BOOTSTRAP INFERENCE FOR MULTIPLE CHANGE-POINTS IN TIME SERIES
ECONOMETRIC THEORY(2022)
摘要
In this paper, we propose two bootstrap procedures, namely parametric and block bootstrap, to approximate the finite sample distribution of change-point estimators for piecewise stationary time series. The bootstrap procedures are then used to develop a generalized likelihood ratio scan method (GLRSM) for multiple change-point inference in piecewise stationary time series, which estimates the number and locations of change-points and provides a confidence interval for each change-point. The computational complexity of using GLRSM for multiple change-point detection is as low as O(n(log n)(3)) for a series of length n. Extensive simulation studies are provided to demonstrate the effectiveness of the proposed methodology under different scenarios. Applications to financial time series are also illustrated.
更多查看译文
AI 理解论文
溯源树
样例
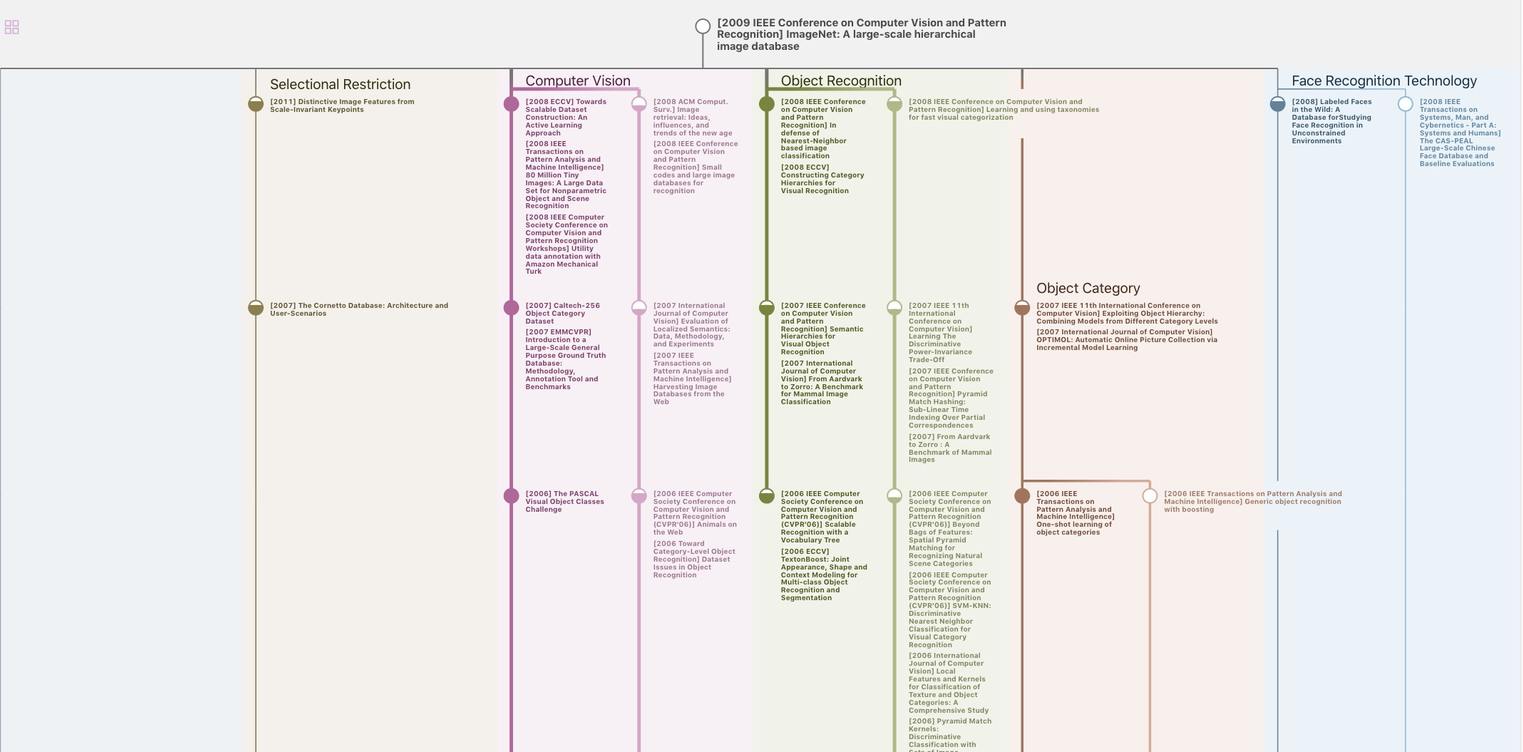
生成溯源树,研究论文发展脉络
Chat Paper
正在生成论文摘要