Automatic modal parameters identification and uncertainty quantification based on block-bootstrap and multi-stage clustering under ambient excitation
JOURNAL OF LOW FREQUENCY NOISE VIBRATION AND ACTIVE CONTROL(2022)
摘要
This study proposes an algorithm for autonomous modal estimation to automatically eliminate false modes and quantify the uncertainty caused by the clustering algorithm and ambient factors. This algorithm belongs to the stochastic subspace identification (SSI) techniques and is based on the Block-Bootstrap and multi-stage clustering analysis. First, the Block-Bootstrap is introduced to decompose the response signal of the structure into M blocks of data. The covariance-driven stochastic subspace identification (SSI-Cov) method is used to process a random sample of data and obtain the corresponding M stabilization diagrams. In addition, the hierarchical clustering method is adopted to carry out the secondary clustering of the picked stable axis according to the defined distance threshold. Then, false modes are eliminated according to the proposed true and false modal discrimination index (MDI). Finally, the above steps are repeated B times, and MDI is used to modify the initial modal parameters of group B. The mean value of elements in the cluster is taken as the recognition result of modal parameters, and the standard deviation is used to measure the accuracy of the recognition result. The numerical simulation results and the modal parameter identification of the Jing-yuan Yellow River Bridge show that, for identifying true and false modals, the proposed modal discrimination index is more effective than the threshold value of the traditional index. Also, it was found that the proposed method can eliminate the uncertainty introduced in the clustering process. In addition, this method can remove the influence of ambient noises, and it can improve the identification accuracy. It will be shown that this method has better anti-noise performance.
更多查看译文
关键词
Automatic modal parameters identification, stochastic subspace identification, uncertainty quantification, block-bootstrap, clustering, stabilization diagram
AI 理解论文
溯源树
样例
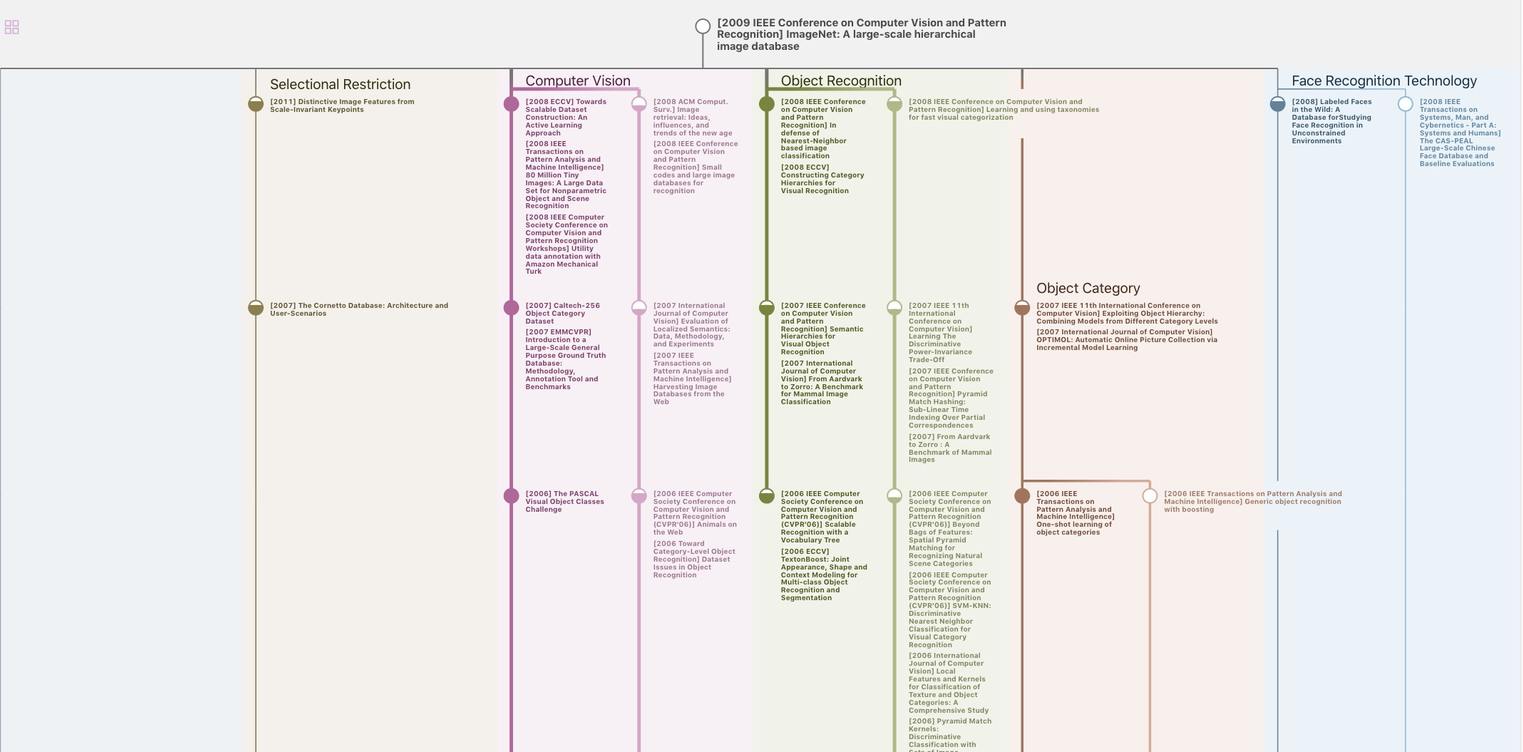
生成溯源树,研究论文发展脉络
Chat Paper
正在生成论文摘要