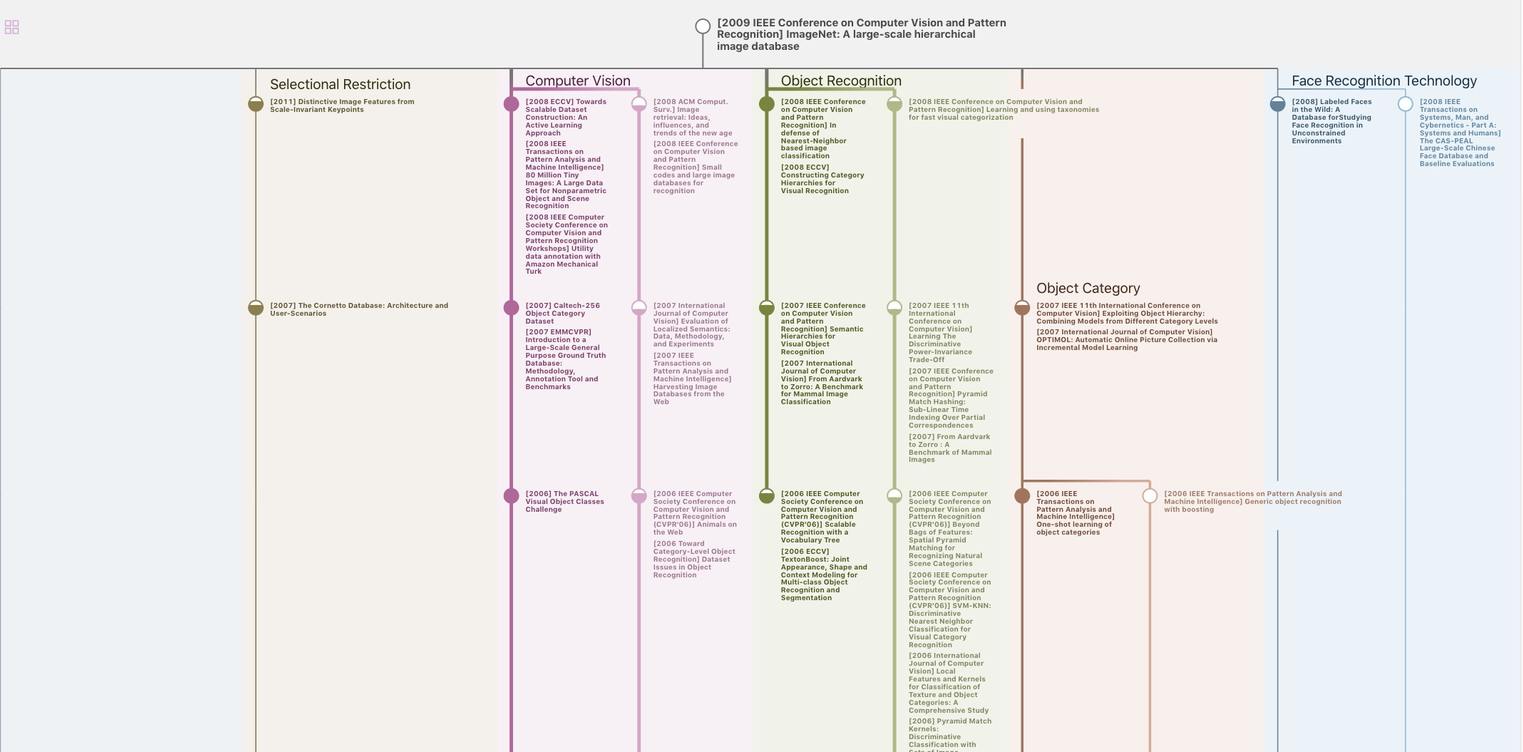
Generate MRT to find the research sequence of this paper
Chat Paper
Summary is being generated by the instructions you defined