Bilinear Multi-Head Attention Graph Neural Network for Traffic Prediction
ICAART: PROCEEDINGS OF THE 14TH INTERNATIONAL CONFERENCE ON AGENTS AND ARTIFICIAL INTELLIGENCE - VOL 2(2022)
摘要
Traffic forecasting is an important component of Intelligent Transportation System (ITS) and it has the significance for reducing traffic accidents and improving public safety. Due to the complex spatial-temporal dependencies and the uncertainty of road network, the research on this problem is quite challenging. Some of the latest studies utilize graph convolutional networks (GCNs) to model spatial-temporal relationships. However, these methods are only based on the linear weighted summation of the neighborhood to form the representation of the target node, which cannot capture the signal between pairwise node interactions. In many scenes, adding pairwise node interaction features is an essential way to better represent the target node. Therefore, in this article, we propose an end-to-end novel framework named Bilinear Multi-Head Attention Graph Neural Network (BMHA-GNN) for traffic prediction. We propose a new aggregation operator which utilizes the weighted sum of pairwise interactions of the neighbour nodes and improves the representation ability of GCN based models. We adopt the encoder-decoder framework, the encoder module outputs the representation of traffic data, and the decoder module outputs the prediction results. The multi-head attention mechanism is introduced to aggregate information of different neighbour nodes automatically and stabilize the training process. Extensive experiments are conducted on two real-world datasets (METR-LA, PEMS-BAY) showing that the proposed model BMHA-GNN achieves the state-of-the-art results.
更多查看译文
关键词
Bilinear Aggregator, Graph Neural Networks, Traffic Forecasting, Multi Attention
AI 理解论文
溯源树
样例
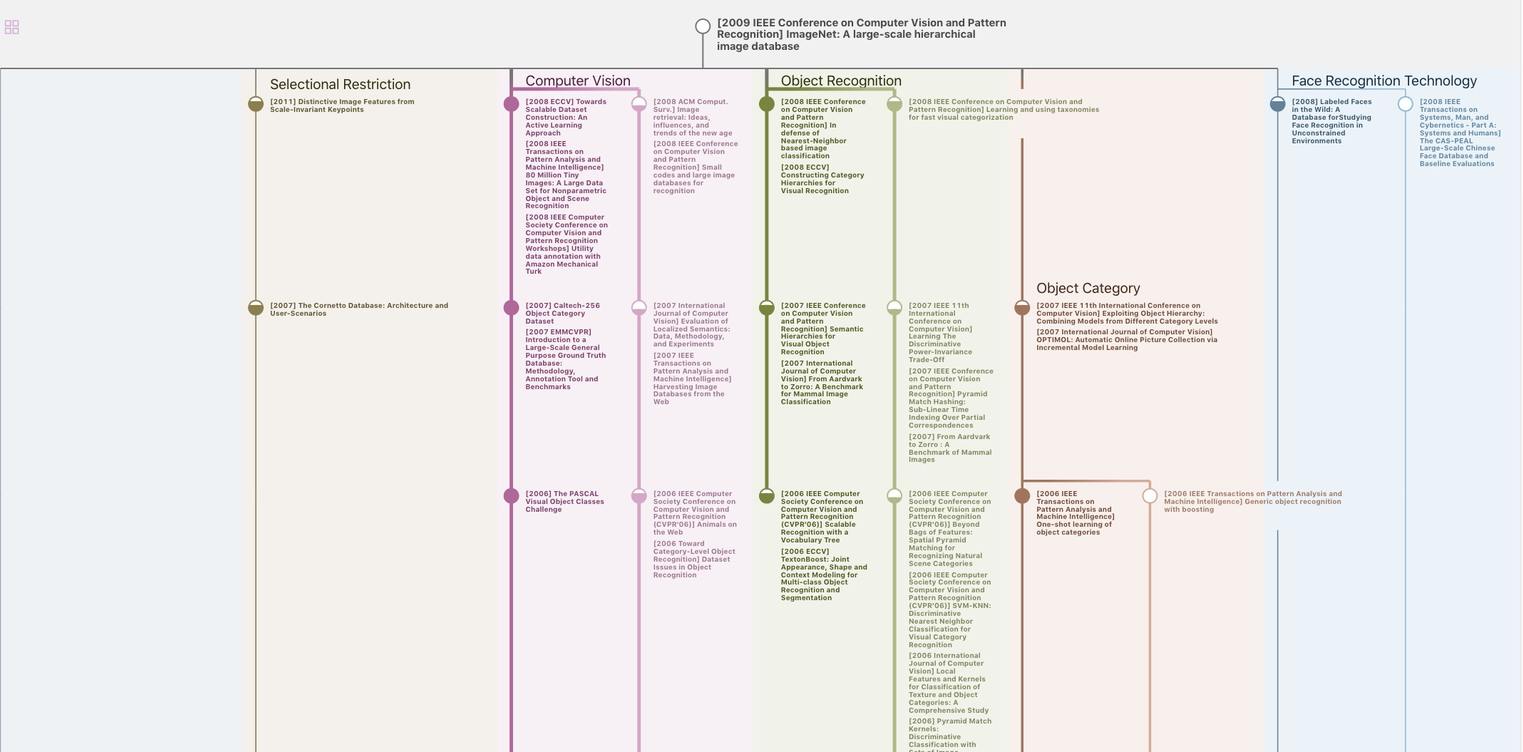
生成溯源树,研究论文发展脉络
Chat Paper
正在生成论文摘要