A vehicle classification system based on a non-intrusive sensor's binary image and convolutional neural networks
2021 14TH IEEE INTERNATIONAL CONFERENCE ON INDUSTRY APPLICATIONS (INDUSCON)(2021)
摘要
Express highways are increasingly adopting Intelligent Transportation Systems. Automatic vehicle classification is one of the most critical subsystems, as it is responsible for the validation of vehicle categories on electronic toll collection. Vehicle classification needs to reach a high success rate, and in real applications, it is achieved by using several intrusive sensors and sophisticated algorithms. This paper proposes a novel classification method based on binary images of vehicle profiles extracted from a non-intrusive optical barrier sensor. We used a modified AlexNet convolutional neural network as a vehicle classification algorithm. The last layers of the network were modified to the vehicle classification domain. We used transfer learning and data augmentation techniques. The method was tested using 11233 images grouped into a set of 11 categories. Results achieved 98.02% accuracy, which indicates that replacing a set of intrusive sensors by only an optical barrier may be a feasible vehicle classification alternative.
更多查看译文
关键词
intelligent transport systems,transfer learning,data augmentation,axle count,vehicle classification
AI 理解论文
溯源树
样例
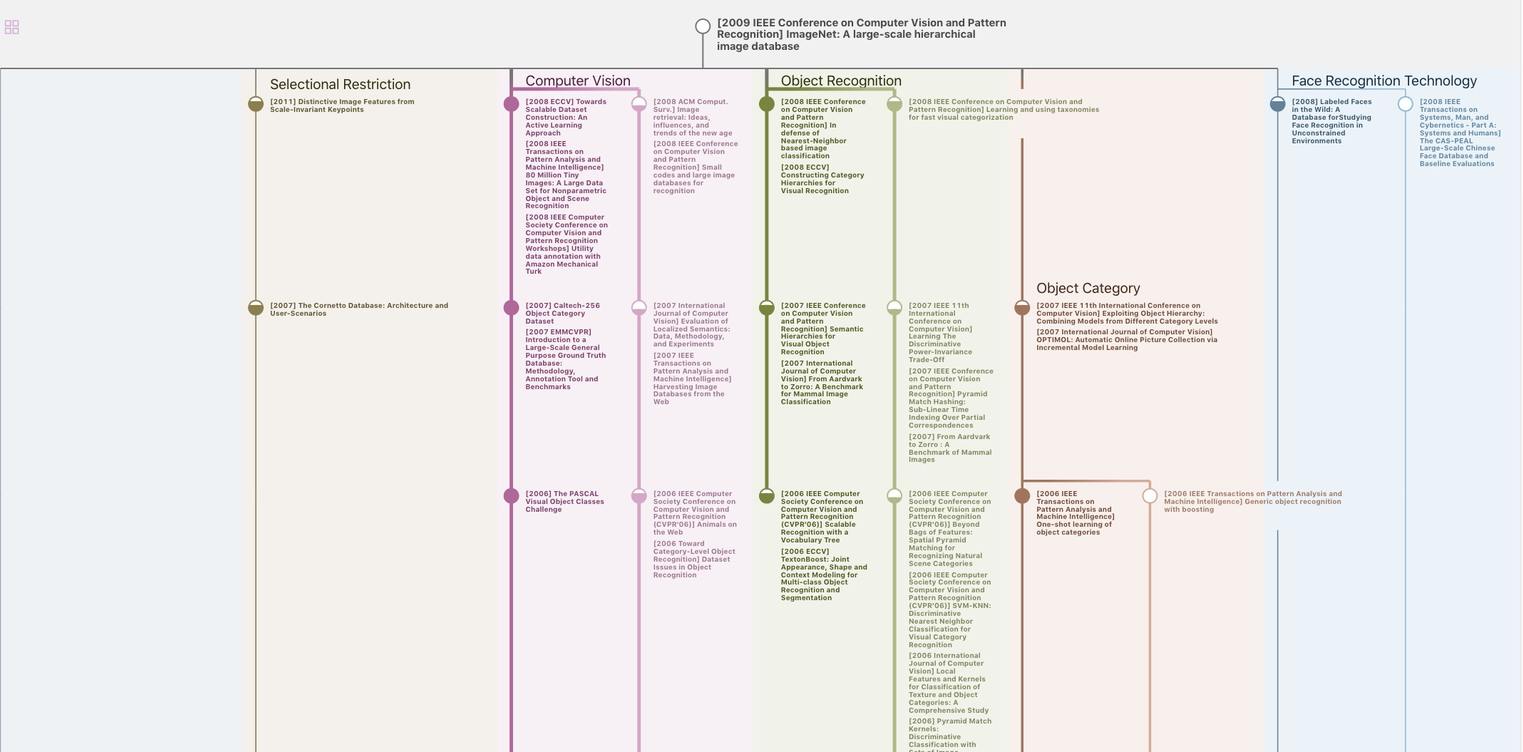
生成溯源树,研究论文发展脉络
Chat Paper
正在生成论文摘要