An Instance-Based Multitask Graph Network for Complex Facility Recognition in Remote Sensing Imagery
IEEE TRANSACTIONS ON GEOSCIENCE AND REMOTE SENSING(2022)
Abstract
With the availability of very high-resolution remote sensing imagery, the fine-grained recognition of complex geospatial facilities has become possible. We can view these facilities as a combination of component objects with specific functions and distribution. However, the existing methods are insufficient in modeling spatial relations of component objects. In this article, we propose an instance-based multitask graph network (IBMG-Net) for complex facility recognition. Specifically, we perform pixel-level component objects prediction and facility recognition simultaneously and achieve performance improvement of both tasks by joint multitasking training. Given the component information, we build an instance-based graph neural network (IBGN) where components are defined as nodes and their spatial relations are encoded as edges. The IBGN module aims to flexibly model spatial relations of complex facility. To enhance the feature representation of component objects, we utilize the multiscale region of interest module (MS-ROI) to retain all scale-specific features and the sparse context information module (SCM) to aggregate long-range context information. In addition, we build a new multitask dataset for complex facility recognition in remote sensing (MCF dataset) to verify the effectiveness of our method and alleviate the lack of pixel-level labeled multitask datasets in remote sensing. Extensive experiments on MCF also indicate that the significant performance improvement of our approach to complex facility recognition.
MoreTranslated text
Key words
Semantics, Feature extraction, Remote sensing, Task analysis, Image segmentation, Image recognition, Data mining, Complex facility, component information, contextual information, graph neural network (GNN), instance segmentation, remote sensing imagery, scene recognition
AI Read Science
Must-Reading Tree
Example
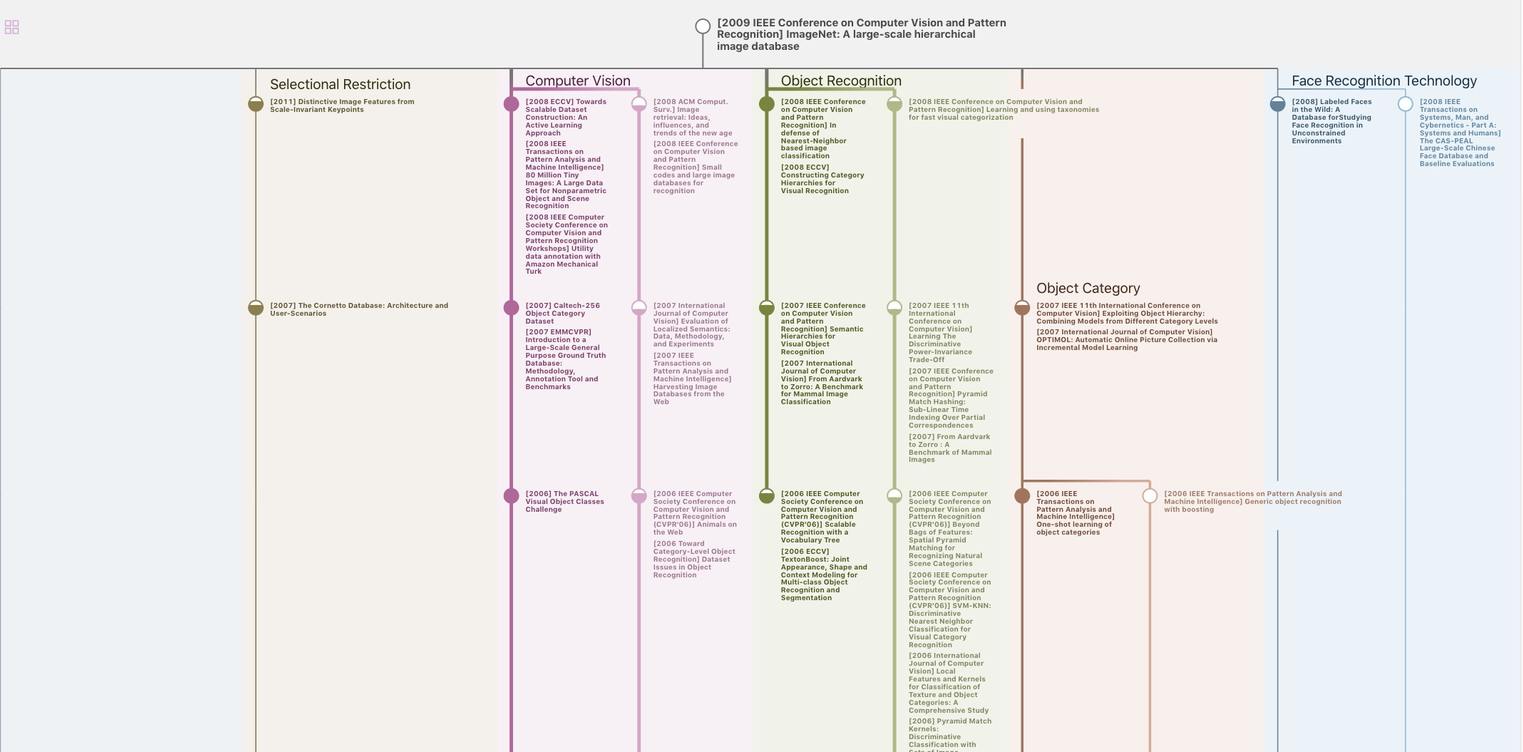
Generate MRT to find the research sequence of this paper
Chat Paper
Summary is being generated by the instructions you defined