Fault diagnosis of rotating machinery based on dual convolutional-capsule network (DC-CN)
Measurement(2022)
Abstract
Health services for rotating machinery are essential to ensure safe industrial production. In recent years, deep learning (DL) methods based on vibration analysis have been continuously developed in rotating mechanical fault diagnosis (MFD). However, current diagnostic models based on DL facing three major challenges: (1) Convolutional neural network (CNN)-based DL algorithms lose spatial information of features, which may inevitably cause recognition errors; (2) Sufficient faulty training samples must be required to guarantee the learning capability of DL models, which may not be satisfied in actual engineering; (3) The poor generality of existing DL-based models make it difficult for different rotating machines or even different components of a mechanical device for diagnostic tasks. Toward the above problems, a revised DL model, namely dual convolution-capsule network (DC-CN), is introduced to diagnose rotating machinery faults under minor sample conditions. The DC-CN well merits the advantages of CNN and the capsule network (CN), which fully retains spatial information while digging in-depth fault features. Therefore, DC-CN achieves an ideal balance between diagnostic effectiveness and data amount. Experiments including different rotating machinery and different fault components are conducted to validate the diagnostic performance of the proposed model, and the t-SNE dimensionality reduction algorithm is introduced to visualize the extracted features. The proposed DC-CN demonstrated the best diagnostic capability than other DL models in different rotating machinery: 1. For rolling bearing, DC-CN achieved 99.78%, 98.22%, 100%, and 100% under 4 different loading conditions; 2. For the planetary gearbox, DC-CN reached 96.91% and 98.22% under different rotational conditions, including 8 health scenarios of different gear types; 3. DC-CN has excellent performance under small sample conditions.
MoreTranslated text
Key words
Rotating machinery,Deep learning,DC-CN,Intelligent fault diagnosis,Rolling bearings,Planetary gearboxes
AI Read Science
Must-Reading Tree
Example
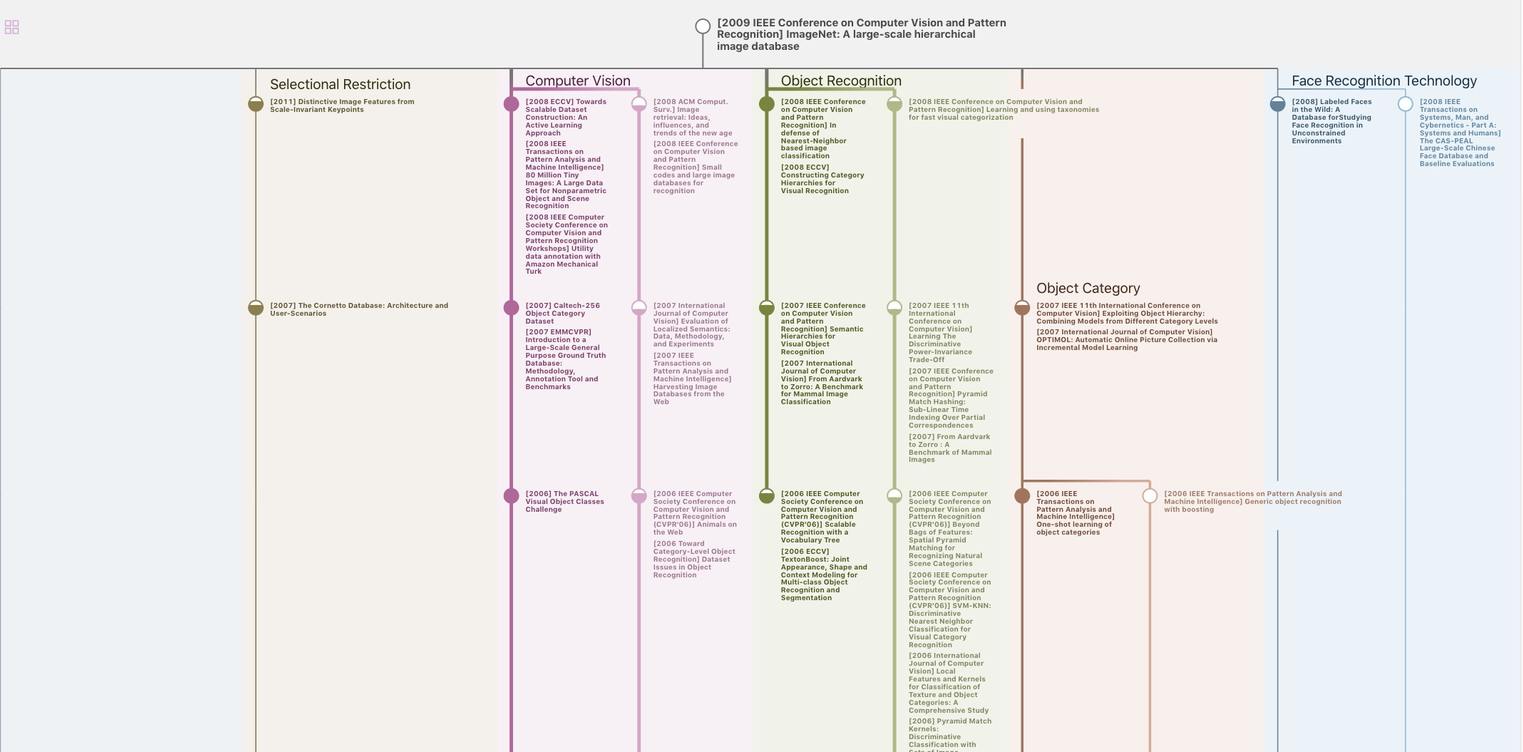
Generate MRT to find the research sequence of this paper
Chat Paper
Summary is being generated by the instructions you defined