HDR Tone mapping: System Implementations and Benchmarking
ITE TRANSACTIONS ON MEDIA TECHNOLOGY AND APPLICATIONS(2022)
Abstract
Ever-increasing demand for high quality display has ensued two decades of research in high dynamic range (HDR) imaging, as it has the potential to replace the standard dynamic range imaging. The most significant feature of HDR is the accurate reproducibility of a scene with its entire spectrum of visible lighting and color depth. But this capability comes with expensive capture, display, storage and distribution resource requirements. Additionally, display of HDR video content on a standard display device with limited dynamic range requires some form of adaptation. Many adaptation algorithms, widely known as tone mapping (TM) operators, have been studied and proposed in these two decades. In this paper, we will discuss those TM algorithms which have been implemented on graphics processing unit (GPU)(1-12)), field-programmable gate array (FPGA)(13-47)) , and application specific integrated circuit (ASIC)(48-60)) Like any other image enhancement algorithms tone mapping is resource intensive; accordingly, they have to be accelerated using hardware for real-time performance. This adaptation effort leads to various design challenges that are encountered during the hardware (HW) development. Any real-time application poses strict timing constraints which requires time exact processing of the algorithm. Also, most of the embedded systems would have limited system resources in terms of battery, computational power and memory resources. These design challenges require novel solutions, and in this paper we focus on these HW issues. Recently machine-learning (ML) algorithms have been providing different approaches to solve various image processing problems including tone mapping. Besides HW-TMOs we briefly review learning-based TM algorithms, and discuss important architectures & datasets. Additionally, we present HDR-Net like neural networks as ideal candidate baselines for realizing TMOs with deep neural network (DNN) on hardware/embedded systems.
MoreTranslated text
Key words
Tone mapping, Survey, High Dynamic Range, Image Sensors, ASIC, FPGA, GPU, GAN, U-Net
AI Read Science
Must-Reading Tree
Example
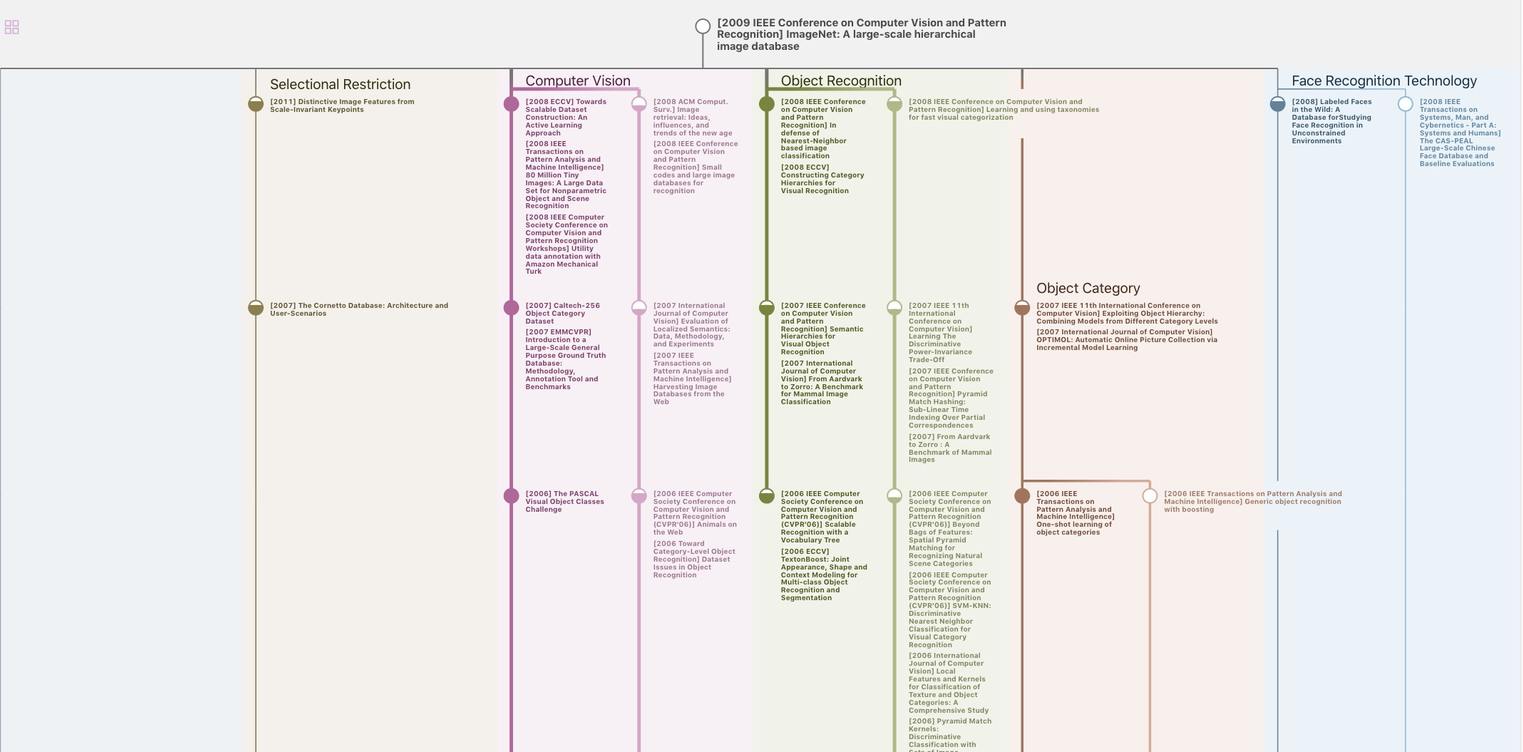
Generate MRT to find the research sequence of this paper
Chat Paper
Summary is being generated by the instructions you defined