Joint latent space models for network data with high-dimensional node variables
BIOMETRIKA(2022)
摘要
Network latent space models assume that each node is associated with an unobserved latent position in a Euclidean space, and such latent variables determine the probability of two nodes connecting with each other. In many applications, nodes in the network are often observed along with high-dimensional node variables, and these node variables provide important information for understanding the network structure. However, classical network latent space models have several limitations in incorporating node variables. In this paper, we propose a joint latent space model where we assume that the latent variables not only explain the network structure, but are also informative for the multivariate node variables. We develop a projected gradient descent algorithm that estimates the latent positions using a criterion incorporating both network structure and node variables. We establish theoretical properties of the estimators and provide insights into how incorporating high-dimensional node variables could improve the estimation accuracy of the latent positions. We demonstrate the improvement in latent variable estimation and the improvements in associated downstream tasks, such as missing value imputation for node variables, by simulation studies and an application to a Facebook data example.
更多查看译文
关键词
High-dimensional data, Latent space model, Network analysis
AI 理解论文
溯源树
样例
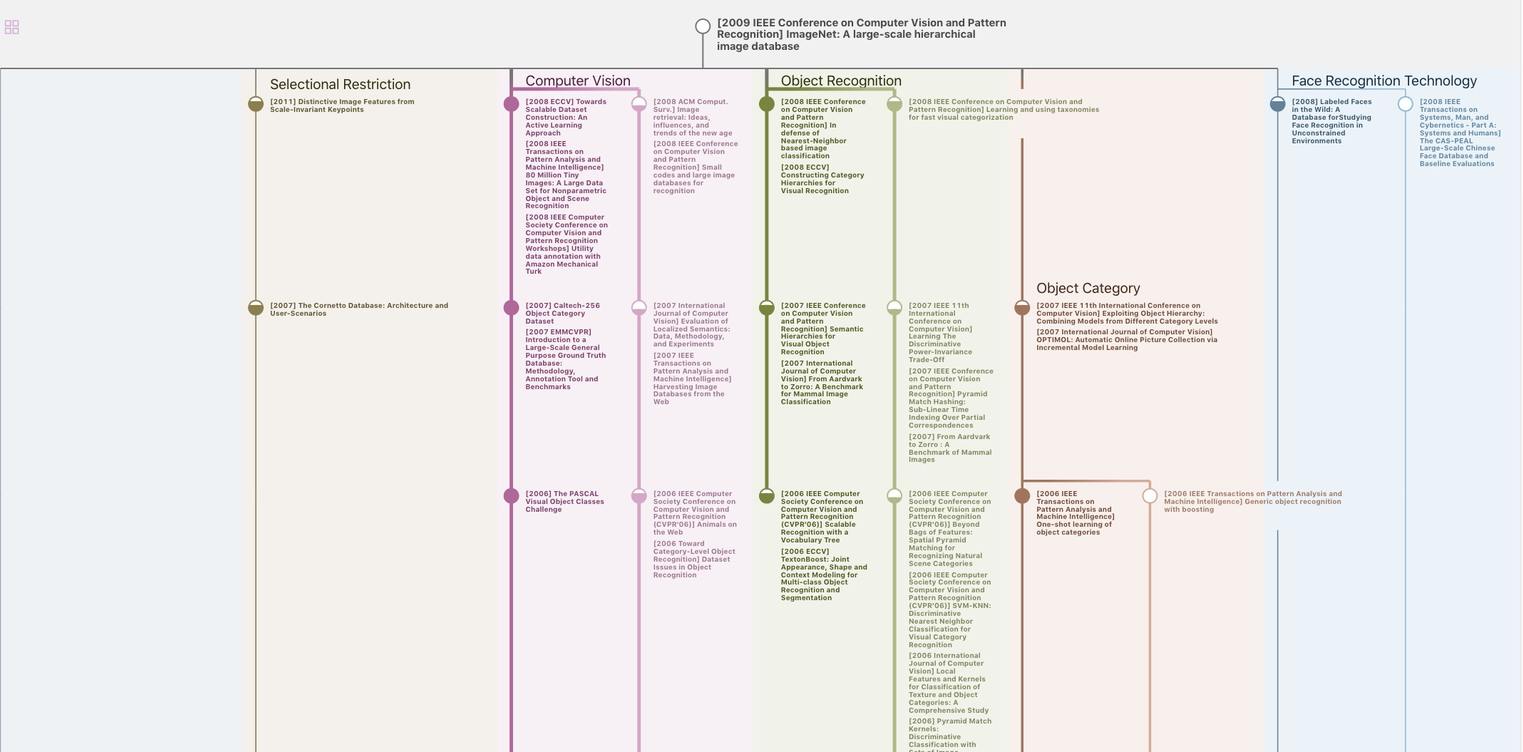
生成溯源树,研究论文发展脉络
Chat Paper
正在生成论文摘要