Automatic risk adjustment for profit maximization in renewable dominated short-term electricity markets
INTERNATIONAL TRANSACTIONS ON ELECTRICAL ENERGY SYSTEMS(2021)
摘要
State-of-the-art trading strategies in short-term electricity markets use risk awareness for reducing, inter alia, their exposure to the volatility of electricity prices. To ensure an optimal balance between risk and profit, risk-aversion parameters are traditionally fine-tuned via an offline out-of-sample analysis. Such a computationally-intensive analysis is typically run once, which yields time-invariant risk policies. Instead, this paper proposes the use of machine learning to select, in an online fashion, optimal risk-aversion parameters. This novel automatic risk-tuning approach offers the benefit of continuously adjusting the risk policy based on the dynamically changing market operating conditions. Our approach is tested on two risk-aversion parameters, that is, the confidence level of the conditional value-at-risk and the budget of uncertainty, respectively considering scenario-based and robust optimization frameworks. A set of performed case studies-focusing on the very short-term dispatch of a market actor participating in electricity markets-using real-world market data from the Belgian power system demonstrate the ability of the proposed methodology to outperform traditional offline risk policies.
更多查看译文
关键词
electricity markets, imbalance settlement, machine learning, risk management, stochastic optimization
AI 理解论文
溯源树
样例
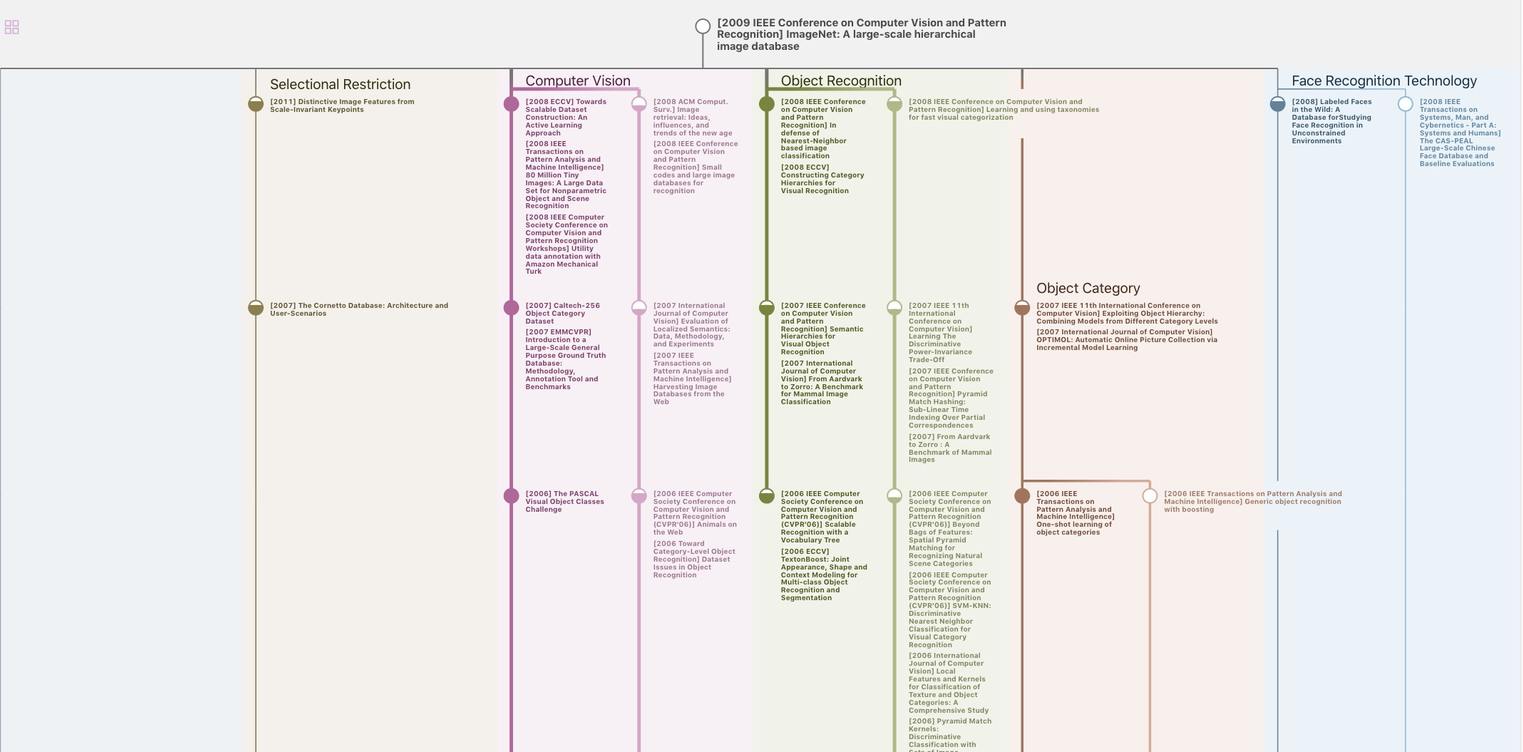
生成溯源树,研究论文发展脉络
Chat Paper
正在生成论文摘要