A Hierarchical Probabilistic Divergent Search Applied to a Binary Classification
ICAART: PROCEEDINGS OF THE 14TH INTERNATIONAL CONFERENCE ON AGENTS AND ARTIFICIAL INTELLIGENCE - VOL 2(2022)
Abstract
The trend in recent years of the scientific community on solving a wide range of problems through Artificial Intelligence has highlighted the benefits of open-ended search algorithms. In this paper we apply a probabilistic version for a divergent search algorithm in combination of a strategy to reduce the number of evaluations and computational effort by gathering the population from a Genetic Programming algorithm into groups and pruning the worst groups each certain number of generations. The combination proposed has shown encouraging results against a standard GP implementation on three binary classification problems, where the time taken to run an experiment is significantly reduced to only 5% of the total time from the standard approach while still maintaining, and indeed exceeding in the experimental results.
MoreTranslated text
Key words
Genetic Programming, Novelty Search, Classification
AI Read Science
Must-Reading Tree
Example
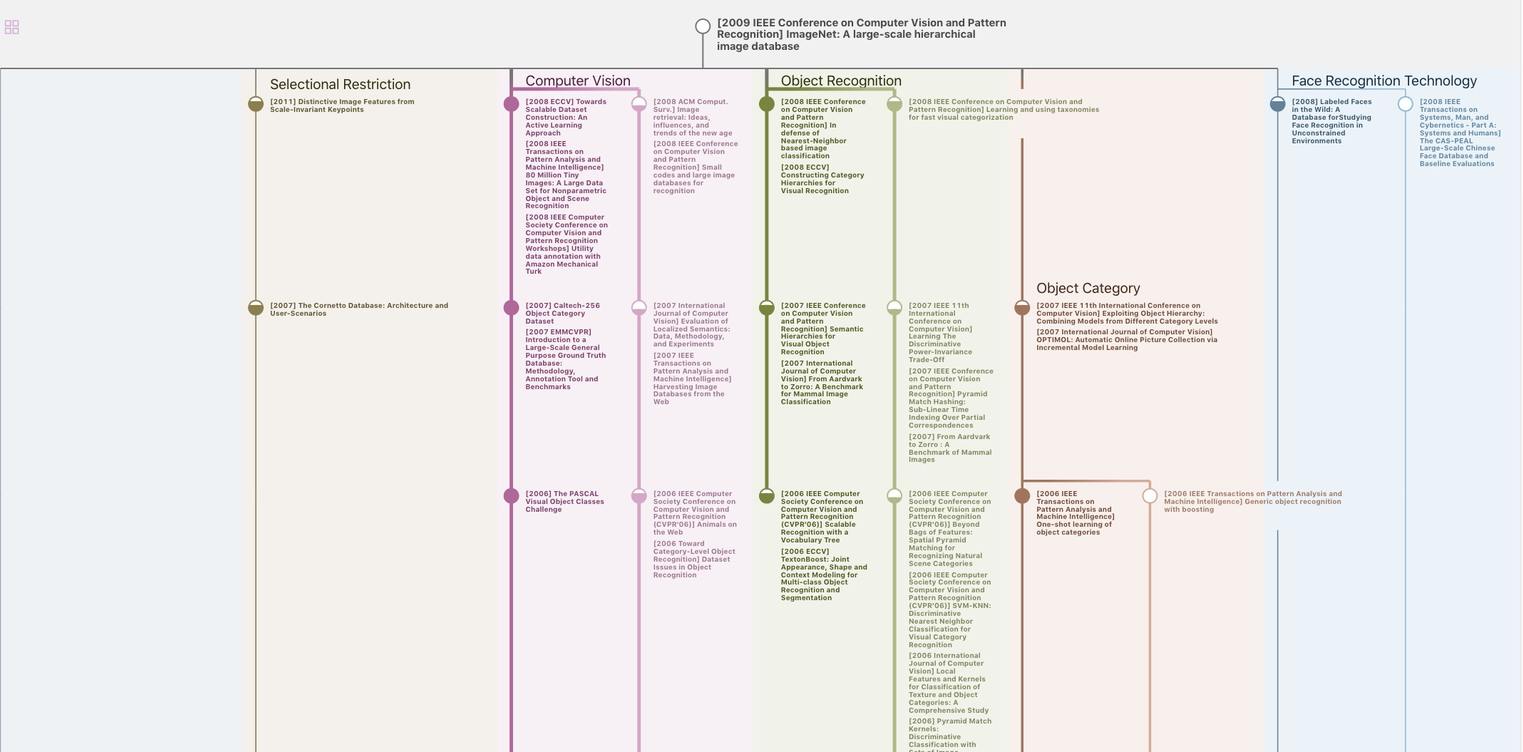
Generate MRT to find the research sequence of this paper
Chat Paper
Summary is being generated by the instructions you defined