Method for Improving Quality of Adversarial Examples
ICAART: PROCEEDINGS OF THE 14TH INTERNATIONAL CONFERENCE ON AGENTS AND ARTIFICIAL INTELLIGENCE - VOL 2(2022)
摘要
To evaluate the robustness of DNNs, most of the adversarial methods such as FGSM, box-constrained L-BFGS, and ATN generate adversarial examples with small L-p-norm. However, these adversarial examples might contain many redundant perturbations. Removing these perturbations increases the quality of adversarial examples. Therefore, this paper proposes a method to improve the quality of adversarial examples by recognizing and then removing such perturbations. The proposed method includes two phases namely the autoencoder training phase and the improvement phase. In the autoencoder training phase, the proposed method trains an autoencoder that learns how to recognize redundant perturbations. In the second phase, the proposed method uses the trained autoencoder in combination with the greedy improvement step to produce more high-quality adversarial examples. The experiments on MNIST and CIFAR-10 have shown that the proposed method could improve the quality of adversarial examples significantly. In terms of L-0-norm, the distance decreases by about 82%-95%. In terms of L-2-norm, the distance drops by around 56%-81%. Additionally, the proposed method has a low computational cost. This shows the potential ability of the proposed method in practice.
更多查看译文
关键词
Adversarial Example Generation, Deep Neural Network, Robustness, Autoencoder
AI 理解论文
溯源树
样例
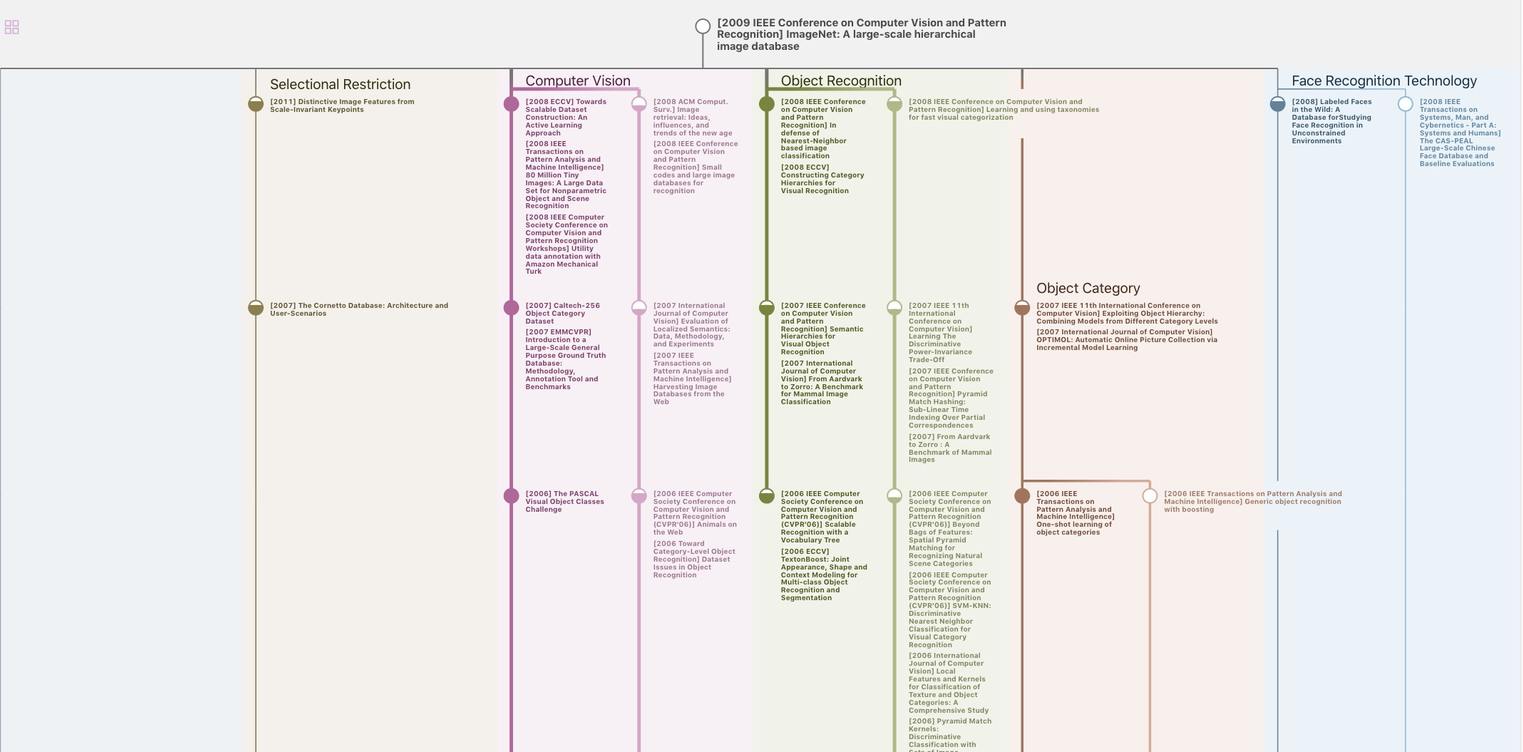
生成溯源树,研究论文发展脉络
Chat Paper
正在生成论文摘要