Emulator-based Bayesian optimization for efficient multi-objective calibration of an individual-based model of malaria
NATURE COMMUNICATIONS(2021)
摘要
Individual-based models have become important tools in the global battle against infectious diseases, yet model complexity can make calibration to biological and epidemiological data challenging. We propose using a Bayesian optimization framework employing Gaussian process or machine learning emulator functions to calibrate a complex malaria transmission simulator. We demonstrate our approach by optimizing over a high-dimensional parameter space with respect to a portfolio of multiple fitting objectives built from datasets capturing the natural history of malaria transmission and disease progression. Our approach quickly outperforms previous calibrations, yielding an improved final goodness of fit. Per-objective parameter importance and sensitivity diagnostics provided by our approach offer epidemiological insights and enhance trust in predictions through greater interpretability. Individual-based models have become important tools in the global battle against infectious diseases, yet model complexity can make calibration challenging. Here, the authors propose a Bayesian optimization framework to calibrate a complex malaria transmission simulator.
更多查看译文
AI 理解论文
溯源树
样例
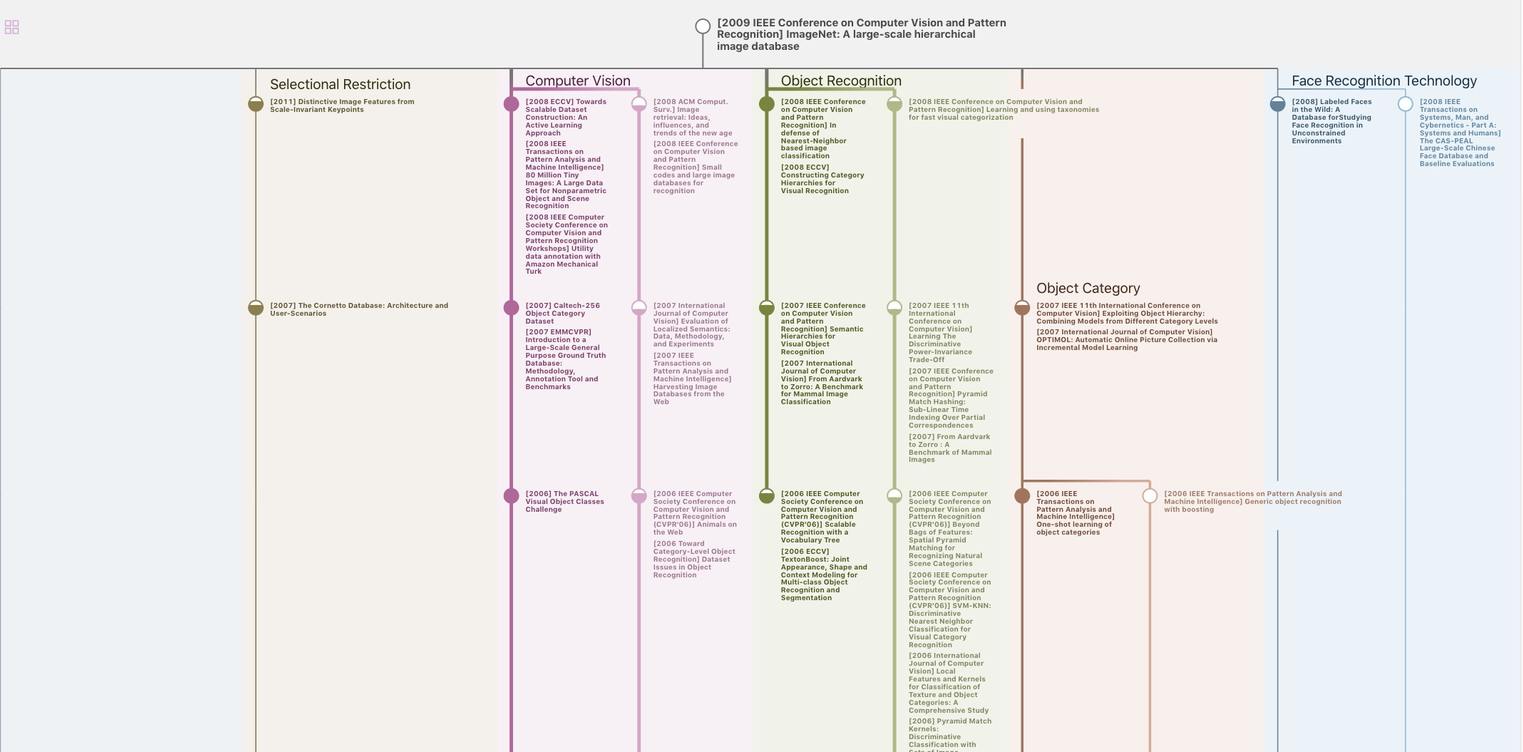
生成溯源树,研究论文发展脉络
Chat Paper
正在生成论文摘要