Deep Learning-Based Detection of Seedling Development from Indoor to Outdoor
SYSTEMS, SIGNALS AND IMAGE PROCESSING, IWSSIP 2021(2022)
摘要
Monitoring plant growth with computer vision is an important topic in plant science. This monitoring can be challenging when plants are located in outdoor conditions due to light variations and other noises. On other hand, there is a lack of annotated datasets available for such outdoor environments to train machine learning algorithms while indoor similar datasets may be more easily available. In this communication, we investigate, for the first time to the best of our knowledge in plant imaging, how to take benefit from model trained in fully controlled environment to build model for an outdoor environment. This is illustrated with a use case recently published for indoor conditions that we revisit and extend. We compare various spatial and spatio-temporal neural network architectures including long-short term memory convolutional neural network, time distributed convolutional neural network and transformer. While the spatio-temporal architectures outperform the spatial one in indoor conditions, the temporal information appears to be degraded by the presence of shadows due to the variation of light in outdoor conditions. We introduce a specific data augmentation and transfer learning approach which enables to reach a performance of 91% of good classifications with very limited effort of annotation.
更多查看译文
关键词
Plant phenotyping, Deep learning, Transfer learning, Data augmentation, CNN-LSTM, Time distributed deep learning, Transformers
AI 理解论文
溯源树
样例
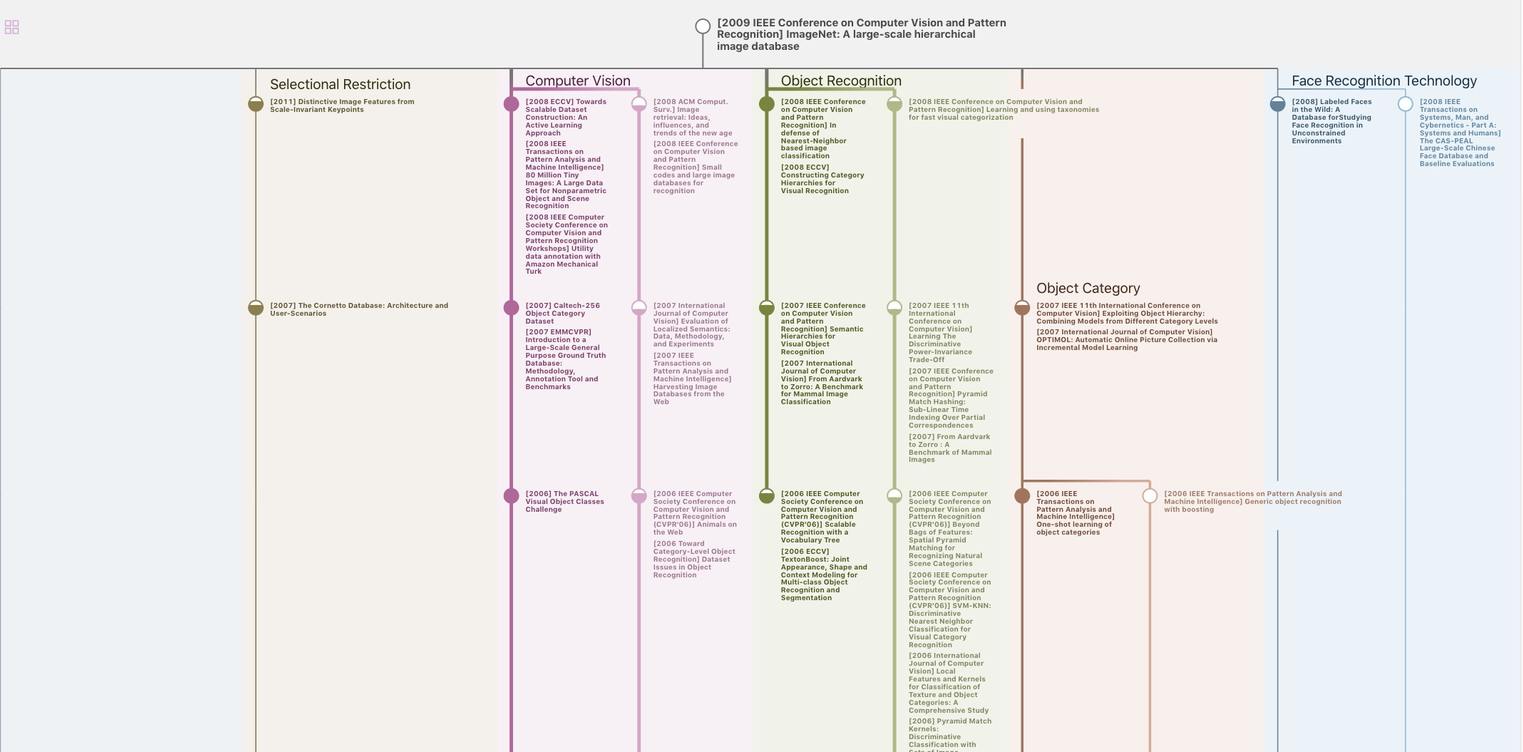
生成溯源树,研究论文发展脉络
Chat Paper
正在生成论文摘要