Antenna Design Using a GAN-Based Synthetic Data Generation Approach
IEEE Open Journal of Antennas and Propagation(2022)
摘要
In this paper, we propose the use of GANs as learned, data-driven knowledge database that can be queried for rapid synthesis of suitable antenna designs given a desired response. As an example, we consider the problem of designing the Log-Periodic Folded Dipole Array (LPFDA) antenna for two non-overlapping ranges of Q-factor values. By representing the antenna with the vector of its structural parameters and considering each desirable range of the Q-factor as a class, we transform our problem to that of generating new samples from a given class. We develop two alternative models, a Conditional Wasserstein GAN and a label-switched library of vanilla Wasserstein GANs and train them with a dataset of features and their associated labels (parameter vectors and Q-factor range). The main component of these models is a generator network that learns to map a normally distributed noise vector along with a binary label to the vector of parameters of candidate structures. We demonstrate that in inference mode, these models can be relied upon for fast generation of suitable designs.
更多查看译文
关键词
Artificial neural networks,electromagnetics,machine learning
AI 理解论文
溯源树
样例
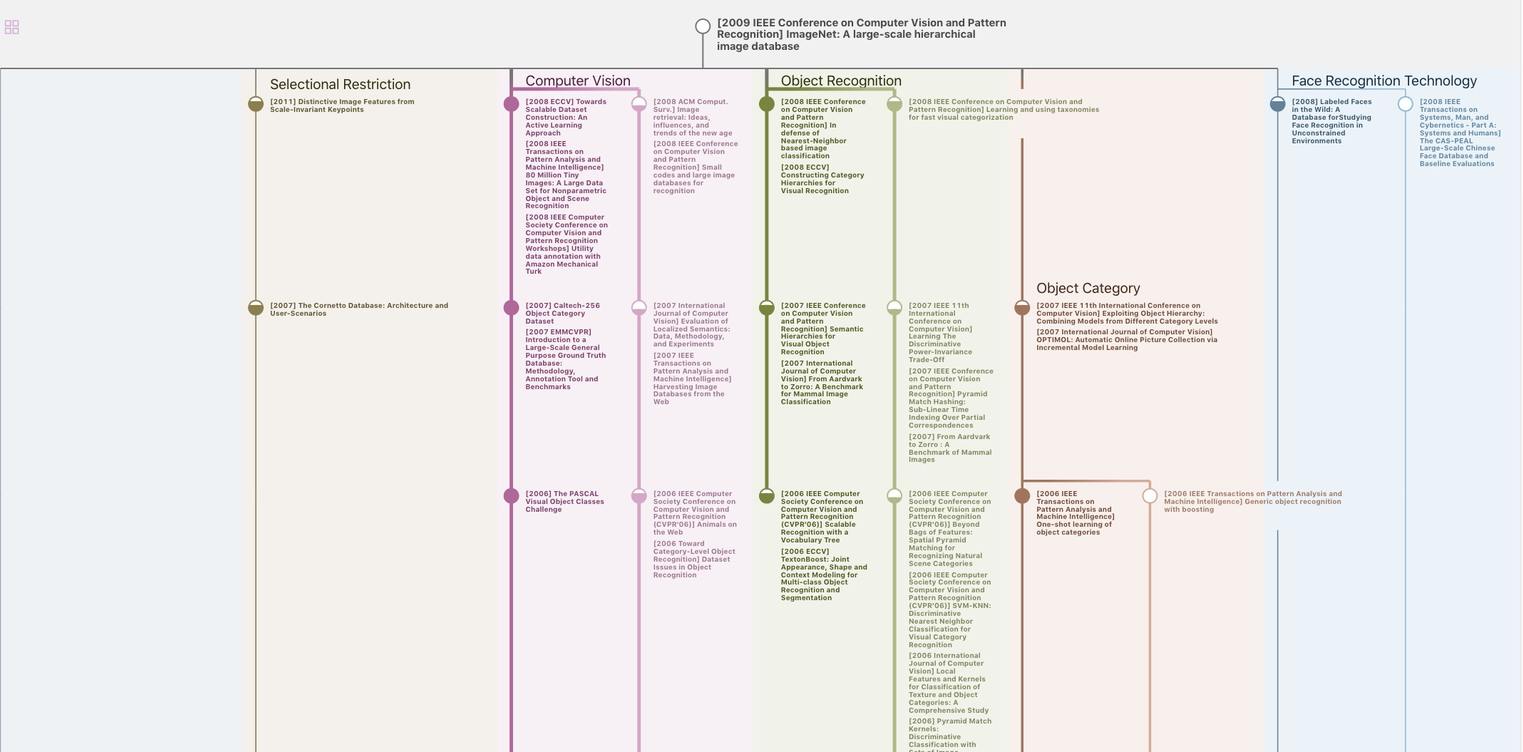
生成溯源树,研究论文发展脉络
Chat Paper
正在生成论文摘要