sEMG Signals Characterization and Identification of Hand Movements by Machine Learning Considering Sex Differences
APPLIED SCIENCES-BASEL(2022)
摘要
Developing a robust machine-learning algorithm to detect hand motion is one of the most challenging aspects of prosthetic hands and exoskeleton design. Machine-learning methods that considered sex differences were used to identify and describe hand movement patterns in healthy individuals. To this purpose, surface Electromyographic (sEMG) signals have been acquired from muscles in the forearm and hand. The results of statistical analysis indicated that most of the same muscle pairs in the right hand (females and males) showed significant differences during the six hand movements. Time features were used an as input to machine-learning algorithms for the recognition of six gestures. Specifically, two types of hand-gesture recognition methods that considered sex differences(differentiating sex datasets and adding a sex label)were proposed and applied to the k-nearest neighbor (k-NN), support vector machine (SVM) and artificial neural network (ANN) algorithms for comparison. In addition, a t-test statistical analysis approach and 5-fold cross validation were used as complements to verify whether considering sex differences could significantly improve classification performance. It was demonstrated that considering sex differences can significantly improve classification performance. The ANN algorithm with the addition of a sex label performed best in movement classification (98.4% accuracy). In the future, hand movement recognition algorithms considering sex differences could be applied to control systems for prosthetic hands or exoskeletons.
更多查看译文
关键词
hand movements, sex differences, machine learning, artificial neural network, exoskeletons, surface electromyography (sEMG)
AI 理解论文
溯源树
样例
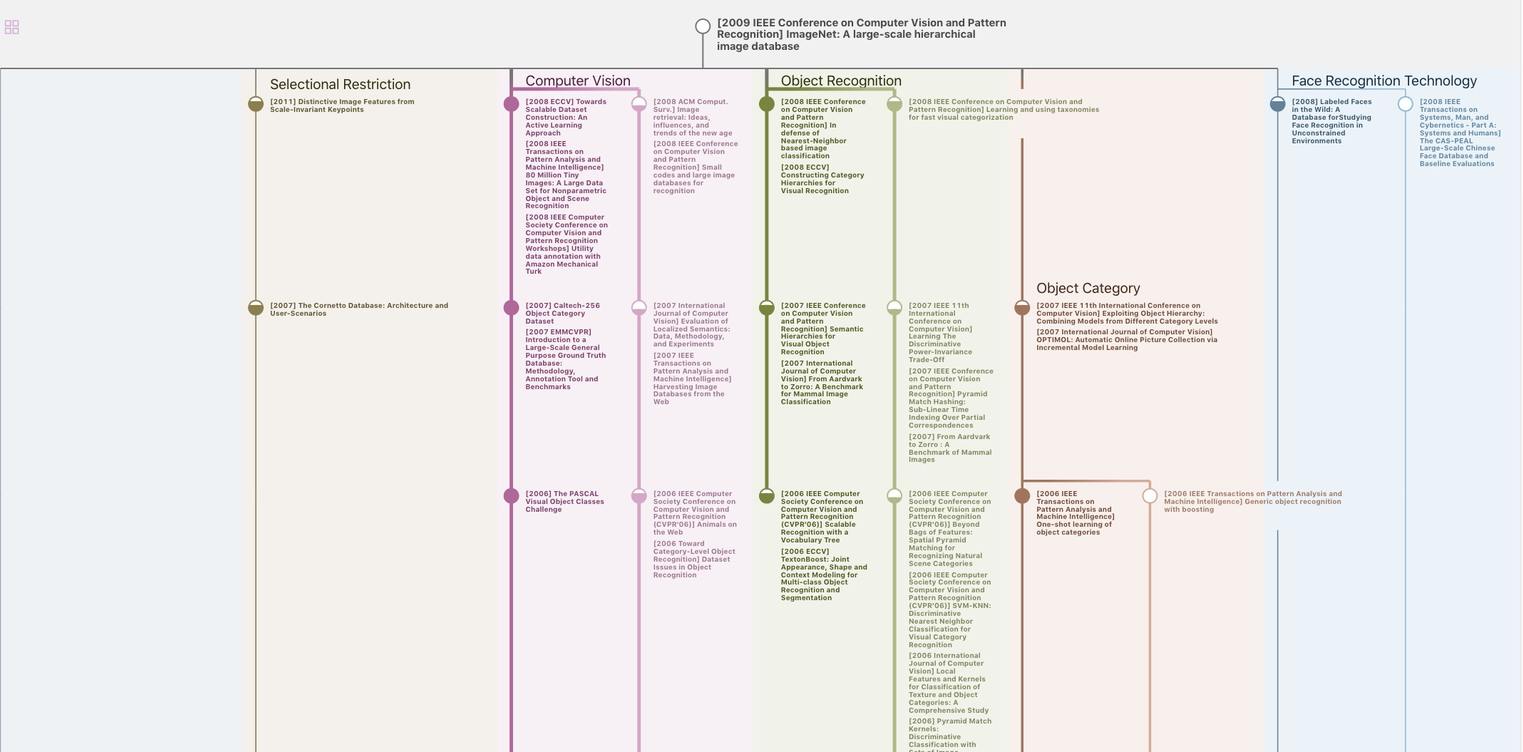
生成溯源树,研究论文发展脉络
Chat Paper
正在生成论文摘要