A control strategy of normal motion and active self-rescue for autonomous underwater vehicle based on deep reinforcement learning
AIP ADVANCES(2022)
Abstract
The survivability of autonomous underwater vehicles (AUV) in complex missions and dangerous situations is of great significance to ocean resource exploration, hydrological research, maritime rescue, and undersea military. Existing researches on motion control for the AUV mainly focus on its normal operating, but the active self-rescue method in emergency situations is hardly found. As classical control methods are not sufficient enough for complicated self-rescue missions of the AUV, this paper uses the deep reinforcement learning (DRL) algorithm to solve this problem because the DRL algorithm has the advantages in learning and decision making for complex robot control missions. In this paper, the normal motion control of the AUV based on the deep deterministic policy gradient algorithm is explored, including the yaw angle adjustment, yaw angle adjustment extension, trajectory tracking, and normal floating-up control of the AUV. Then, active self-rescue methods are successfully achieved to recover the AUV from emergencies, such as ocean water density decreasing sharply or one fin getting jammed at a random angle. What is more, real environment experiments are successfully conducted on a self-developed platform of the AUV to validate the feasibility of the proposed control methods. The results can effectively improve the survivability of the AUV and can be a reference to submarine survivability technologies. (c) 2022 Author(s). All article content, except where otherwise noted, is licensed under a Creative Commons Attribution (CC BY) license (http://creativecommons.org/licenses/by/4.0/).
MoreTranslated text
Key words
Underwater Robotics,Autonomous Underwater Vehicles,Reinforcement Learning,Deep Learning
AI Read Science
Must-Reading Tree
Example
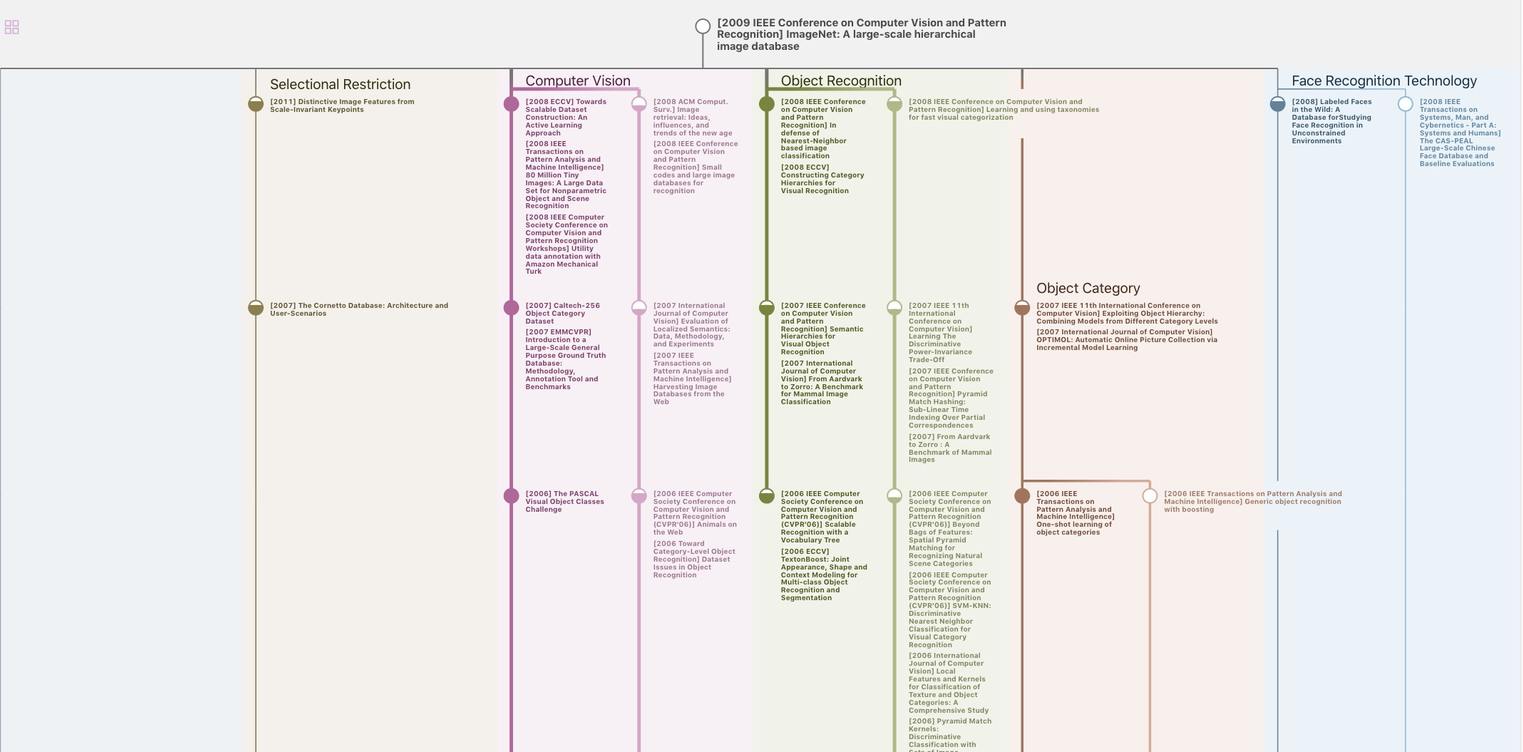
Generate MRT to find the research sequence of this paper
Chat Paper
Summary is being generated by the instructions you defined