Simulating the climate driven runoff in data-scarce mountains by machine learning and downscaling reanalysis data
STOCHASTIC ENVIRONMENTAL RESEARCH AND RISK ASSESSMENT(2022)
Abstract
Lacking climatic data because of scarce meteorological stations make it difficult to assess the non-stationary climatic-runoff processes driven by climate change in the high mountains of northwest China. To address these challenges, this study developed an integrated method by using climate downscaling based on reanalysis products, improved complete ensemble empirical mode decomposition with adaptive noise, and four machine learning algorithms (i.e., radial basis function artificial neural network, random forests, support vector regression, and eXtreme gradient boosting tree) to simulate streamflow. We tested the developed method in seven mountainous basins originating from high Tienshan Mountains, and the observed runoff of hydrological stations indicated the good performance of this method. The Nash–Sutcliffe efficiency coefficient is above 0.7, and mean absolute error and root mean square error between the simulations and observations are below 3 × 10 8 m 3 . This study confirms the importance of multi-scale modeling in improving the accuracy of runoff simulations. On inter-decadal scale, runoff has a more significant non-linear relationship than on inter-annual and seasonal scales. This study also indicates that the runoff changes are mainly controlled by temperature in high mountains, while precipitation contributes more to runoff changes in lowlands. This research can serve the hydrological forecasting and water resources management in data-scarce high mountains.
MoreTranslated text
Key words
Data-scarce mountainous basins, Runoff simulation, Machine learning, Multi-scale modeling
AI Read Science
Must-Reading Tree
Example
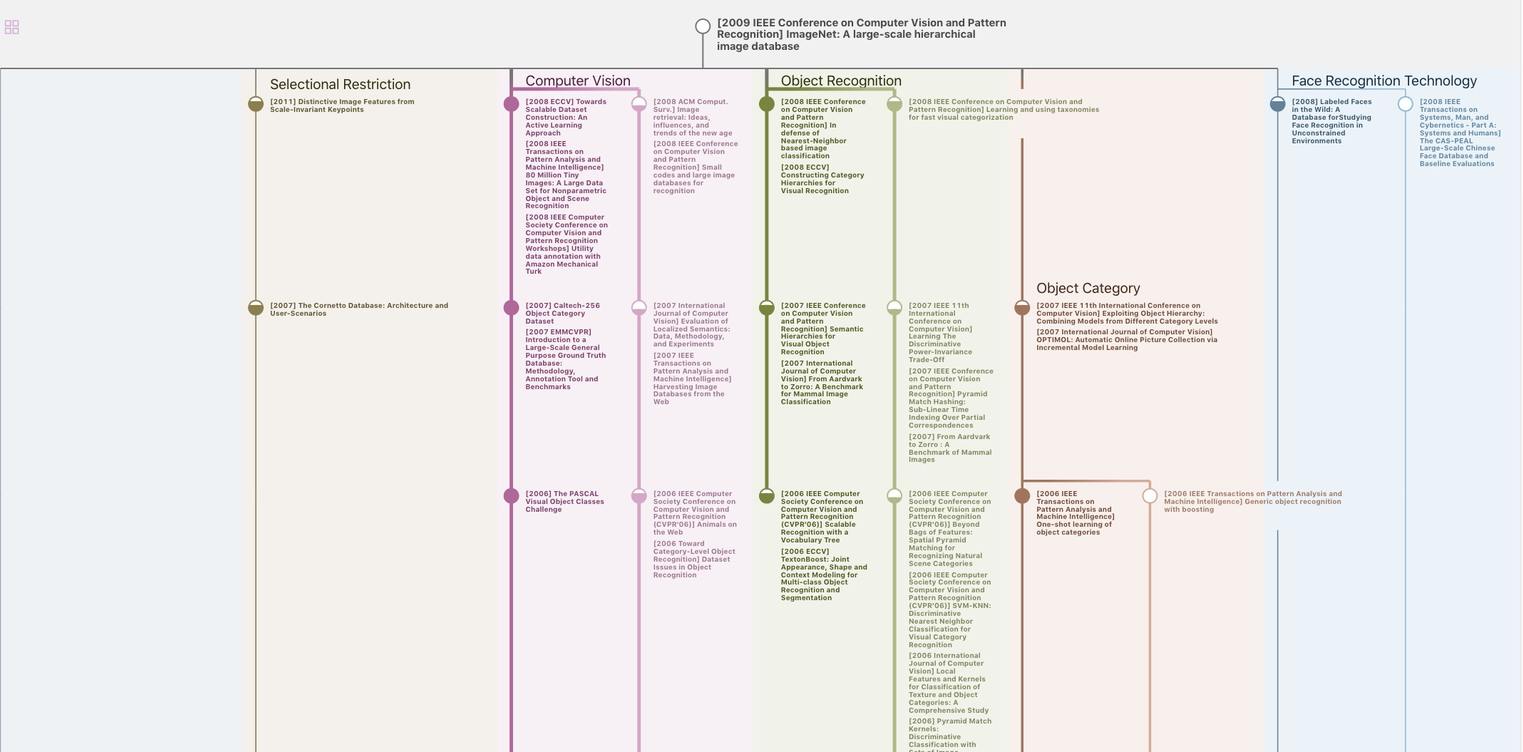
Generate MRT to find the research sequence of this paper
Chat Paper
Summary is being generated by the instructions you defined