Significance of Meteorological Feature Selection and Seasonal Variation on Performance and Calibration of a Low-Cost Particle Sensor
ATMOSPHERE(2022)
摘要
Poor air quality is a major environmental concern worldwide, but people living in low- and middle-income countries are disproportionately affected. Measurement of PM2.5 is essential for establishing regulatory standards and developing policy frameworks. Low-cost sensors (LCS) can construct a high spatiotemporal resolution PM2.5 network, but the calibration dependencies and subject to biases of LCS due to variable meteorological parameters limit their deployment for air-quality measurements. This study used data collected from June 2019 to April 2021 from a PurpleAir Monitor and Met One Instruments' Model BAM 1020 as a reference instrument at Alberta, Canada. The objective of this study is to identify the relevant meteorological parameters for each season that significantly affect the performance of LCS. The meteorological features considered are relative humidity (RH), temperature (T), wind speed (WS) and wind direction (WD). This study applied Multiple Linear Regression (MLR), k-Nearest Neighbor (kNN), Random Forest (RF) and Gradient Boosting (GB) models with varying features in a stepwise manner across all the seasons, and only the best results are presented in this study. Improvement in the performance of calibration models is observed by incorporating different features for different seasons. The best performance is achieved when RF is applied but with different features for different seasons. The significant meteorological features are PM2.5_LCS in Summer, PM2.5_LCS, RH and T in Autumn, PM2.5_LCS, T and WS in Winter and PM2.5_LCS, RH, T and WS in Spring. The improvement in R-2 for each season (values in parentheses) is Summer (0.66-0.94), Autumn (0.73-0.96), Winter (0.70-0.95) and Spring (0.70-0.94). This study signifies selecting the right combination of models and features to attain the best results for LCS calibration.
更多查看译文
关键词
low-cost sensor, calibration, machine learning, meteorological features, seasonal analysis
AI 理解论文
溯源树
样例
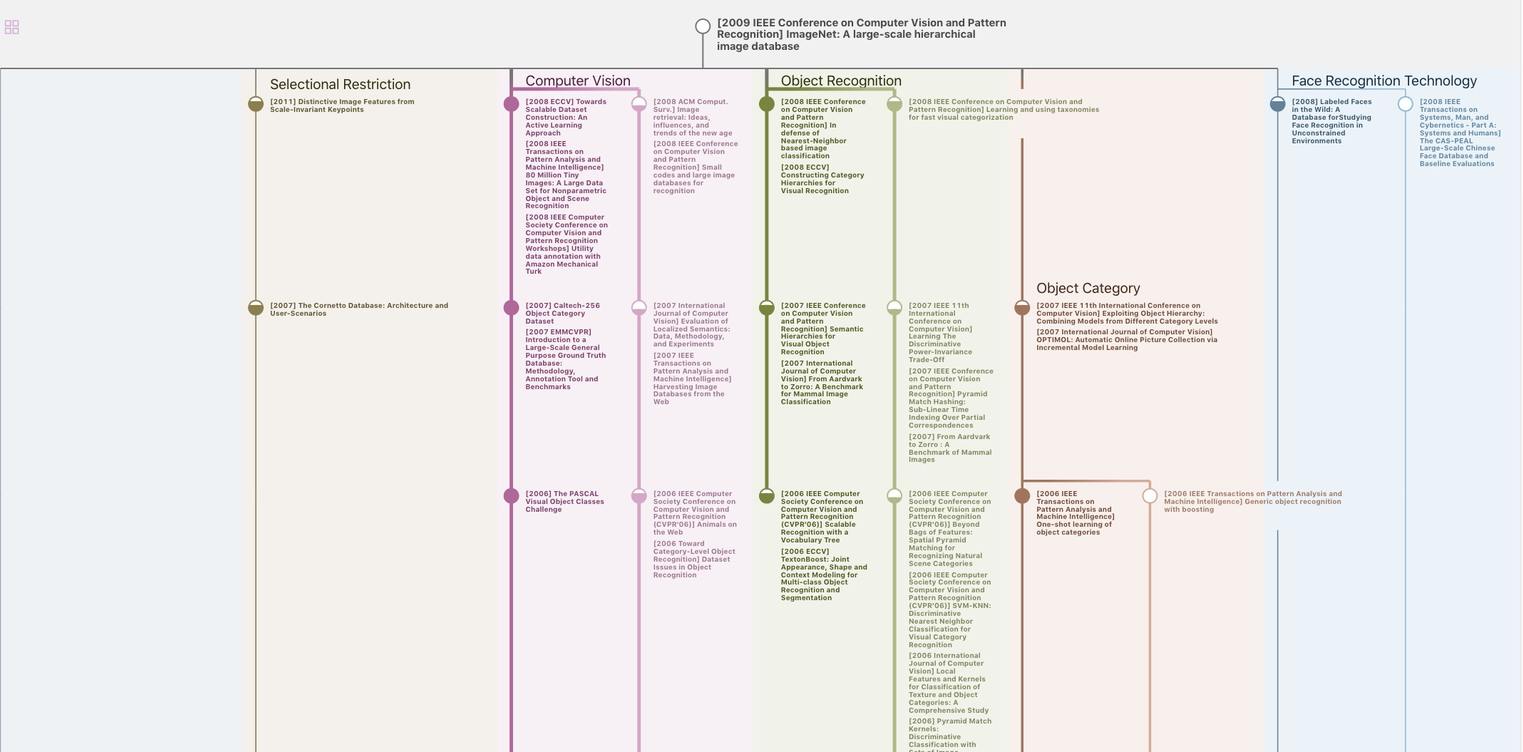
生成溯源树,研究论文发展脉络
Chat Paper
正在生成论文摘要