Leveraging Causal Relations to Provide Counterfactual Explanations and Feasible Recommendations to End Users
ICAART: PROCEEDINGS OF THE 14TH INTERNATIONAL CONFERENCE ON AGENTS AND ARTIFICIAL INTELLIGENCE - VOL 2(2022)
摘要
Over the last years, there has been a growing debate on the ethical issues of Artificial Intelligence (AI). Explainable Artificial Intelligence (XAI) has appeared as a key element to enhance trust of AI systems from both technological and human-understandable perspectives. In this sense, counterfactual explanations are becoming a de facto solution for end users to assist them in acting to achieve a desired outcome. In this paper, we present a new method called Counterfactual Explanations as Interventions in Latent Space (CEILS) to generate explanations focused on the production of feasible user actions. The main features of CEILS are: it takes into account the underlying causal relations by design, and can be set on top of an arbitrary counterfactual explanation generator. We demonstrate how CEILS succeeds through its evaluation on a real dataset of the financial domain.
更多查看译文
关键词
Explainable Artificial Intelligence, Counterfactual Explanations, Causality, Recourse
AI 理解论文
溯源树
样例
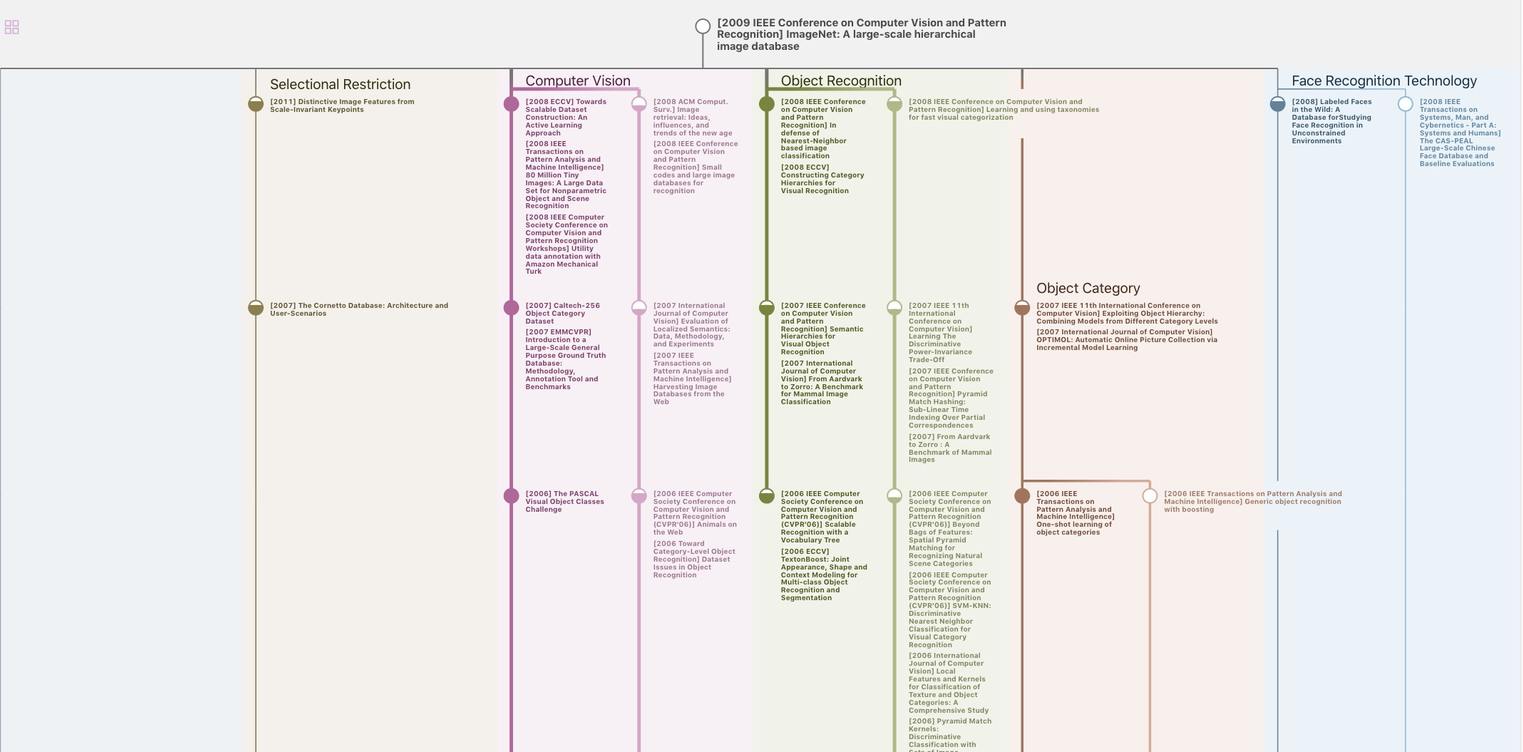
生成溯源树,研究论文发展脉络
Chat Paper
正在生成论文摘要