Measuring Prediction Accuracy in a Maritime Accident Warning System
PRODUCTION AND OPERATIONS MANAGEMENT(2022)
摘要
Advances in machine learning methods and the availability of new data sources show promise for improving prediction of operational risk. Maritime transportation is the backbone of global supply chains and maritime accidents can lead to costly disruptions. We describe a case study performed for the United States Coast Guard (USCG) to develop a prototype risk prediction system to provide early alerts of elevated risk levels to vessel traffic managers and operators in the Lower Mississippi River, the second largest port of entry in the United States. Integrating incident and accident data from the USCG with environmental and traffic data sources, we tested existing machine learning algorithms in their predictive ability. We found poor accident prediction accuracy in cross-validation using the traditional measures of precision and sensitivity. In this specific operational context, however, such single-class accuracy metrics can be misleading. We define action precision and action sensitivity metrics that measure the accuracy of predictions in engendering the correct behavioral response (actions) among vessel operators, rather than getting the specific event classification correct. We use these operationally appropriate measures for maritime risk prediction to choose an algorithm for our prototype system. While the traditional metrics indicated that none of the algorithms would perform sufficiently well to use in the early warning system, the modified metrics show that the top performing algorithm will perform well in this operational context.
更多查看译文
关键词
predictive analytics, operational risk, maritime transportation, supply chain disruption
AI 理解论文
溯源树
样例
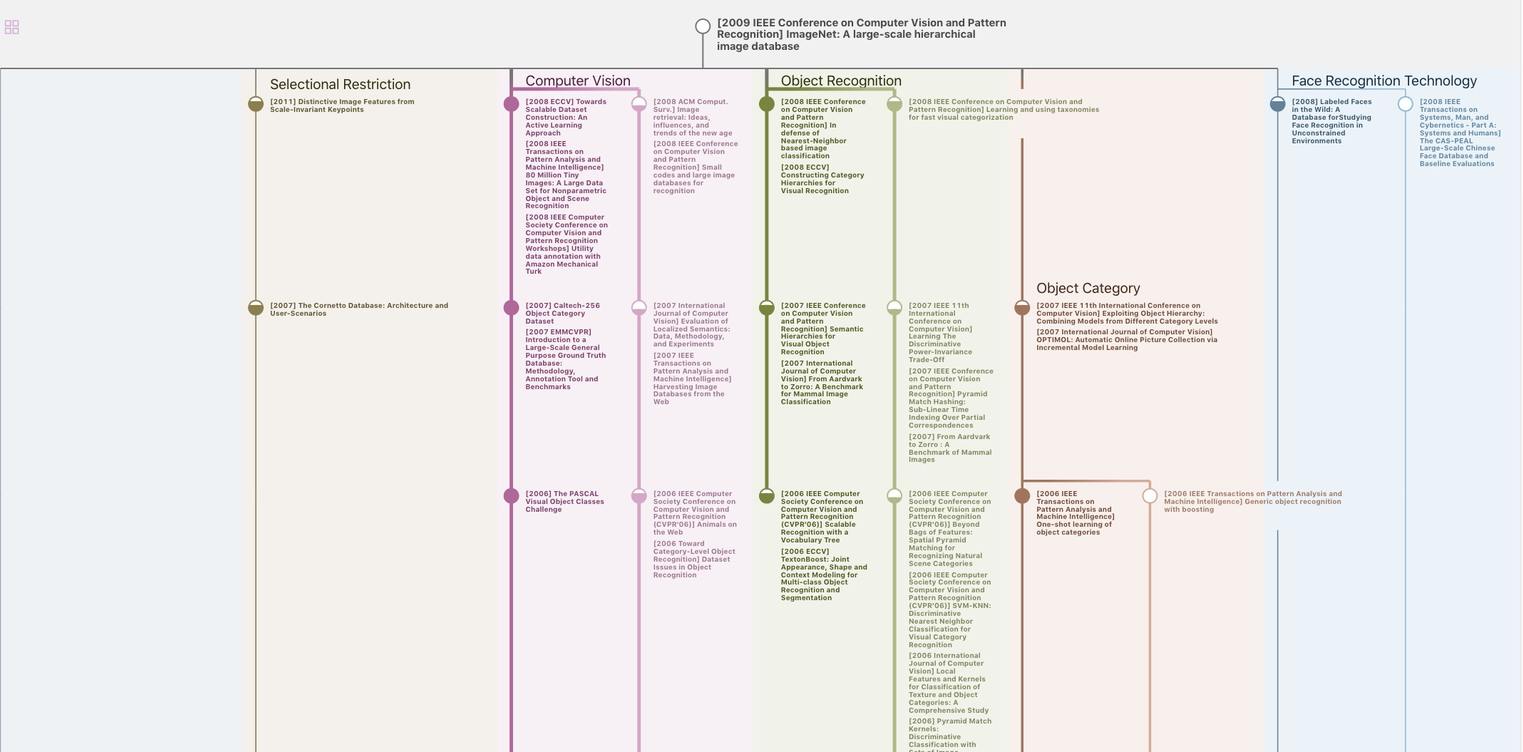
生成溯源树,研究论文发展脉络
Chat Paper
正在生成论文摘要