Learning to Cooperate: Decision Fusion Method for Few-Shot Remote-Sensing Scene Classification
IEEE GEOSCIENCE AND REMOTE SENSING LETTERS(2022)
摘要
Recently, remote-sensing scene classification has become an essential primary research topic. Nowadays, scholars have proposed various few-shot remote-sensing scene classification methods to achieve superior performance with few labeled data. Most of the prior work utilized a meta-learning strategy, which suffered from too little data affecting performance. In this letter, we apply the pre-trained feature extractor for image embedding. Meanwhile, because of the negative transfer problem caused by the inadaptability of the pre-trained feature extractor to remote-sensing data, we propose to exploit two pre-trained models to classify the remote-sensing scene, respectively. Then we fuse the decision to obtain the final classification category. We design a decision attention module to automatically update combination weights for each decision. It comprehensively considers the contribution of various decisions and further improves the discrimination of features. We conduct comprehensive experiments to validate the method and achieve state-of-the-art performance on two benchmark remote-sensing scene datasets, namely NWPU-RESISC45 and UC Merced.
更多查看译文
关键词
Feature extraction, Remote sensing, Image analysis, Task analysis, Data models, Data mining, Training, Decision fusion, few-shot learning, remote-sensing scene classification
AI 理解论文
溯源树
样例
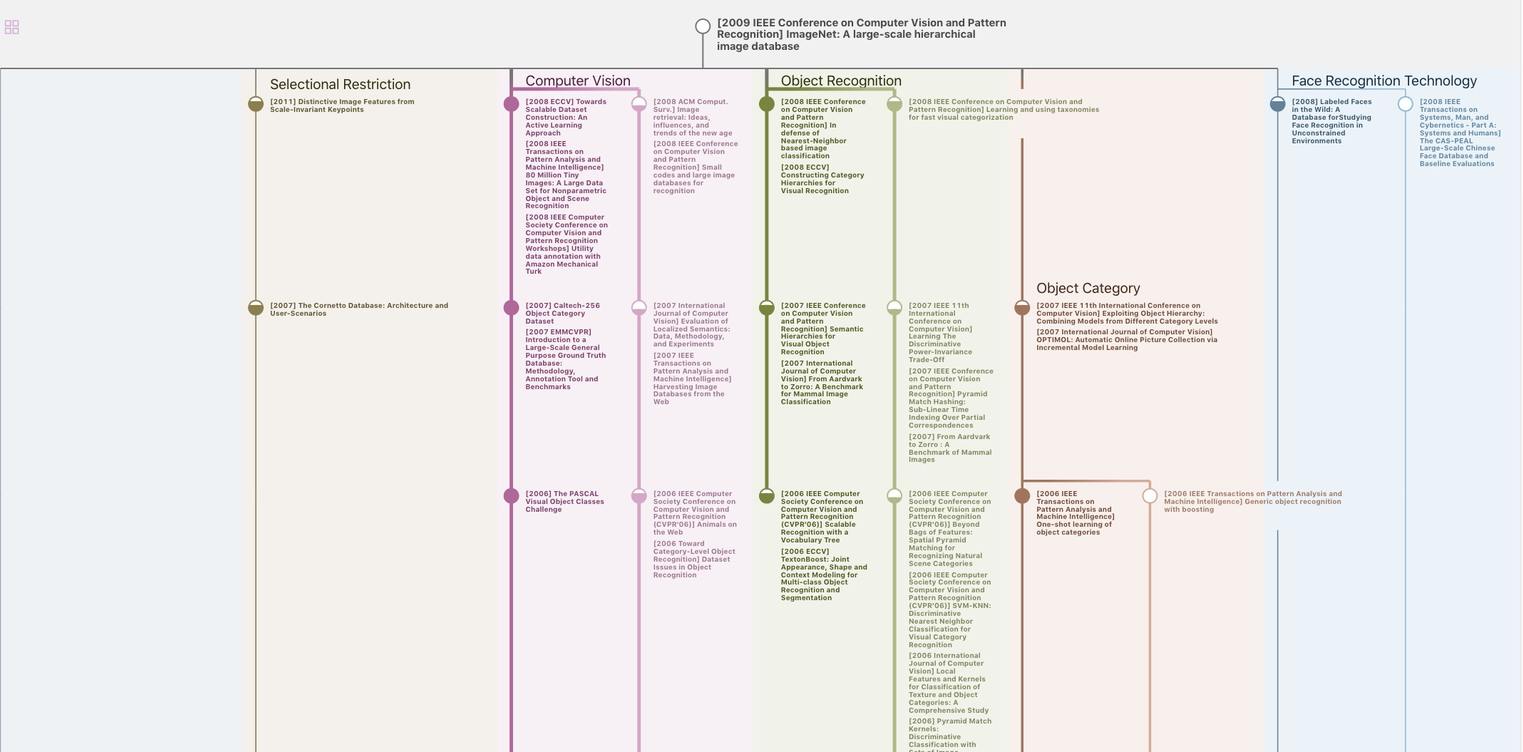
生成溯源树,研究论文发展脉络
Chat Paper
正在生成论文摘要