Automatic pixel-level crack detection and evaluation of concrete structures using deep learning
STRUCTURAL CONTROL & HEALTH MONITORING(2022)
摘要
To achieve effective inspection of reinforced concrete structures, a smart solution to obtain quantitative crack information automatically from inspection is essential. Therefore, a novel crack feature pyramid network (Crack-FPN) based on image analysis is proposed, which has a distinctive feature extraction ability and reduced computational cost. In this method, the network is trained using public annotated pixel-level image datasets. Additionally, beam loading tests and image dataset collection were performed to validate the effectiveness of the proposed method. The crack regions of test images are detected by YOLOv5 using bounding boxes and segmented by Crack-FPN. Compared to existing methods, Crack-FPN shows higher detection accuracy and computational efficiency for crack images affected by illumination conditions and complex backgrounds. In terms of crack width estimation, the relative error of the proposed method in a wide crack width range is approximately 5%, which is considerably small compared with manual measurement results.
更多查看译文
关键词
concrete structures, Crack-FPN, image processing, pixel-level crack detection, segmentation and measurement
AI 理解论文
溯源树
样例
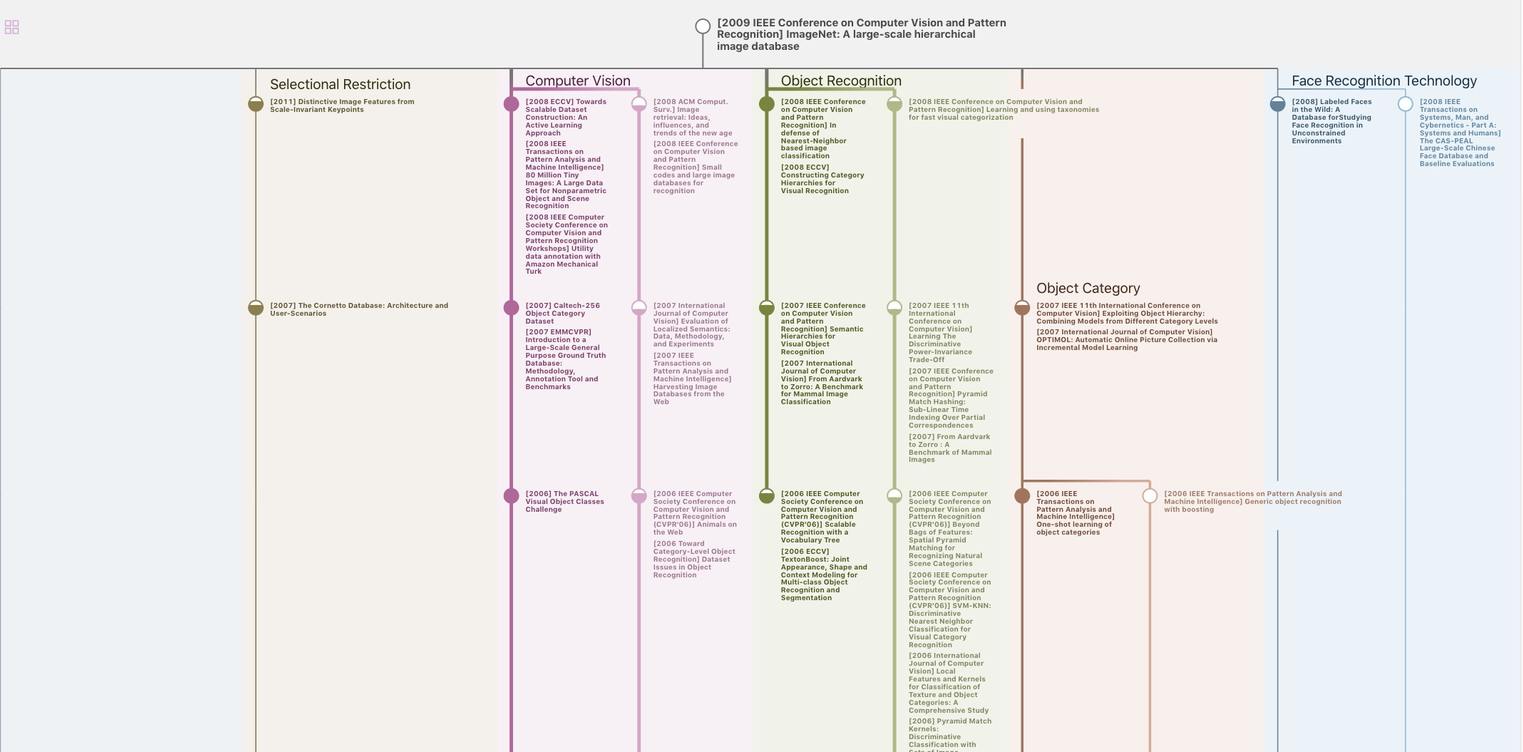
生成溯源树,研究论文发展脉络
Chat Paper
正在生成论文摘要