Improved DCNN Based on Multi-Source Signals for Motor Compound Fault Diagnosis
MACHINES(2022)
Abstract
Induction motors, the key equipment for rotating machinery, are prone to compound faults, such as a broken rotor bars and bearing defects. It is difficult to extract fault features and identify faults from a single signal because multiple fault features overlap and interfere with each other in a compound fault. Since current signals and vibration signals have different sensitivities to broken rotor and bearing faults, a multi-channel deep convolutional neural network (MC-DCNN) fault diagnosis model based on multi-source signals is proposed in this paper, which integrates the original signals of vibration and current of the motor. Dynamic attenuation learning rate and SELU activation function were used to improve the network hyperparameters of MC-DCNN. The dynamic attenuated learning rate can improve the stability of model training and avoid model collapse effectively. The SELU activation function can avoid the problems of gradient disappearance and gradient explosion during model iteration due to its function configuration, thereby avoiding the model falling into local optima. Experiments showed that the proposed model can effectively solve the problem of motor compound fault identification, and three comparative experiments verified that the improved method can improve the stability of model training and the accuracy of fault identification.
MoreTranslated text
Key words
fault diagnosis,compound faults,information fusion,learning rate decay,convolutional neural network
AI Read Science
Must-Reading Tree
Example
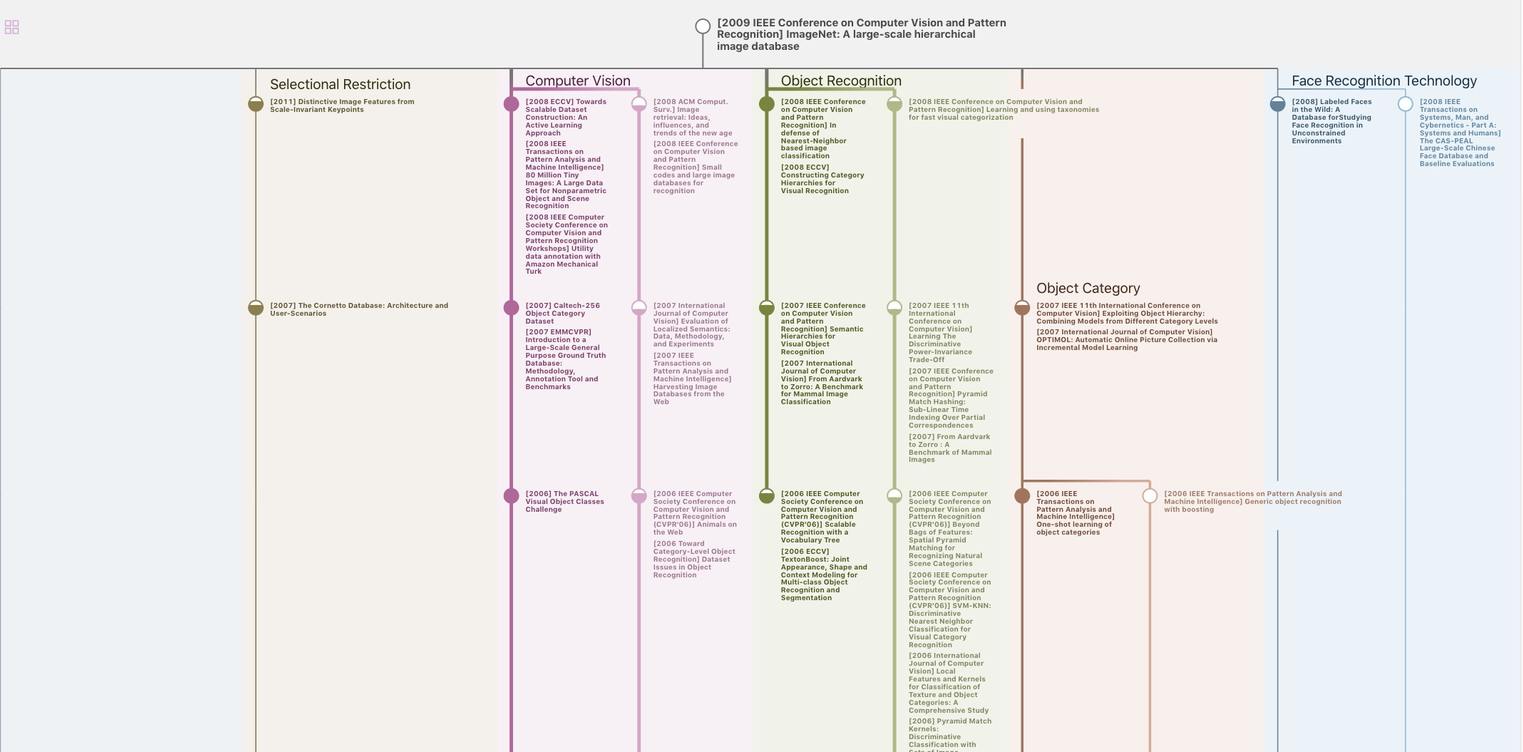
Generate MRT to find the research sequence of this paper
Chat Paper
Summary is being generated by the instructions you defined