Recognition of penetration state in GTAW based on vision transformer using weld pool image
The International Journal of Advanced Manufacturing Technology(2022)
摘要
Automatic welding penetration recognition is a significant and challenging research direction to improve the manufacturing quality and intelligence level of gas tungsten arc welding (GTAW). In this paper, a data-driven deep learning approach based on vision transformer (ViT) is proposed to implement welding penetration recognition. To meet the requirement of the model training on data, a large weld pool image dataset composed of four penetration categories is constructed. Twelve ViT models with different architectures are constructed and trained from scratch to explore their feasibility for penetration recognition. Then, pretrained on ImageNet, a state-of-art ViT-B/16 model, is employed, which alleviates the issue of modeling complexity and data insufficiency and improves the validation accuracy by 4.45%. The transfer learning results demonstrate a 98.11% as the test accuracy for the ViT model. Compared with the other conventional recognition methods, the proposed model has fewer misclassifications and superior generalization performance due to self-attention. Furthermore, the attention rollout method is applied to visualize the image region of interest (ROI), confirming the reliability and effectiveness of the developed approach. This study expands the application scope of the ViT to penetration recognition and achieves remarkable effects, laying a reliable foundation for automated welding quality optimization in GTAW.
更多查看译文
关键词
Gas tungsten arc welding,Penetration recognition,Deep learning,Vision transformer,Self-attention
AI 理解论文
溯源树
样例
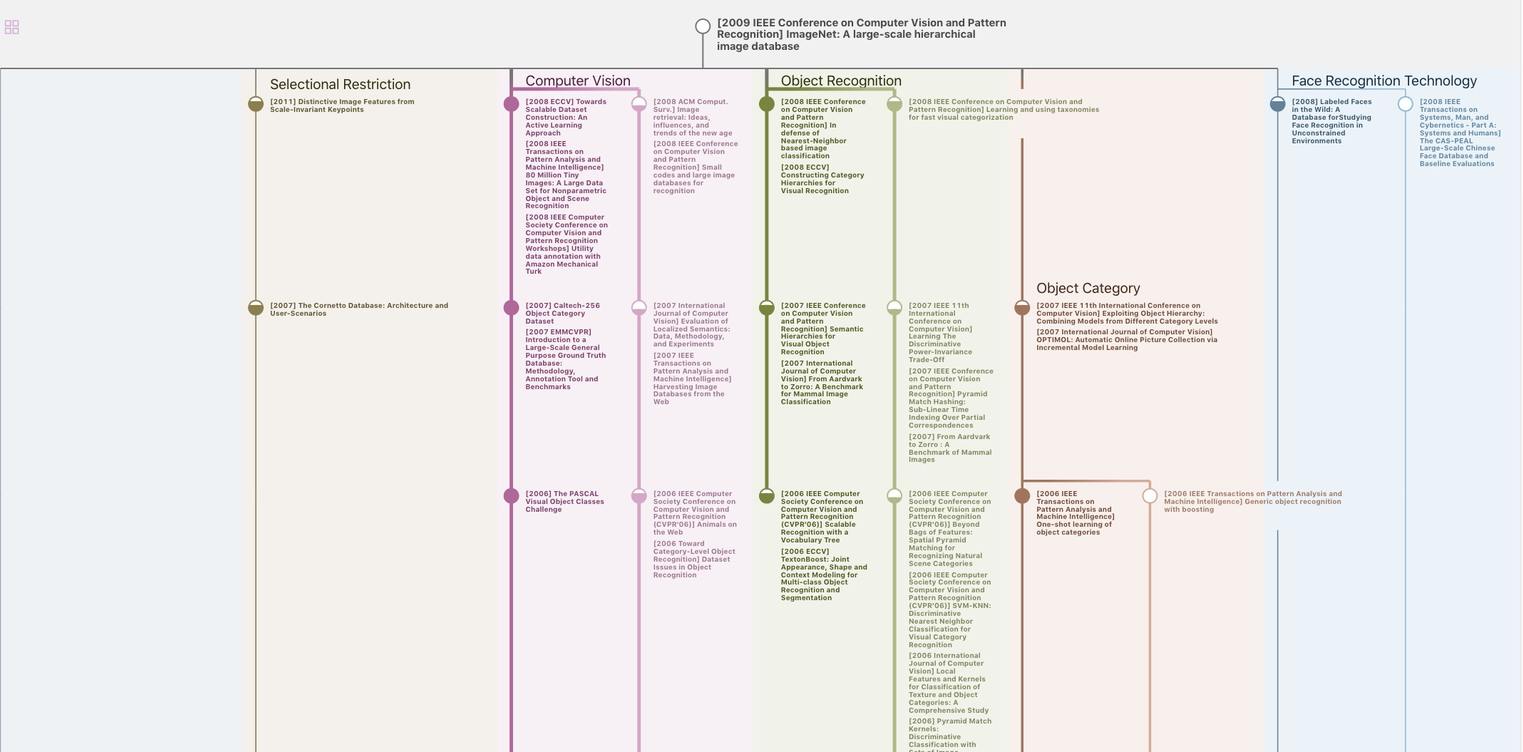
生成溯源树,研究论文发展脉络
Chat Paper
正在生成论文摘要